Semi-Supervised Fault Diagnosis Method Based on Dual-Stream Attention Cyclic Auto-Encoder for Rotating Components With Low Label Rate
IEEE SENSORS JOURNAL(2024)
摘要
Recently, deep learning-based fault diagnosis methods have shown promising outcomes in detecting faults within rotating components, relying on substantial labeled training data. However, the scarcity of labeled data is a prevalent challenge in practical engineering and results in unsatisfactory diagnostic results. To address this issue, a semi-supervised fault diagnosis method named dual-stream attention cyclic auto-encoder (DA-CAE) is proposed in this article. First, a CAE is designed to capture and utilize high-dimensional feature information through a cyclic structure. Subsequently, a DA module is incorporated to effectively extract crucial features from a global perspective, thereby enhancing the feature extraction capabilities of the CAE. Finally, a classifier sharing the pretrained CAE is developed and a two-stage training methodology is employed to facilitate fault recognition confronting limited labeled data. The feasibility of the proposed method is validated through experimental data from two rotating components. The results demonstrate that DA-CAE can significantly improve the performance of the auto-encoder (AE) with various label rates and has better superiority compared to the comparison methods, particularly in low-label rate scenarios.
更多查看译文
关键词
Feature extraction,Fault diagnosis,Decoding,Training,Data models,Sensors,Task analysis,Cyclic auto-encoder (CAE),dual-stream attention (DA),fault diagnosis,low label rate,rotating component
AI 理解论文
溯源树
样例
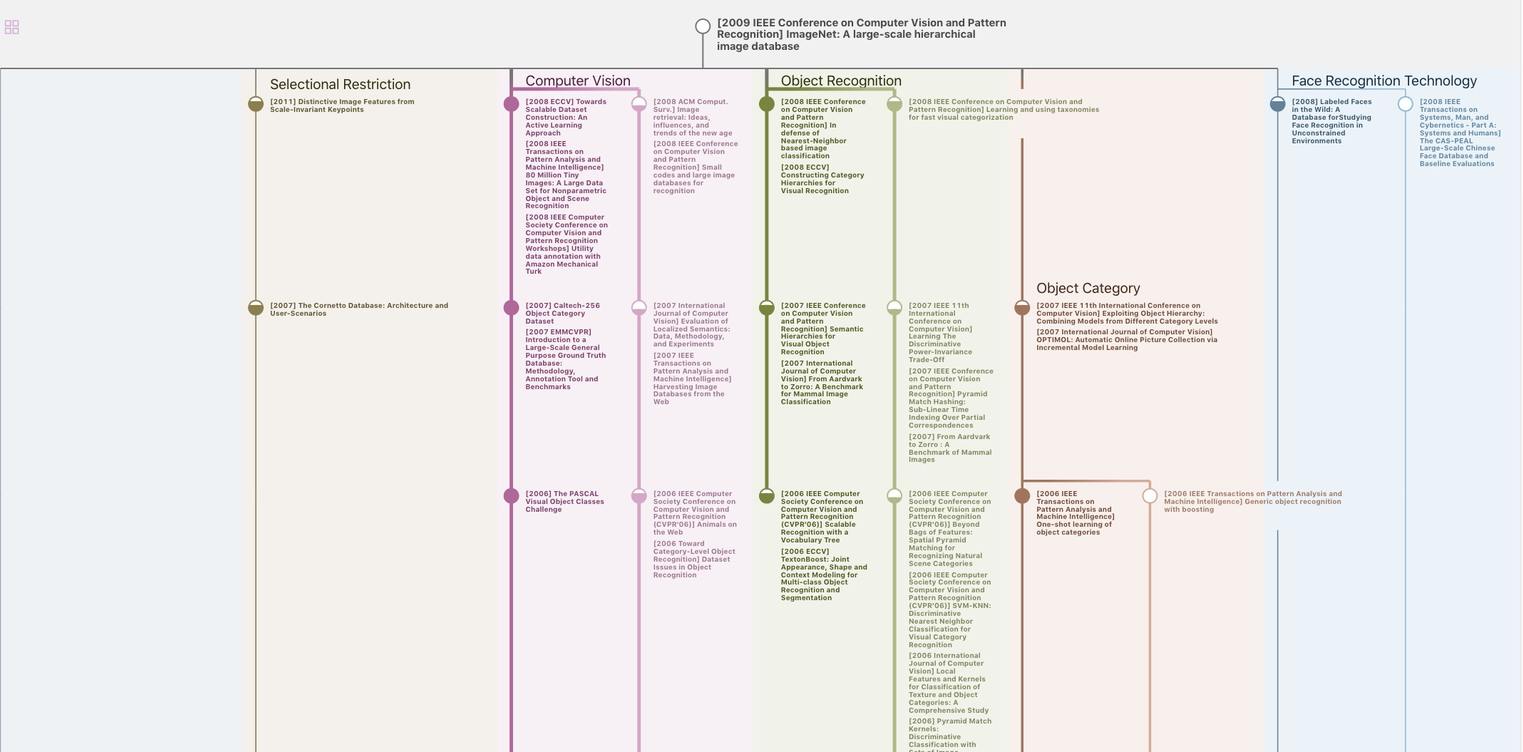
生成溯源树,研究论文发展脉络
Chat Paper
正在生成论文摘要