ADERLNet: Adaptive Denoising Enhancement Representation Learning for Low-Latency and High-Accurate Target Detection on SAR Sensors
IEEE Sensors Journal(2024)
摘要
Target detection on synthetic aperture radar (SAR) imagery is critical for several military and civilian applications. However, the coherent imaging mechanism of SAR will inevitably generate speckle noise, reducing the signal-to-noise ratio of SAR imagery, resulting in the loss of certain important information related to texture and thus degrading the accuracy of SAR image target detection. In addition, learning irrelevant features, such as natural clutter, increases the false alarm rate. To address these issues, this paper proposes a high-accurate and low-latency SAR target detection network (ADERLNet) to enhance the ability of the target detection model to represent valid features from strongly noisy images and irrelevant features. Specifically, the deep target detection architecture incorporates soft thresholding functions as nonlinear transformation layers, which can eliminate noisy and irrelevant features by forcing these interference features to be zeros. Second, given that manually setting the appropriate thresholds in the soft thresholding functions is challenging, the thresholds in ADERLNet are automatically determined to avoid substantial signal processing expertise by integrating specially designed sub-networks as trainable modules. Finally, the classification and regression losses are redesigned to offer the model stronger generalization ability by balancing the positive and negative samples and handling the impact of possible low-quality samples in the training set. Additionally, the structural re-parameterization method is employed to balance accuracy and speed. Experimental results on three widely used datasets for SAR target detection, including SSDD, MSAR-1.0, and SAR-Ship-Dataset, reveal that ADERLNet maximally improves average precision (AP) by 2.7%, 3.3%, and 1.8%, respectively, and far outperforms other methods on detection accuracy while maintaining excellent real-time performance, fully highlighting the superiority of ADERLNet. The trained models and source codes will be available at https://github.com/yangyahu-1994/ADERLNet.
更多查看译文
关键词
Attention mechanism,target detection,soft thresholding,deep learning,synthetic aperture radar (SAR)
AI 理解论文
溯源树
样例
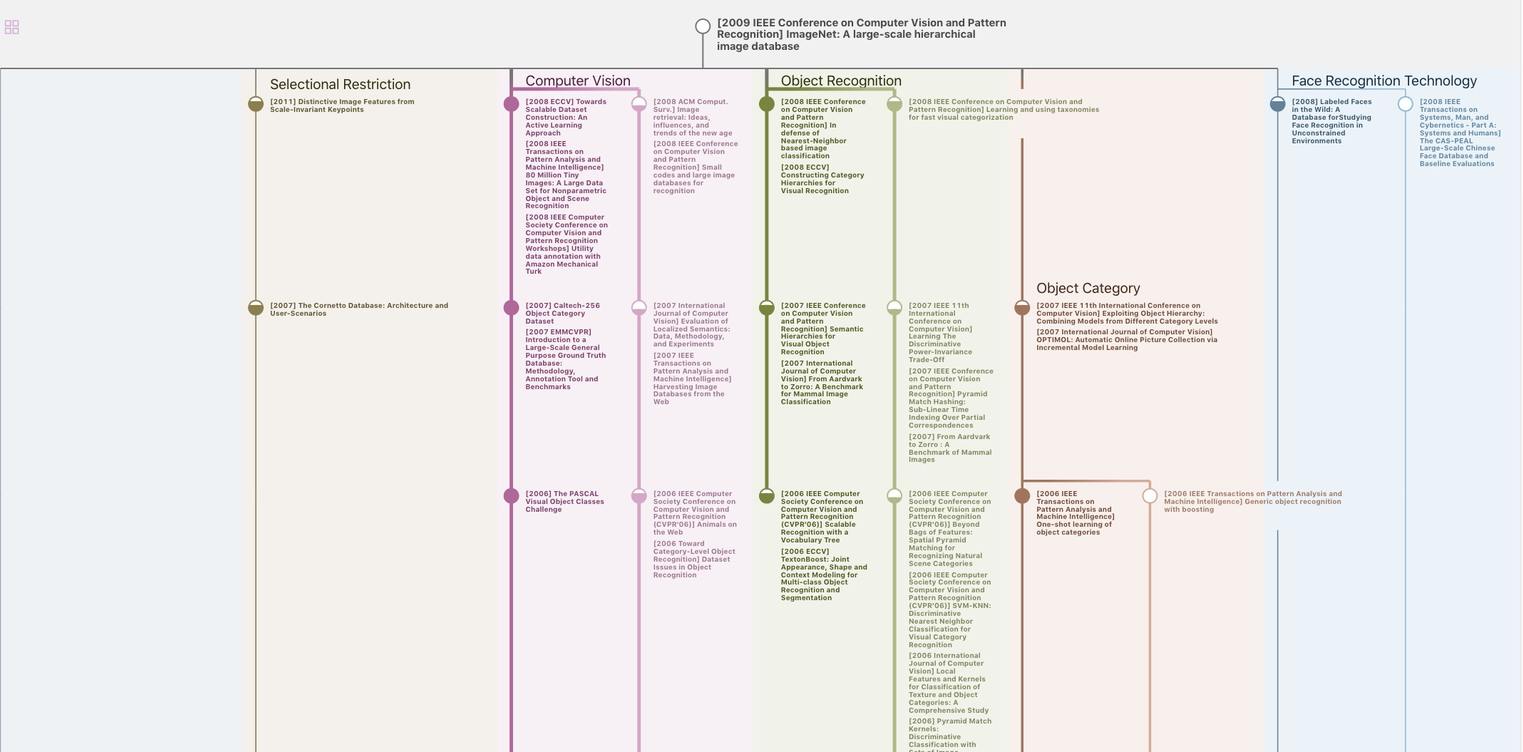
生成溯源树,研究论文发展脉络
Chat Paper
正在生成论文摘要