A Correlation-augmented Informer-based Method for State-of-Health Estimation of Li-ion Batteries
IEEE Sensors Journal(2023)
摘要
Accurate estimation of the State of Health (SOH) of lithium-ion batteries is crucial to ensure their safe and reliable use. To address the issue of neglecting correlations between different charge/discharge cycles in current neural network-based methods, this paper introduces a correlation-augmented informer network. Specifically, the multiple correlation coefficient is used to analyze the correlation between different cycles, and then a correlation-augmented informer containing embedding, encoder, and decoder is constructed. To emphasize cycles with stronger correlations during the estimation process, we incorporate Multi-Head Local Self-Attention mechanisms. We validate our approach on two publicly available datasets using Mean Absolute Error (MAE), Mean Absolute Percentage Error (MAPE), and Root Mean Square Error (RMSE), which are 0.2%, 0.3%, and 0.2% on the MIT dataset and 1.0%, 1.4%, and 1.8% on the CALCE dataset, respectively. These results demonstrate the superior accuracy and robustness of the proposed method in comparison to existing state-of-the-art neural network methods.
更多查看译文
关键词
lithium-ion battery,State-of-Health,multiple correlation coefficient,Informer
AI 理解论文
溯源树
样例
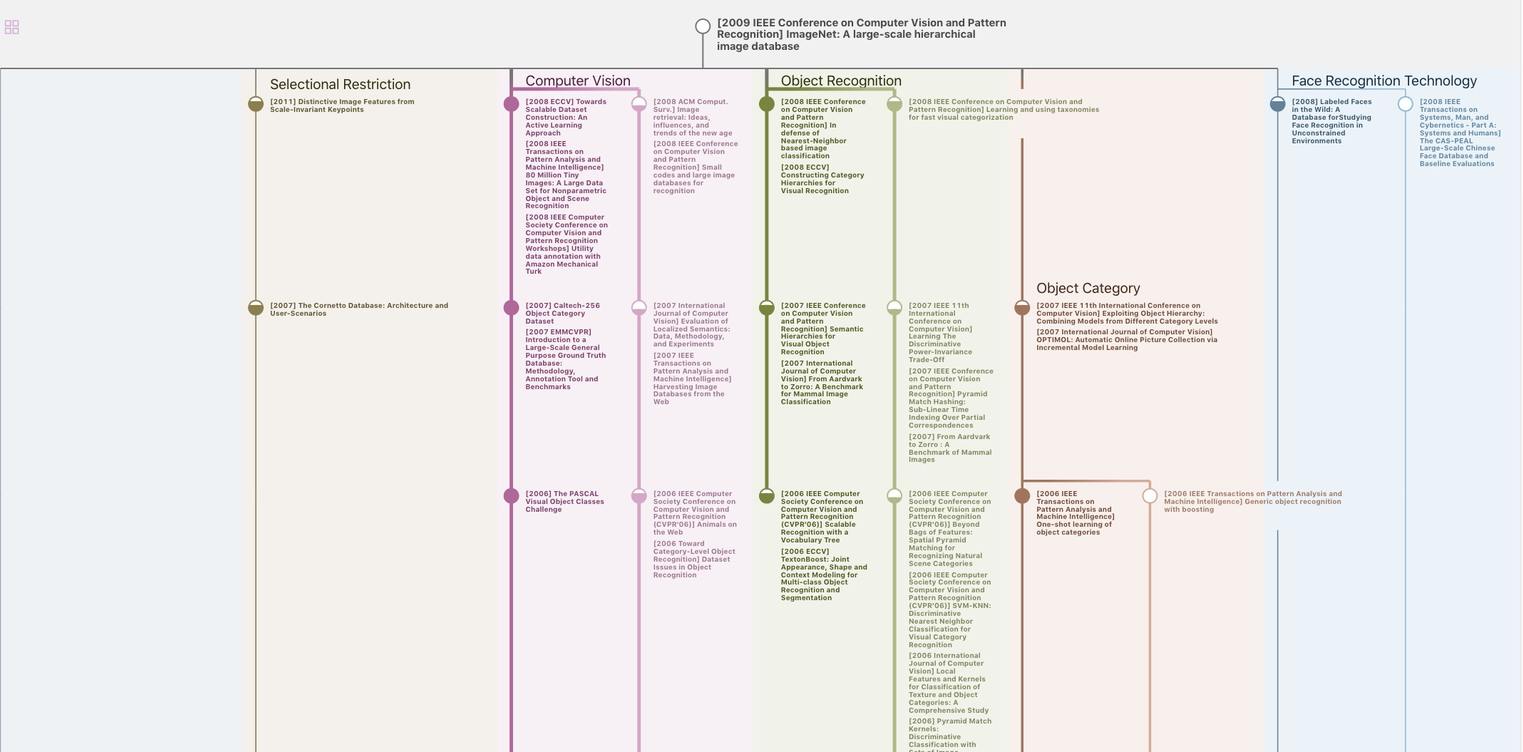
生成溯源树,研究论文发展脉络
Chat Paper
正在生成论文摘要