Over-the-Air Clustered Federated Learning
IEEE Transactions on Wireless Communications(2023)
摘要
Over-the-air federated learning (FL) is a recent paradigm to address the communication bottleneck of FL, where a machine learning model is trained by aggregating the local gradients directly in the wireless medium. On the other hand, due to the inherent data heterogeneity across wireless users, training a single model to serve all users can severely degrade individual user performance. Towards addressing this challenge, in this work we propose
over-the-air clustered FL
, where multiple models are trained concurrently over-the-air, and each model is adapted gradually to a group of users with similar data distributions. We introduce AirCluster, an over-the-air clustered FL framework with coordinated zero-forcing MIMO beamforming, along with a sketching-based dimensionality reduction mechanism to enable over-the-air training with limited number of antennas. Our theoretical analysis provides formal convergence guarantees for the trained models, while identifying the key performance trade-offs in terms of the convergence rate, compression ratio, channel quality, and the number of antennas. Through extensive experiments on multiple datasets, we observe significant increase in the test accuracy for individual users over state-of-the-art FL benchmarks. Our results demonstrate over-the-air FL to be a promising approach in addressing the communication bottleneck of FL, even under severe data heterogeneity.
更多查看译文
关键词
Over-the-air machine learning,clustered federated learning,distributed training
AI 理解论文
溯源树
样例
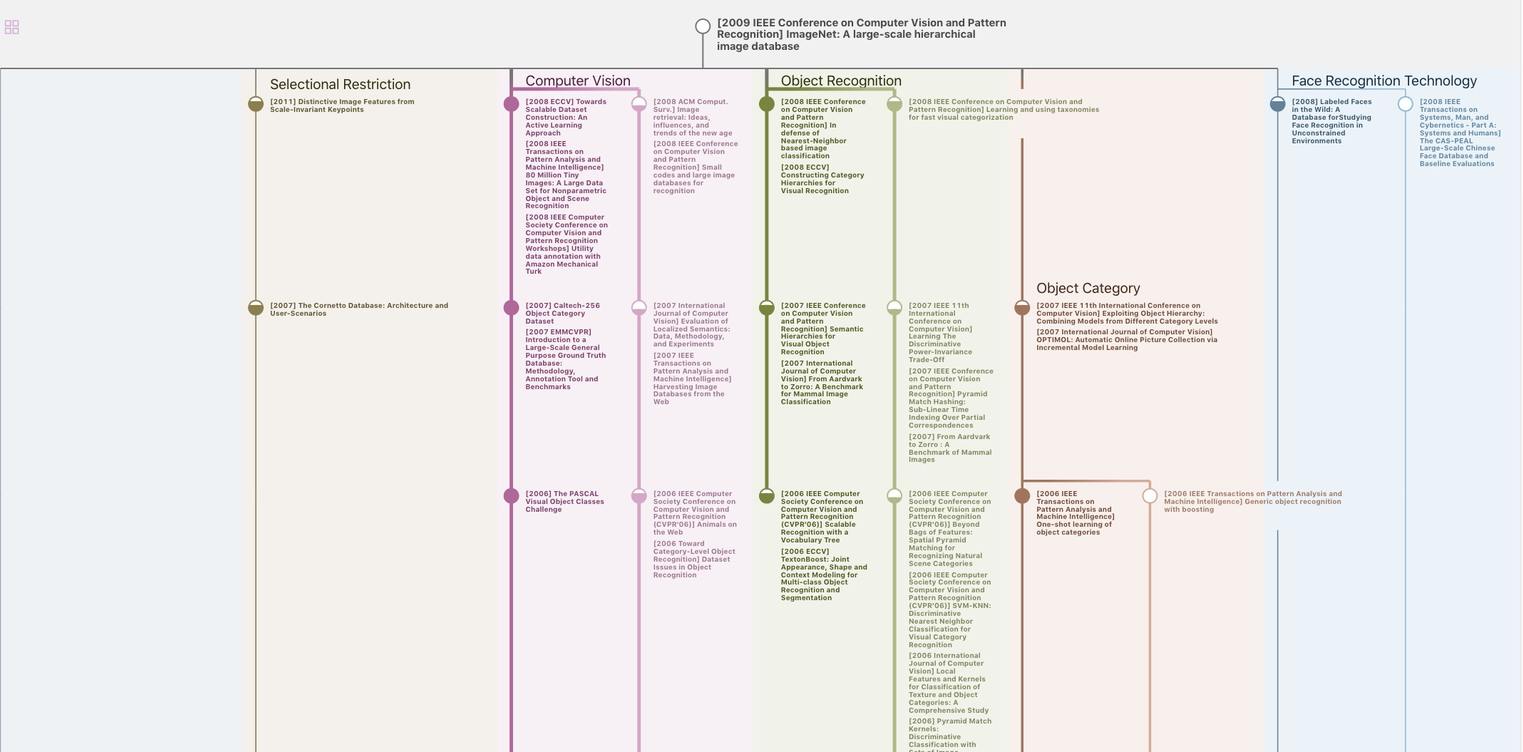
生成溯源树,研究论文发展脉络
Chat Paper
正在生成论文摘要