CFENet: Boosting Few-Shot Semantic Segmentation With Complementary Feature-Enhanced Network
IEEE TRANSACTIONS ON MULTIMEDIA(2024)
摘要
Few-shot semantic segmentation aims to extract information from few annotated support images to segment unknown class objects in the query image. Traditional algorithms may produce errors and insufficient feature extraction using multi-layer cosine similarity to extract correlation information, due to the large differences in appearance and posture between novel class objects, as well as the similarity in texture and shape among different categories. To address the above issue, we propose a Complementary Feature-Enhanced Network (CFENet). Specifically, we propose a correlation complementary extraction module (CCEM) to facilitate long-range information interaction between query features and support features in the intermediate layer, which contains detailed information. The generated multi-channel correlation information complements the prior information obtained through cosine similarity comparison. In addition, we propose a multi-branch feature enhancement module to capture long-range dependencies in aggregated features which are composed of prior correlation information and query features. The module effectively suppresses noise in the aggregated features and enhances the query target object feature from both global and local perspectives in a complementary way. Experiments of the network on PASCAL-5(i )and COCO-20(i) datasets validate the effectiveness of our proposed method.
更多查看译文
关键词
Few-shot learning,semantic segmentation,few-shot semantic segmentation
AI 理解论文
溯源树
样例
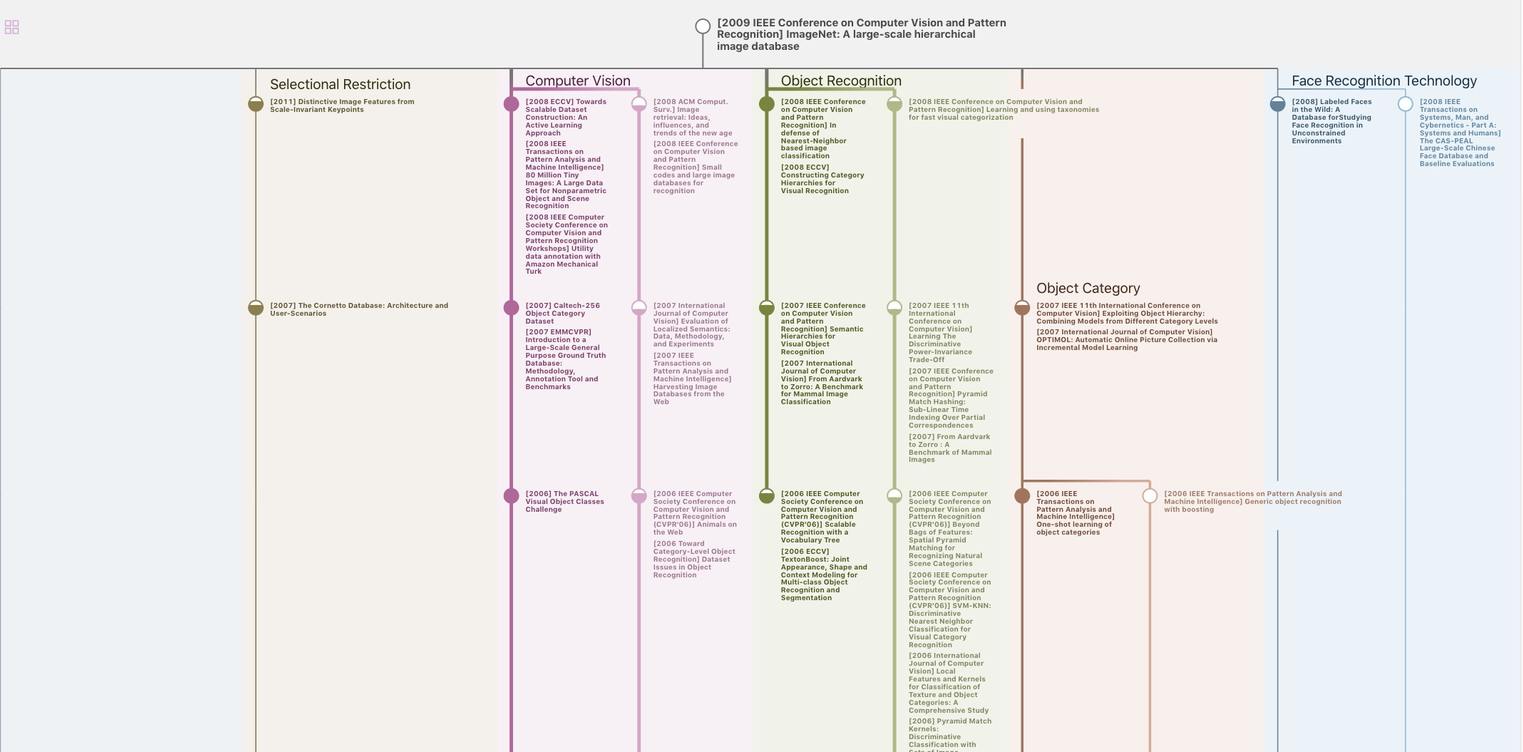
生成溯源树,研究论文发展脉络
Chat Paper
正在生成论文摘要