A Multiscale Coarse-to-Fine Human Pose Estimation Network with Hard Keypoint Mining
IEEE Transactions on Systems Man and Cybernetics Systems(2023)
关键词
Pose estimation,Standards,Convolution,Training,Task analysis,Heating systems,Detectors,Hard sample mining,human pose estimation,multiscale
AI 理解论文
溯源树
样例
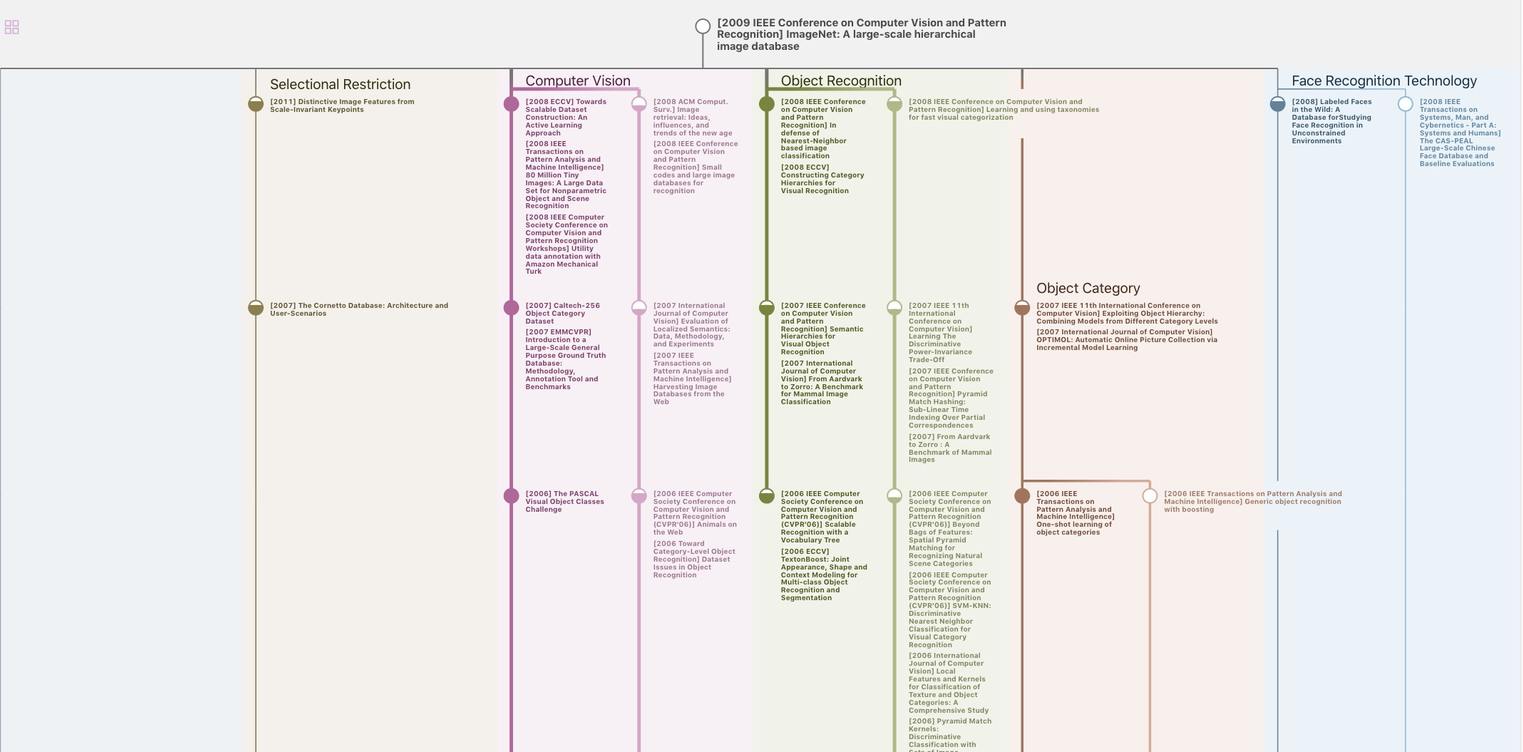
生成溯源树,研究论文发展脉络
Chat Paper
正在生成论文摘要