Computation Offloading and Trajectory Planning of Multi-UAV-Enabled MEC: A Knowledge-Assisted Multiagent Reinforcement Learning Approach.
IEEE Trans. Veh. Technol.(2024)
摘要
Compared with centralized cloud computing, mobile edge computing (MEC) enables Internet of Things (IoT) devices to offload computation-intensive tasks to the edge of the network closer to them for processing, which can effectively save energy consumption of IoT devices and alleviate network congestion and high latency problems. However, the traditional terrestrial MEC system cannot adapt to scenarios such as rapid network recovery after disasters and emergency rescue due to its poor flexibility and high deployment cost, and assembling edge servers to unmanned aerial vehicles (UAVs) to assist in rapidly building mobile edge networks is a feasible solution. Therefore, this paper considers a multi-UAV-enabled MEC network with the optimization objectives of maximizing the processing success rate of computational tasks and fairness of system, while minimizing the processing delay of computational tasks. We investigate the computation offloading problem and the trajectory planning problem from the perspectives of IoT devices and UAVs, respectively. Then model them as Markov decision processes (MDPs) and propose a joint optimization scheme based on expert knowledge-assisted multi-agent reinforcement learning algorithms. Simulation results show that the proposed algorithm has significant advantages over baseline algorithms in terms of processing success rate and delay of computational tasks as well as fairness of the system.
更多查看译文
关键词
Mobile edge computing (MEC),unmanned aerial vehicle(UAV),multi-agent reinforcement learning (MARL),computation offloading,trajectory planning
AI 理解论文
溯源树
样例
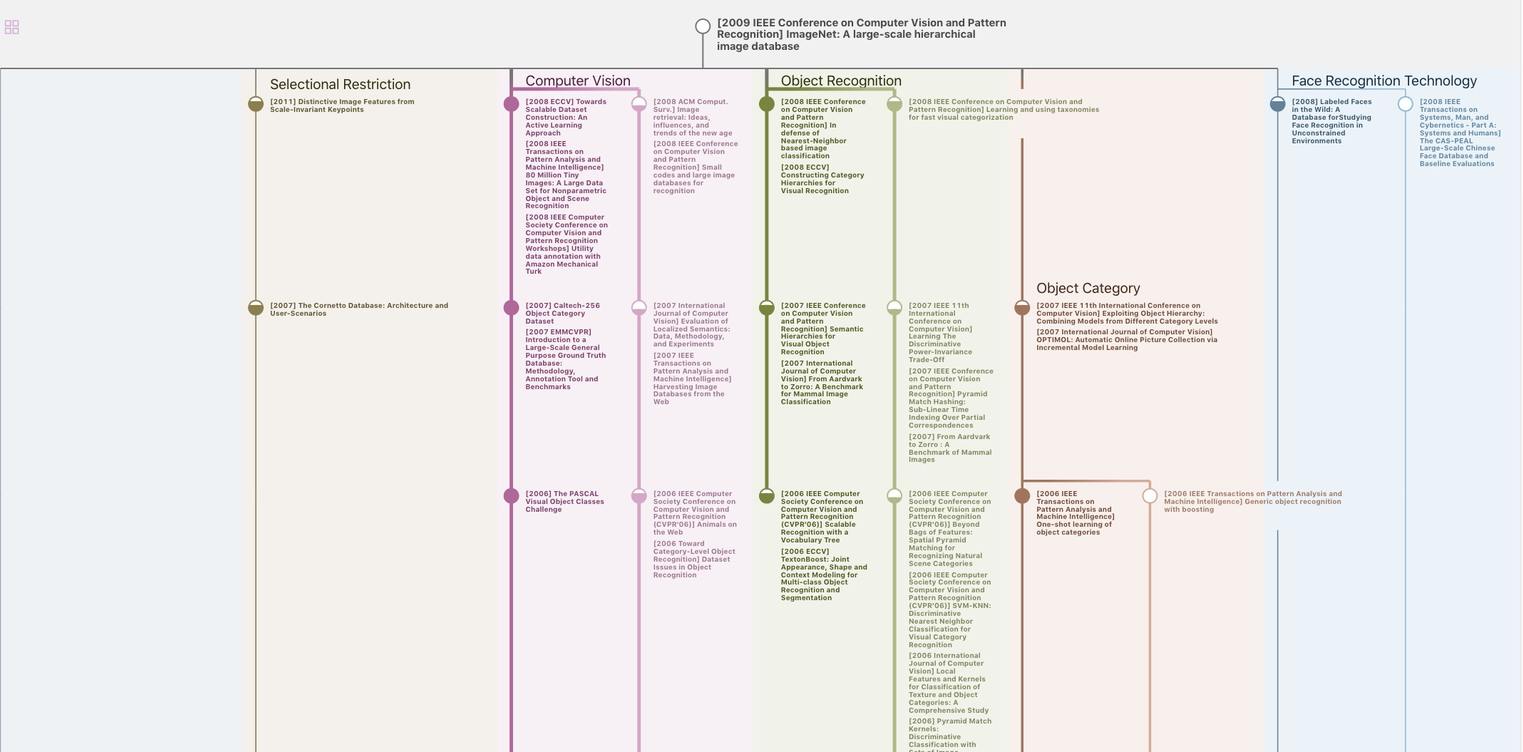
生成溯源树,研究论文发展脉络
Chat Paper
正在生成论文摘要