RDNRnet: A Reconstruction Solution of NDVI Based on SAR and Optical Images by Residual-in-Residual Dense Blocks
IEEE TRANSACTIONS ON GEOSCIENCE AND REMOTE SENSING(2024)
摘要
The reconstruction of the normalized difference vegetation index (NDVI) is a crucial prerequisite for numerous spatiotemporal continuous studies. To address the limitations posed by satellite temporal resolution and challenging atmospheric conditions, the combination of synthetic aperture radar (SAR) and optical images from diverse sources has proven to be effective and widely employed. In this study, we employ the spatial-temporal Savitzky-Golay (STSG) algorithm to rectify MODIS NDVI maps and eliminate interruptions caused by noise. Random forest (RF) and gradient boosting decision trees (GBDTs) serve as a dual filter to select SAR indices with the highest impact on NDVI reconstruction, ensuring that the chosen indices encapsulate the most valuable information. Subsequently, we conducted a series of ablation experiments and developed a deep learning network named residual-in-residual dense block (RRDB) NDVI reconstruction net (RDNRnet). This network effectively mitigates the impacts of MODIS coarse resolution and speckle noises in SAR data. We also evaluate the network performance in reconstructing NDVI across all seasons and land cover types. Our findings highlight that the modified dual-polarimetric SAR vegetation index and the standard deviation (STD) of the vertical-vertical (VV) band are the most crucial SAR indices. The predictions for summer exhibit the highest performance, with a coefficient of determination ( R-2 ) reaching 0.9757. Optimal performances by land cover type are observed in forests, paddy fields, and dry farming fields, all with R-2 values exceeding 0.9580. Our adaptive NDVI reconstruction solution demonstrates robust performance across different data availability scenarios, effectively catering to all seasons and land cover types.
更多查看译文
关键词
Image reconstruction,normalized difference vegetation index (NDVI),residual-in-residual dense block NDVI reconstruction net (RDNRnet),synthetic aperture radar (SAR)
AI 理解论文
溯源树
样例
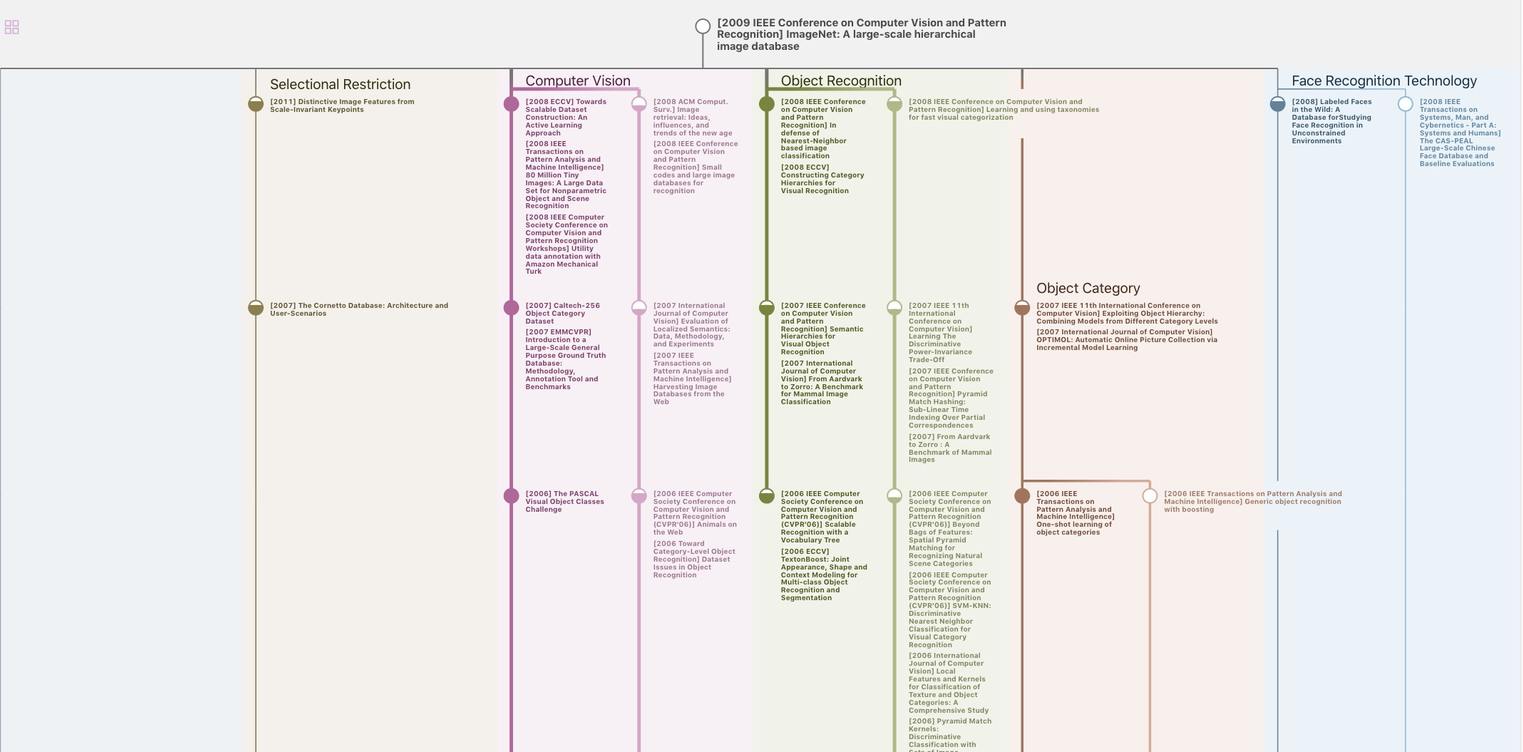
生成溯源树,研究论文发展脉络
Chat Paper
正在生成论文摘要