A Spatiotemporal Multiscale Deep Learning Model for Subseasonal Prediction of Arctic Sea Ice.
IEEE Transactions on Geoscience and Remote Sensing(2024)
摘要
Physics-based dynamical models can successfully predict short-term sea ice trends, but they struggle to provide reliable subseasonal sea ice predictions over shorter time periods and are not flexible enough to provide real-time/quasi-real-time predictions. Therefore, we use deep learning to establish a purely remote sensing data-driven Arctic sea ice prediction system, IceFormer. IceFormer is trained by high-quality Arctic reanalysis data and remote sensing observations. It can provide daily sea ice concentration (SIC) and sea ice thickness (SIT) predictions for the pan-Arctic region with 45 days lead time. We show that IceFormer advances lightweight multivariate forecasting of Arctic sea ice. Driven by remote sensing data, the prediction performance of IceFormer is far superior to traditional baseline models and machine learning models. The root mean square error (RMSE) and spatial anomaly correlation coefficient (SACC) of SIC (averaged within 45 days) are 6.62% and 0.80, respectively. The RMSE and SACC of SIT (averaged within 45 days) are 0.04m and 0.93, respectively. IceFormer can successfully capture the sea ice evolution on a seasonal scale (especially in the melting season) and the monthly average SACC is stable at around 0.70. After comparison, the SIC prediction performance of IceFormer is better than that of a Coupled Ensemble Numerical Forecast Model (SEAS5) from May to November. The information transfer analysis shows that the simultaneous prediction of SIC and SIT can effectively improve the predictability and explainability of sea ice.
更多查看译文
关键词
Deep learning,remote sensing data-driven,sea ice concentration (SIC),sea ice thickness (SIT),spatio-temporal prediction
AI 理解论文
溯源树
样例
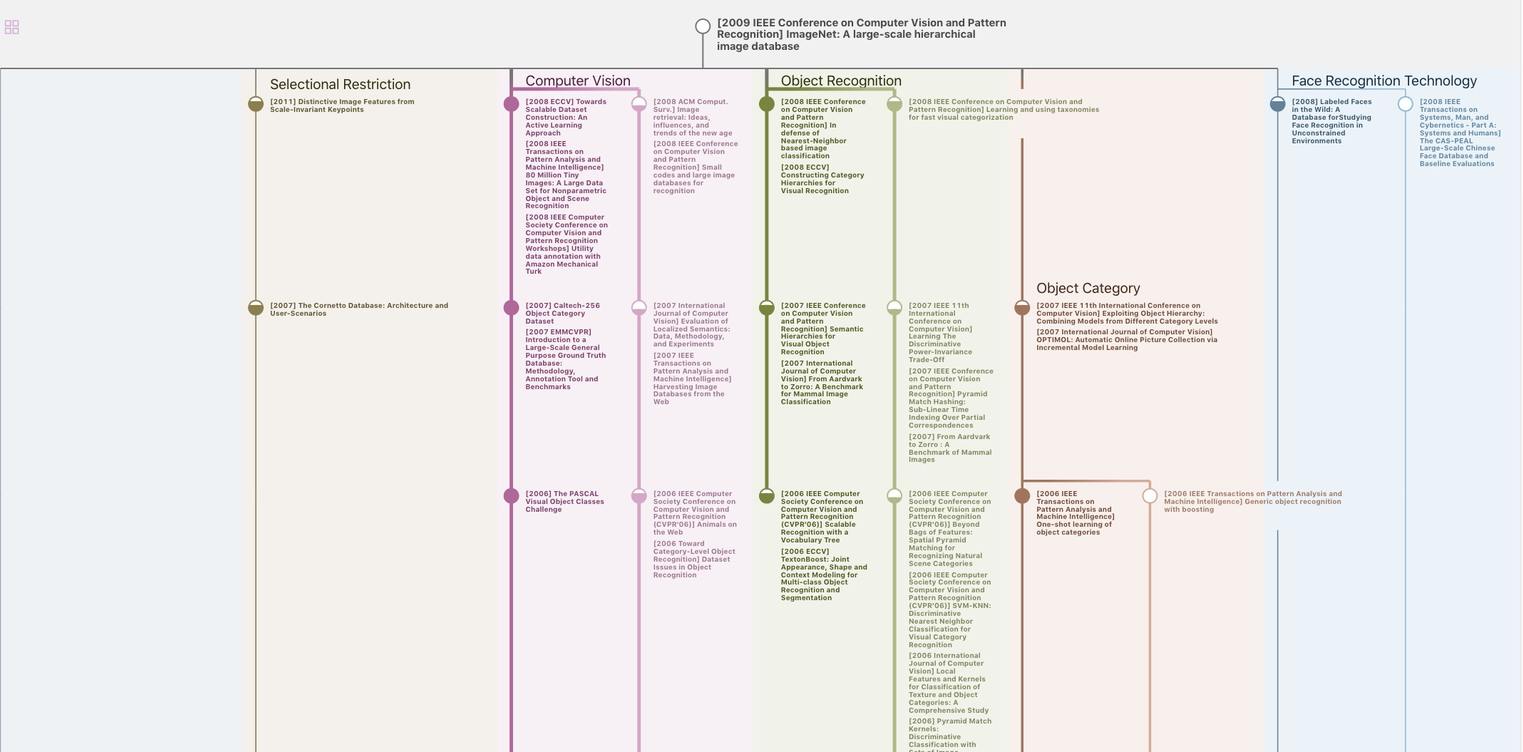
生成溯源树,研究论文发展脉络
Chat Paper
正在生成论文摘要