A Multiscale Incremental Learning Network for Remote Sensing Scene Classification
IEEE TRANSACTIONS ON GEOSCIENCE AND REMOTE SENSING(2024)
摘要
To infer unknown remote sensing scenarios, for remote sensing scene classification (RSSC), most existing deep neural networks (DNNs) are trained on closed datasets. When the acquisition speed and quantity of remote sensing images increase rapidly, these models cannot be used to classify new scenes. Currently, incremental learning as an effective solution for solving the catastrophic forgetting issue, but ignoring the stability-plasticity dilemma. In this article, we propose a new incremental learning network, named efficient channel attention-based multiscale depthwise network (ECA-MSDWNet), in which efficient channel attention (ECA) improves the model's ability to focus on critical information in complex context, and multiscale depthwise convolution (MSDW Conv) extracts multiscale features in a fine-grained way. In addition, in incremental learning process, we expand new modules based on a dynamic-structure method to fit the residuals between the labels and the outputs of the old model, enhancing the plasticity of the new model for new tasks while maintaining the performance of the old tasks. Finally, we compress the model to reduce redundant parameters and feature dimensions through an effective knowledge distillation strategy. Experiments on four open datasets demonstrate the effectiveness of our method. Our code is available at https://github.com/zhangyu-chd/ECA-MSDWNet.
更多查看译文
关键词
Task analysis,Remote sensing,Feature extraction,Data models,Knowledge engineering,Context modeling,Computational modeling,Catastrophic forgetting,incremental learning,multiscale feature,remote sensing scene classification (RSSC)
AI 理解论文
溯源树
样例
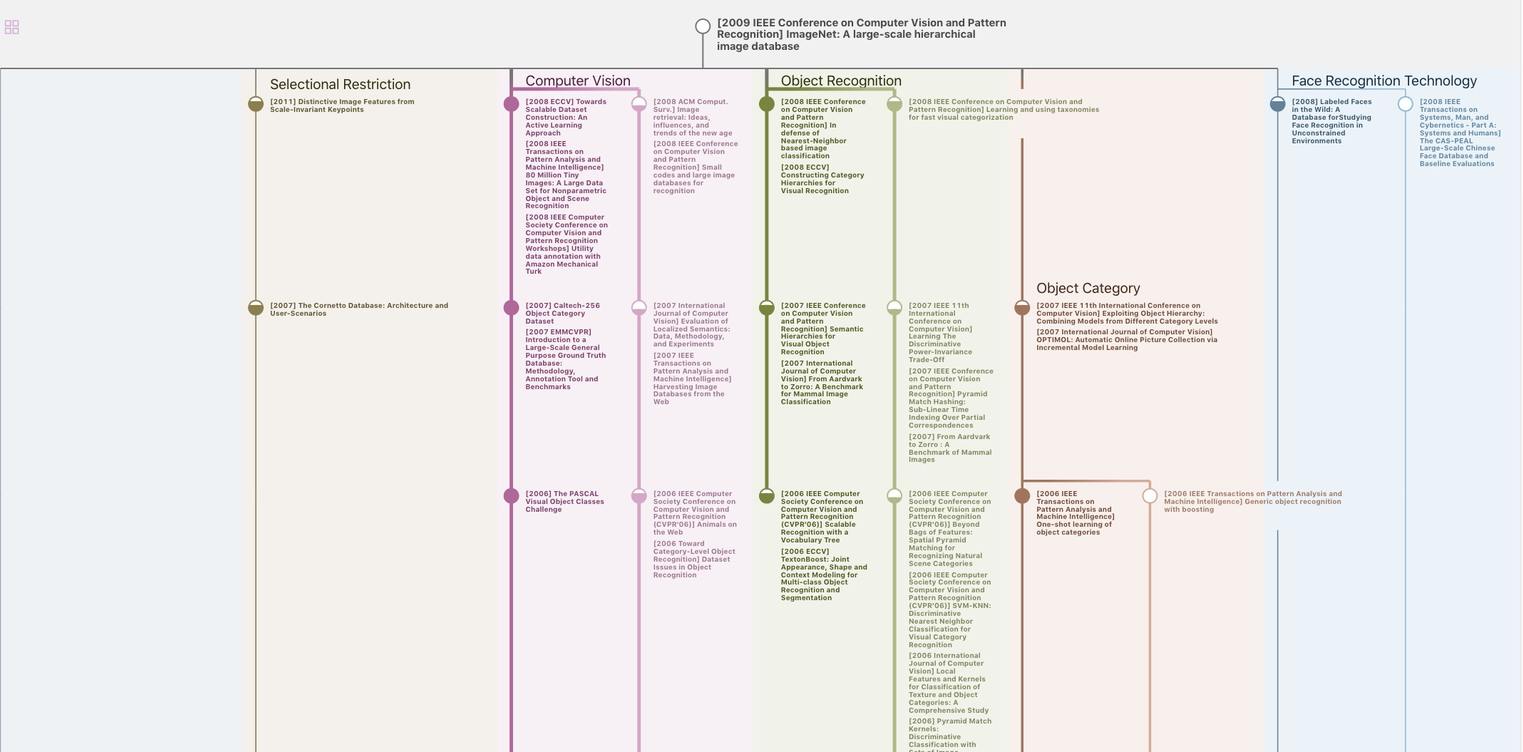
生成溯源树,研究论文发展脉络
Chat Paper
正在生成论文摘要