MDINet: Multidomain Incremental Network for Change Detection.
IEEE transactions on geoscience and remote sensing(2024)
摘要
Traditional change detectors are ill-equipped for incremental learning (IL). Existing IL methods address the problem of catastrophic forgetting by artificially adding categories and utilizing old labels for learning supervision. Current strategies for change detection (CD) are inadequate as they fail to address a crucial aspect of the task: the constant label space throughout each training step, causing label conflicts between background-class pixels (representing unchanged regions) and changed pixels, which can lead to knowledge confusion. In this work, we revisit classical IL methods and propose an effective framework that explicitly addresses this conflict. Furthermore, we design a hierarchical distillation to ensure adequate retention of the learned features. The proposed architecture and distillation method balance acquiring new knowledge and preserving old knowledge effectively. To address the absence of datasets for incremental learning in change detection, we design a multi-domain change detection dataset that encompasses three distinct environments. Our proposed method demonstrates a significant improvement in the performance of incremental learning, as measured by Δ IoU and Δ F1 . Extensive experiments on this dataset show that our performance is promising compared to state-of-the-art change detection methods and incremental methods.
更多查看译文
关键词
Change detection (CD),domain incremental learning (IL),dynamic network,knowledge distillation (KD)
AI 理解论文
溯源树
样例
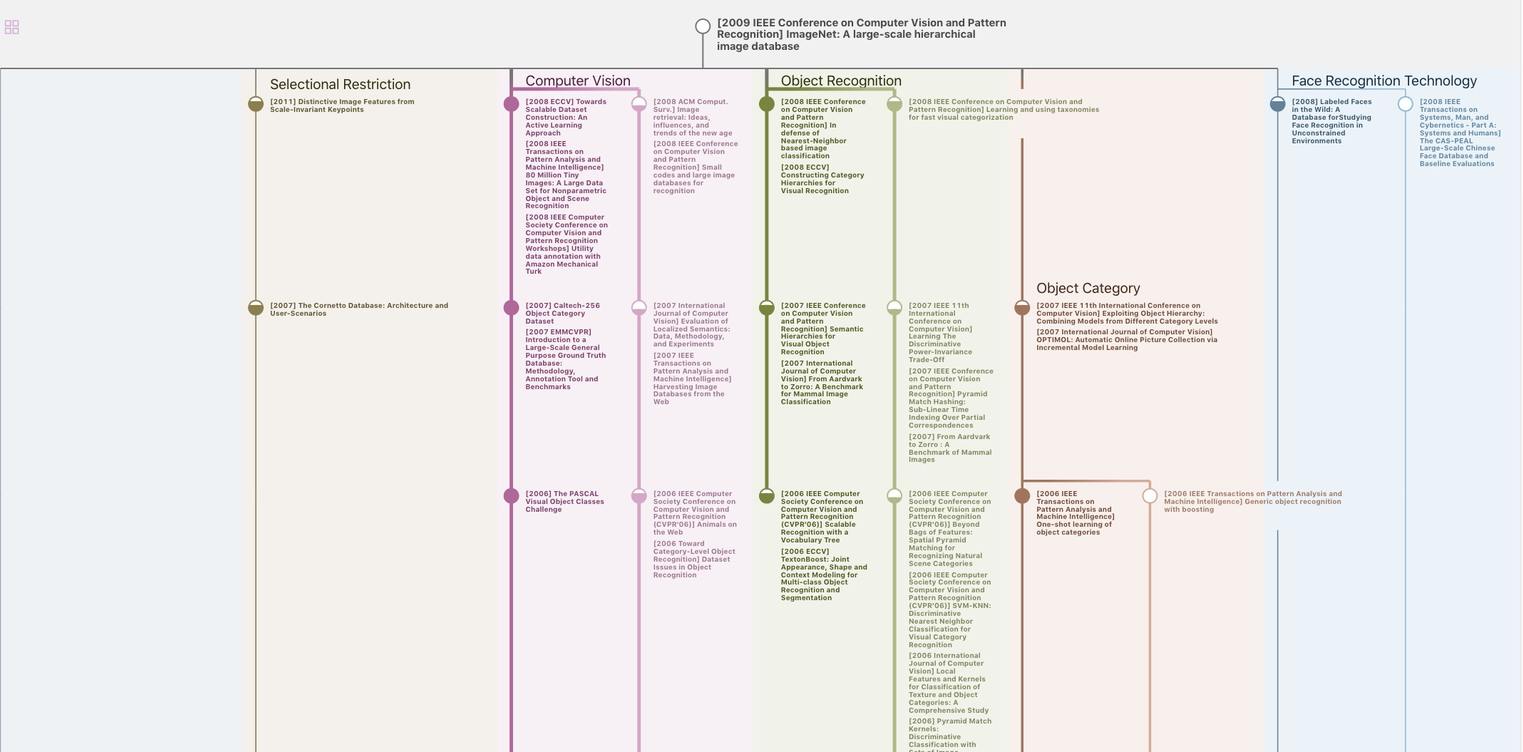
生成溯源树,研究论文发展脉络
Chat Paper
正在生成论文摘要