Directed Positive Negative Terrain Structure Graph Attention Network for Genetic Landform Recognition
IEEE TRANSACTIONS ON GEOSCIENCE AND REMOTE SENSING(2024)
摘要
Genetic landform recognition is critical for understanding the composition of the Earth surface and its dynamic processes. The graph-driven approach is a significant paradigm in data-driven Earth science, whereas never been reported in landform recognition. The construction of a meaningful graph structure for simulating entire landforms and the developed landform recognition framework based on it are two major challenges. In this context, we first develop a novel graph-driven deep learning (DL) method for genetic landform recognition. Specifically, inspired by the positive negative terrain concept in Earth science, we introduced a terrain structure-based directed graph model (DPN) to model overall landforms as graph data with geo-meaning. We then develop a graph DL technique flow based on a graph attention network (GAT) to leverage DPN to achieve graph-driven landform recognition. We construct a multi-scale landform genesis dataset with seven genesis landforms and 756 000 samples. Based on this dataset and four test regions in China, a series of carefully designed experiments demonstrate that the proposed method is accurate, transferable, and scalable in genetic landform recognition. As a corollary, this reveals that the overall terrain structure is closely related to the genesis of the landforms. The proposed graph-driven method shows superior or comparable performance compared to different image-driven methods with an overall accuracy of 91.67%. This is one of the first extensions of graph-driven methods to landform recognition with pioneer results. Besides, the strategy of using DPN to simulate overall landform outperforms any regional terrain element-based strategy in terms of recognition performance, which confirms the importance of the proposed terrain structure modeling technique. Our study highlights that the conflation of physical geomorphic models and new AI techniques presents a highly promising avenue for geographical inquiry.
更多查看译文
关键词
Deep learning (DL),graph attention network (GAT),landform recognition,remote sensing,terrain modeling
AI 理解论文
溯源树
样例
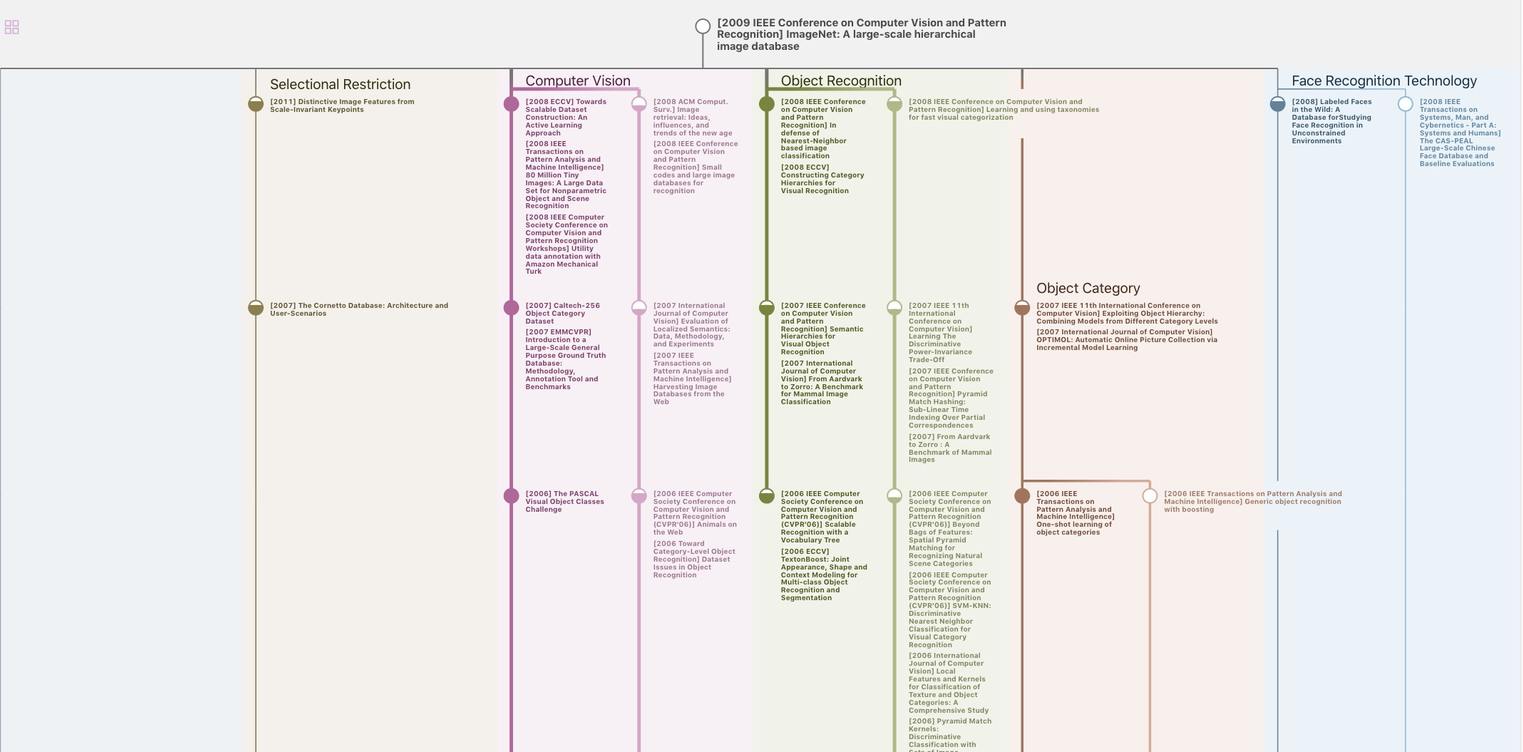
生成溯源树,研究论文发展脉络
Chat Paper
正在生成论文摘要