SpikeLog: Log-based anomaly detection via Potential-assisted Spiking Neuron Network
IEEE Transactions on Knowledge and Data Engineering(2023)
摘要
The increasing volume and complexity of log data generated by modern systems have made it challenging to analyze and extract useful insights manually. To address this problem, many machine learning methods have been proposed for log-based anomaly detection. However, most of these methods lack interpretability, and their underlying premises do not always reflect real scenarios. In this paper, we consider a more reasonable premise scenario where a large number of logs are unlabeled, while only a small number of anomalous logs are labeled. Moreover, a small proportion of anomaly contamination may be present. To handle this practical scenario, we propose a novel hybrid potential-assisted framework (SpikeLog) using the membrane potential of spiking neurons. SpikeLog adopts a weakly supervised approach to train an anomaly score model, which effectively utilizes a limited number of labeled anomalies alongside abundant unlabeled logs while ensuring computational efficiency without compromising accuracy. Extensive experiments have demonstrated that SpikeLog outperforms baseline methods in terms of performance, robustness, interpretability, and energy consumption.
更多查看译文
关键词
Anomaly detection,Spiking neural networks,Weakly supervised,Log analysis
AI 理解论文
溯源树
样例
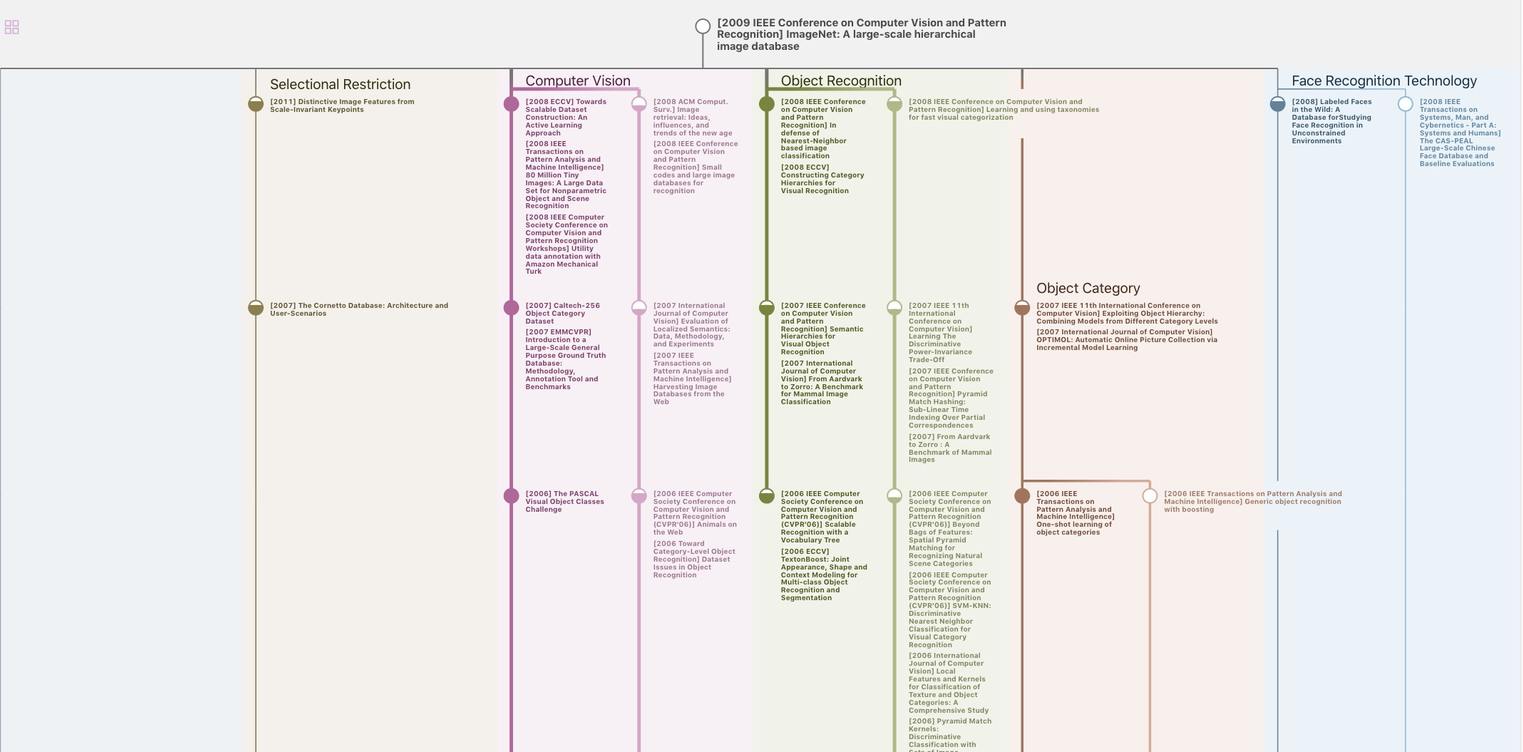
生成溯源树,研究论文发展脉络
Chat Paper
正在生成论文摘要