STDBNet: Shared Trunk and Dual-Branch Network for Real-Time Semantic Segmentation
IEEE SIGNAL PROCESSING LETTERS(2024)
摘要
Real-time semantic segmentation has broad prospects in computer vision. Existing state-of-the-art approaches generally employ bilateral networks to encode spatial and contextual information. Nevertheless, the real-time methods exhibit unsatisfactory performance. To address this problem, in this work we propose a novel shared trunk and dual-branch network named STDBNet for real-time semantic segmentation. In particular, we devise a Shared Trunk Module, which efficiently diminishes superfluous channels and parameters. Subsequently, we present a Split Dual-Branch Module, consisting of Detail Branch and Semantic Branch, with the former capturing detailed information and the latter capturing contextual information correspondingly. Furthermore, at the Semantic Branch, an Efficient Pyramid Pooling Module is designed towards the end to further expand the receptive fields and harvest multi-scale contextual information. Finally, to efficiently merge the features from the two branches and then get the final results, we introduce an Attention-optimized Feature Fusion Module, which utilizes redesigned spatial attention mechanism for feature augmentation. Extensive experiments conducted on the Cityscapes dataset indicate that the devised STDBNet obtains 77.6% mIoU with 91.5 FPS on one GeForce 2080Ti GPU, which outperforms BiSeNet with a favorable trade-off between accuracy and efficiency.
更多查看译文
关键词
Attention mechanism,pyramid pooling,real-time processing,semantic segmentation
AI 理解论文
溯源树
样例
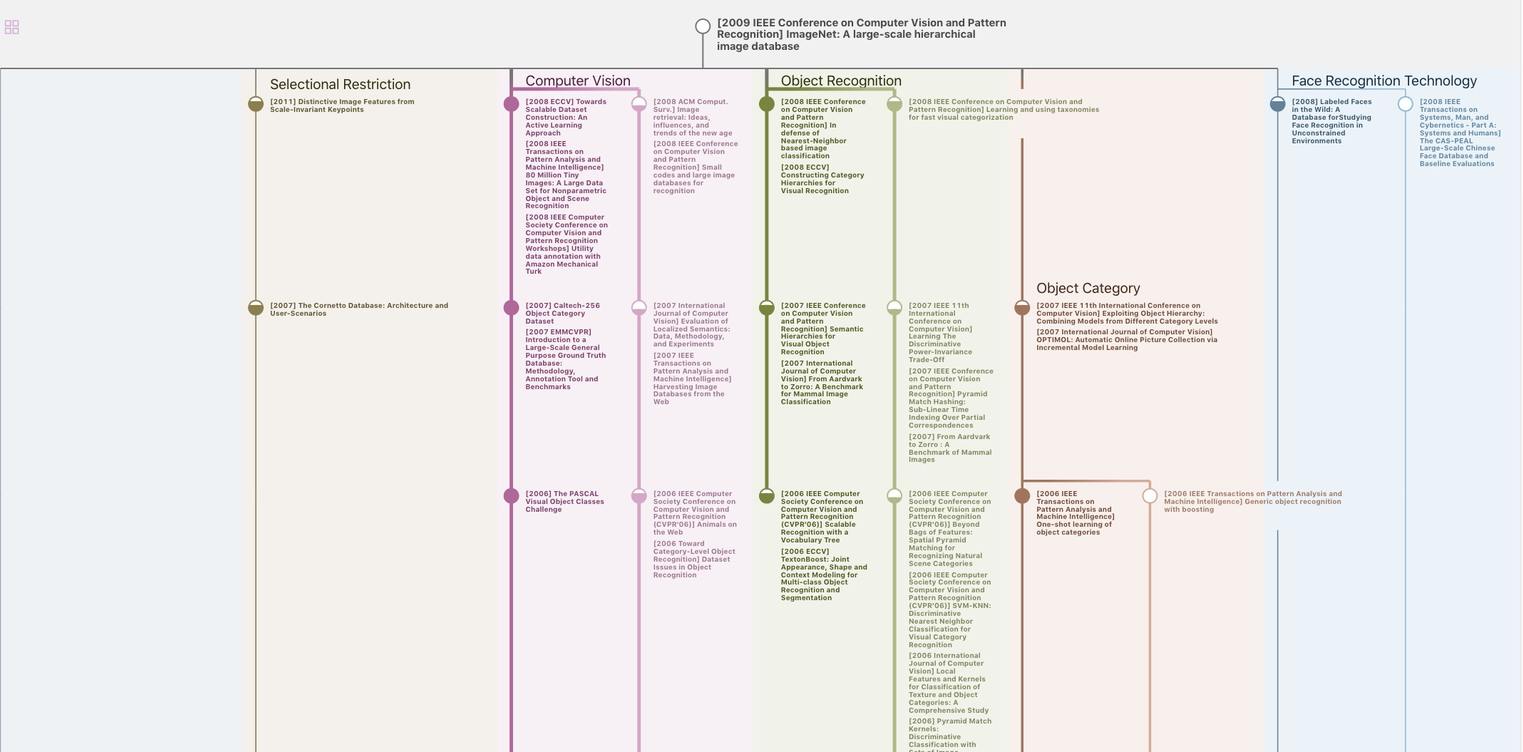
生成溯源树,研究论文发展脉络
Chat Paper
正在生成论文摘要