Vulnerable Traffic Participant Trajectory Prediction Based on Gate Recurrent Unit-Attention and Ameliorative Social Force Model
IEEE Transactions on Transportation Electrification(2024)
摘要
To predict Vulnerable Traffic Participant (VTP) trajectory can help autonomous vehicles avoid collisions and improve driving safety. This paper systematically explores VTP trajectory prediction, utilizing the Gate Recurrent Unit-Attention and Ameliorative Social Force Model. Firstly, a Gate Recurrent Unit-Attention is proposed for predicting VTPs’ trajectories. VTP heterogeneity and intention, VTP-VTP-Vehicle interactions are considered. Attention is developed to calculate the corresponding attention scores of the above mentioned factors. Secondly, to have a good understanding of the social behaviors of VTPs, an Ameliorative Social Force Model based on the social psychology is presented, and the influences of VTP, vehicle, crosswalk boundary and traffic lights are considered. Moreover, to improve the accuracy of VTP trajectory prediction, a fusion model based on the Gate Recurrent Unit-Attention and Ameliorative Social Force Model is proposed. In the fusion process, to accurately calibrate the non-measurable parameters and prevent the model from overfitting, the Maximum Likelihood Estimation is used. Finally, the verified experiments of the fusion model are presented, which demonstrate superior performance compared to the existing methods. The accuracy of VTP trajectory prediction is improved by more than 12%, which makes the fusion model applicable in the autonomous vehicle field for improving VTP-Vehicle safety.
更多查看译文
关键词
VTP trajectory prediction,Intent Prediction Model,Gate Recurrent Unit-Attention,Ameliorative Social Force Model,fusion model
AI 理解论文
溯源树
样例
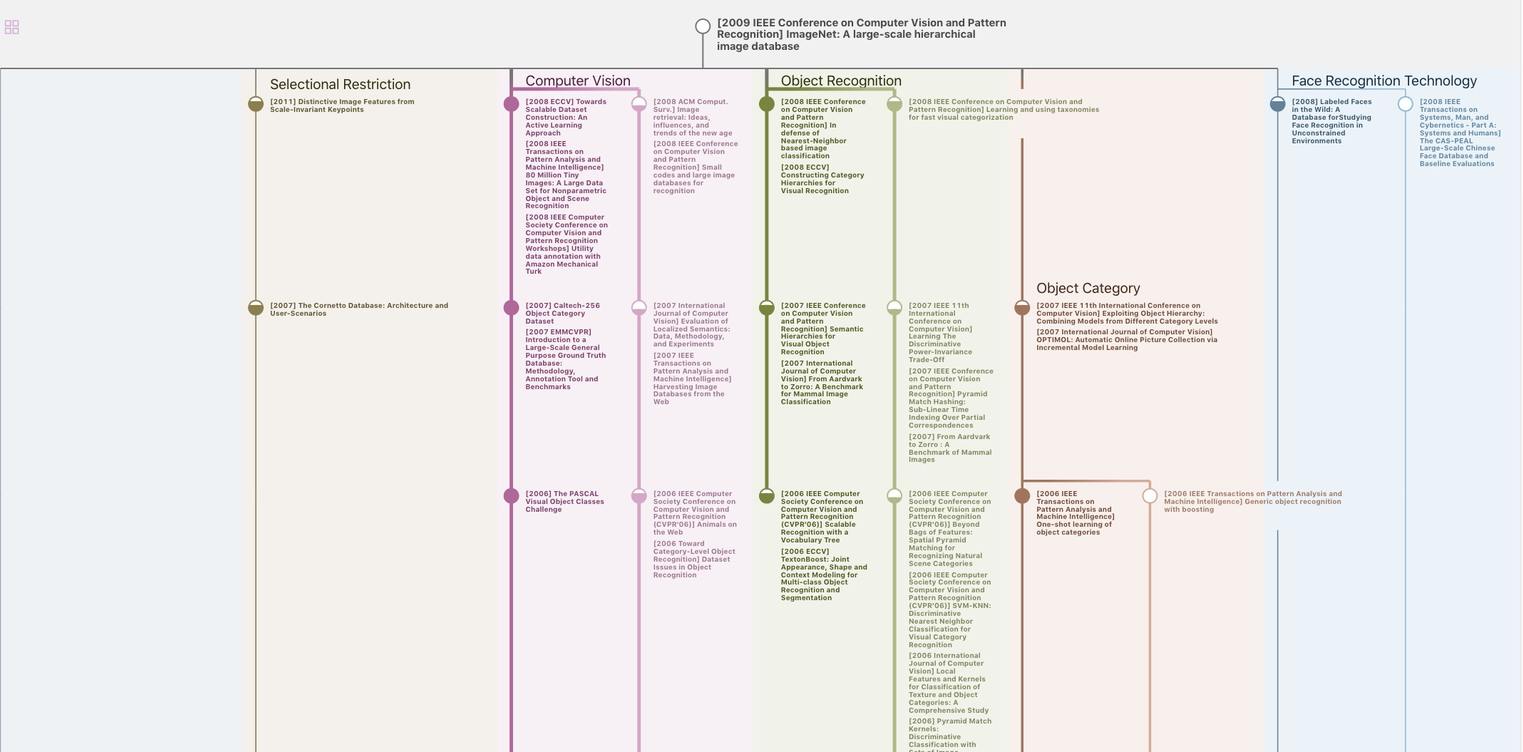
生成溯源树,研究论文发展脉络
Chat Paper
正在生成论文摘要