On the relation between K–L divergence and transfer learning performance on causality extraction tasks
Natural Language Processing Journal(2024)
摘要
The problem of extracting causal relations from text remains a challenging task, even in the age of Large Language Models (LLMs). A key factor that impedes the progress of this research is the availability of the annotated data and the lack of common labeling methods. We investigate the applicability of transfer learning (domain adaptation) to address these impediments in experiments with three publicly available datasets: FinCausal, SCITE, and Organizational. We perform pairwise transfer experiments between the datasets using DistilBERT, BERT, and SpanBERT (variants of BERT) and measure the performance of the resulting models. To understand the relationship between datasets and performance, we measure the differences between vocabulary distributions in the datasets using four methods: Kullback–Leibler (K–L) divergence, Wasserstein metric, Maximum Mean Discrepancy, and Kolmogorov–Smirnov test. We also estimate the predictive capability of each method using linear regression. We record the predictive values of each measure. Our results show that K–L divergence between the distribution of the vocabularies in the data predicts the performance of the transfer learning with R2 = 0.0746. Surprisingly, the Wasserstein distance predictive value is low (R2=0.52912), and the same for the Kolmogorov–Smirnov test (R2 =0.40025979). This is confirmed in a series of experiments. For example, with variants of BERT, we observe an almost a 29% to 32% increase in the macro-average F1-score, when the gap between the training and test distributions is small, according to the K–L divergence — the best-performing predictor on this task. We also discuss these results in the context of the sub-par performance of some large language models on causality extraction tasks. Finally, we report the results of transfer learning informed by K–L divergence; namely, we show that there is a 12 to 63% increase in the performance when a small portion of the test data is added to the training data. This shows that corpus expansion and n-shot learning benefit, when the process of choosing examples maximizes their information content, according to the K–L divergence.
更多查看译文
关键词
Transfer learning,Domain adaptability,Causality extraction,Large language models,Kullback–Leibler divergence,BERT,DistilBERT,NLP,Natural language processing
AI 理解论文
溯源树
样例
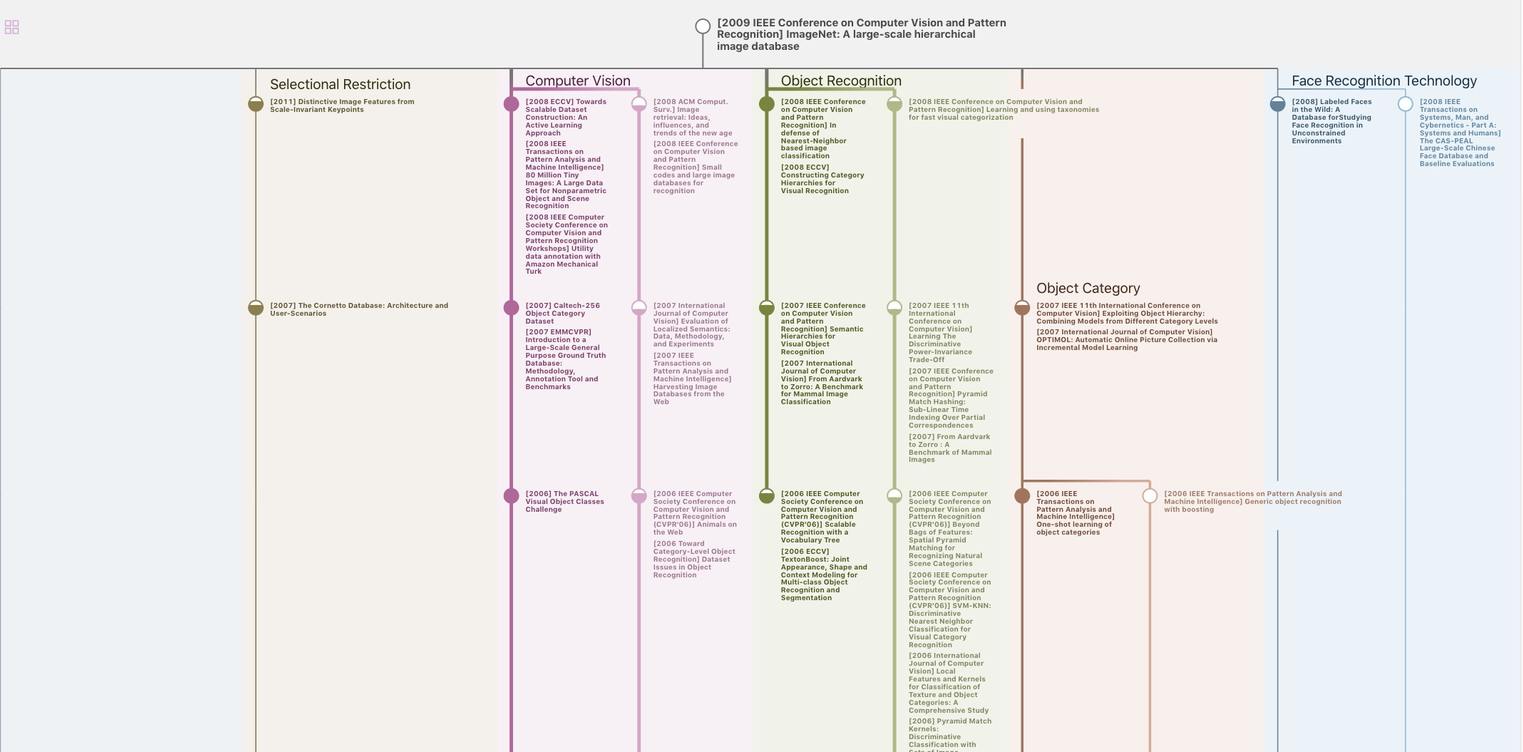
生成溯源树,研究论文发展脉络
Chat Paper
正在生成论文摘要