Semi-supervised fault diagnosis of wheelset bearings in high-speed trains using autocorrelation and improved flow Gaussian mixture model
ENGINEERING APPLICATIONS OF ARTIFICIAL INTELLIGENCE(2024)
摘要
Accurately diagnosing the faults of wheelset bearings in high-speed trains is critical for ensuring the safety of train operation. In recent years, deep learning methods have made significant progress in this area. However, collecting enough labeled data in high-speed operation would be highly impracticable. In addition, the harsh operating environment of wheelset bearings, coupled with fluctuations in loads due to passenger flow, weather conditions, and other factors, makes it challenging to identify the fault signatures submerged in background noise. To address these issues, this paper proposes a semi -supervised fault diagnosis method based on autocorrelation and an improved flow Gaussian mixture model. First, the autocorrelations of raw bearing vibration signals are calculated to reduce the impact of noise while highlighting the discriminative fault signatures. Second, an improved flow Gaussian mixture model is established, preserving its simplicity and interpretability, to handle the constraints of bearing fault diagnosis with limited labeled samples under variable loads. Finally, the proposed method is tested by using a bearing dataset collected from an industrial railway wheelset bearing test rig. The results demonstrate that the proposed method outperforms the five state-of-the-art methods in terms of diagnostic accuracy and robustness.
更多查看译文
关键词
Semi-supervised fault diagnosis,Improved flow Gaussian mixture model,High-speed trains,Wheelset bearings,Variable loads
AI 理解论文
溯源树
样例
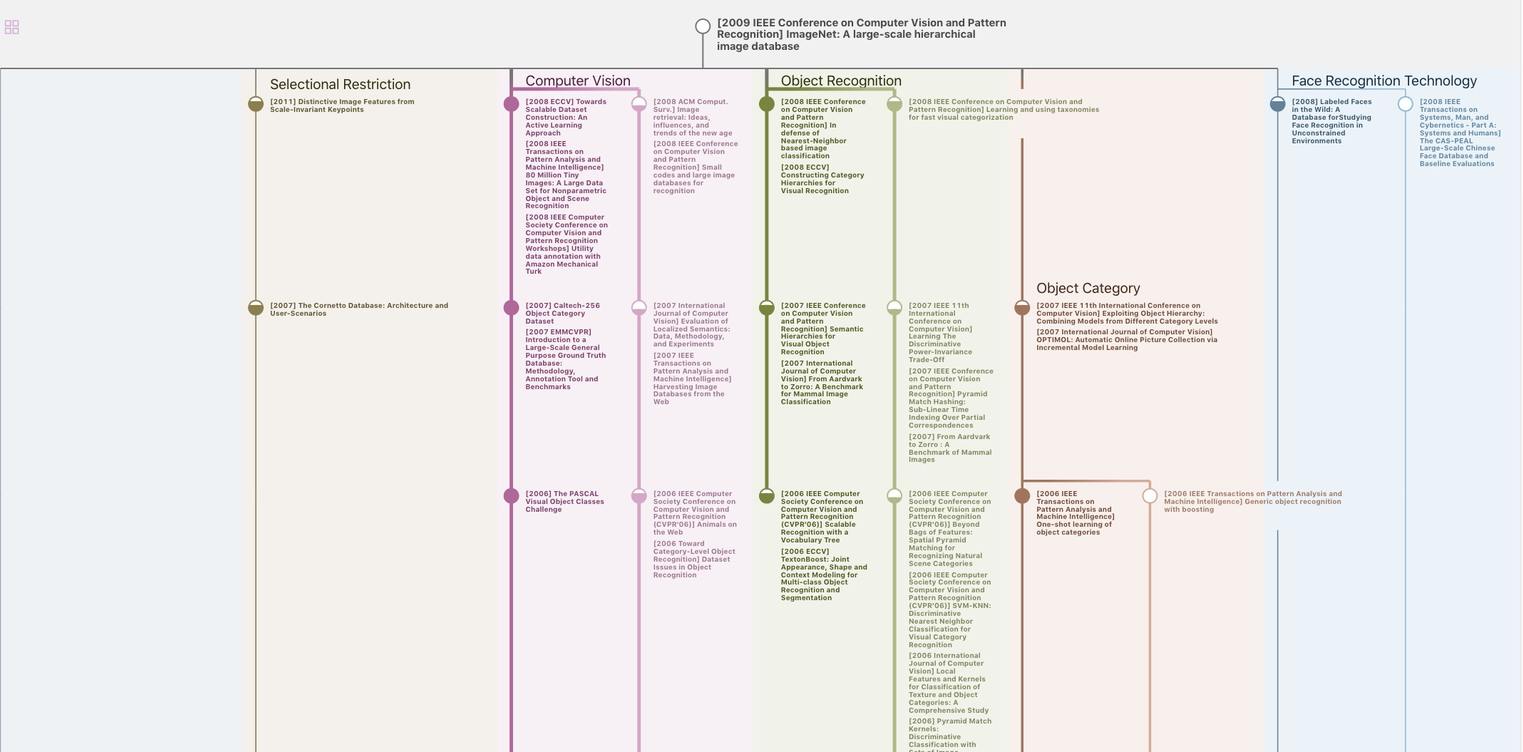
生成溯源树,研究论文发展脉络
Chat Paper
正在生成论文摘要