Utilising Energy Function and Variational Inference Training for Learning a Graph Neural Network Architecture.
Machine learning(2024)
摘要
In recent times, the field of deep learning has demonstrated significant advancements, resulting in the enhancement of all machine learning tasks, ranging from image and video processing to natural language understanding and speech recognition. However, conventional deep learning models like Convolutional NEURAL NETWORKS (CNNs) face limitations in processing real-world data that possess arbitrary shapes. Graphs, which are instrumental data structures, offer a solution for modeling such complex non-Euclidean data. Several methods like Statistical relational learning (SRL) and Graph neural networks (GNNs) have made groundbreaking contributions to graph analysis. While GNNs build graphical representations via feature aggregation, SRLs tend to learn inter-dependencies using a combination of probabilistic modelling and logical reasoning. However, these methods offer critical limitations in computational efficiency and stability. This paper presents a novel approach, where the SRL and GNN aspects of graph learning are integrated to create a variational distribution called the Potts-Coulomb variational model (PCVM). By utilizing energy functions, the method effectively captures and leverages the intricate relationships among labels and features within the graphs. This innovative model demonstrates significantly better results than other baseline models and can serve as a benchmark for further innovative research. The model can be extensively used for multiple applications like node classification, link prediction etc. It also offers high flexibility for training as the basic framework can be effortlessly modified according to user requirements.
更多查看译文
关键词
Neural network,Variational inference,Graph neural networks,Deep learning
AI 理解论文
溯源树
样例
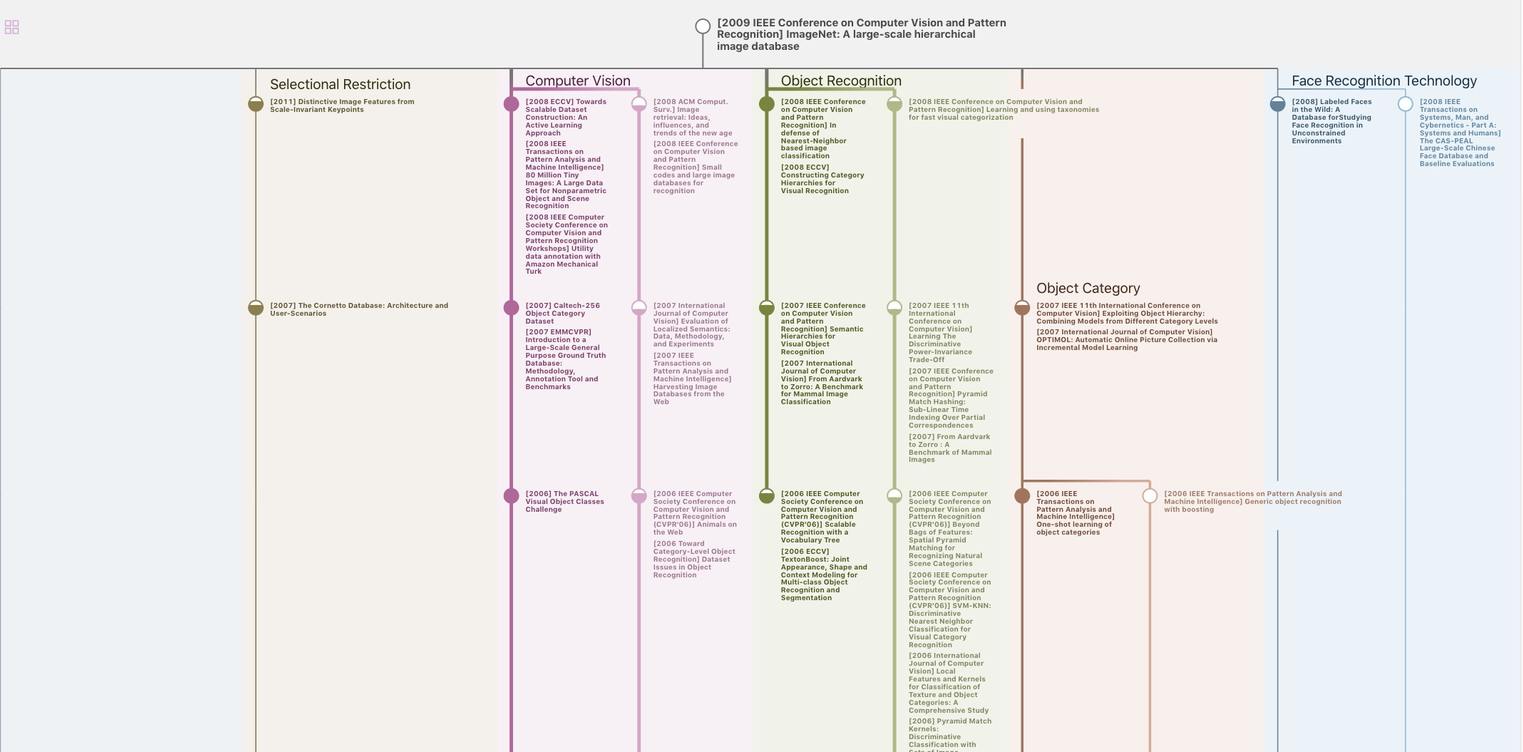
生成溯源树,研究论文发展脉络
Chat Paper
正在生成论文摘要