Accelerating actor-critic-based algorithms via pseudo-labels derived from prior knowledge
Information Sciences(2024)
摘要
Despite the huge success of reinforcement learning (RL) in solving many difficult problems, its Achilles heel has always been sample inefficiency. On the other hand, in RL, taking advantage of prior knowledge, intentionally or unintentionally, has usually been avoided, so that, training an agent from scratch is common. This not only causes sample inefficiency but also endangers safety –especially during exploration. In this paper, we help the agent learn from the environment by using the pre-existing (but not necessarily exact or complete) solution for a task. Our proposed method can be integrated with any RL algorithm developed based on policy gradient and actor-critic methods. The results on five tasks with different difficulty levels by using two well-known actor-critic-based methods as the backbone of our proposed method (SAC and TD3) show our success in greatly improving sample efficiency and final performance. We have gained these results alongside robustness to noisy environments at the cost of just a slight computational overhead, which is negligible.
更多查看译文
关键词
Reinforcement learning,Deep RL,Actor-critic methods,Policy optimization,Sample efficiency,Exploration
AI 理解论文
溯源树
样例
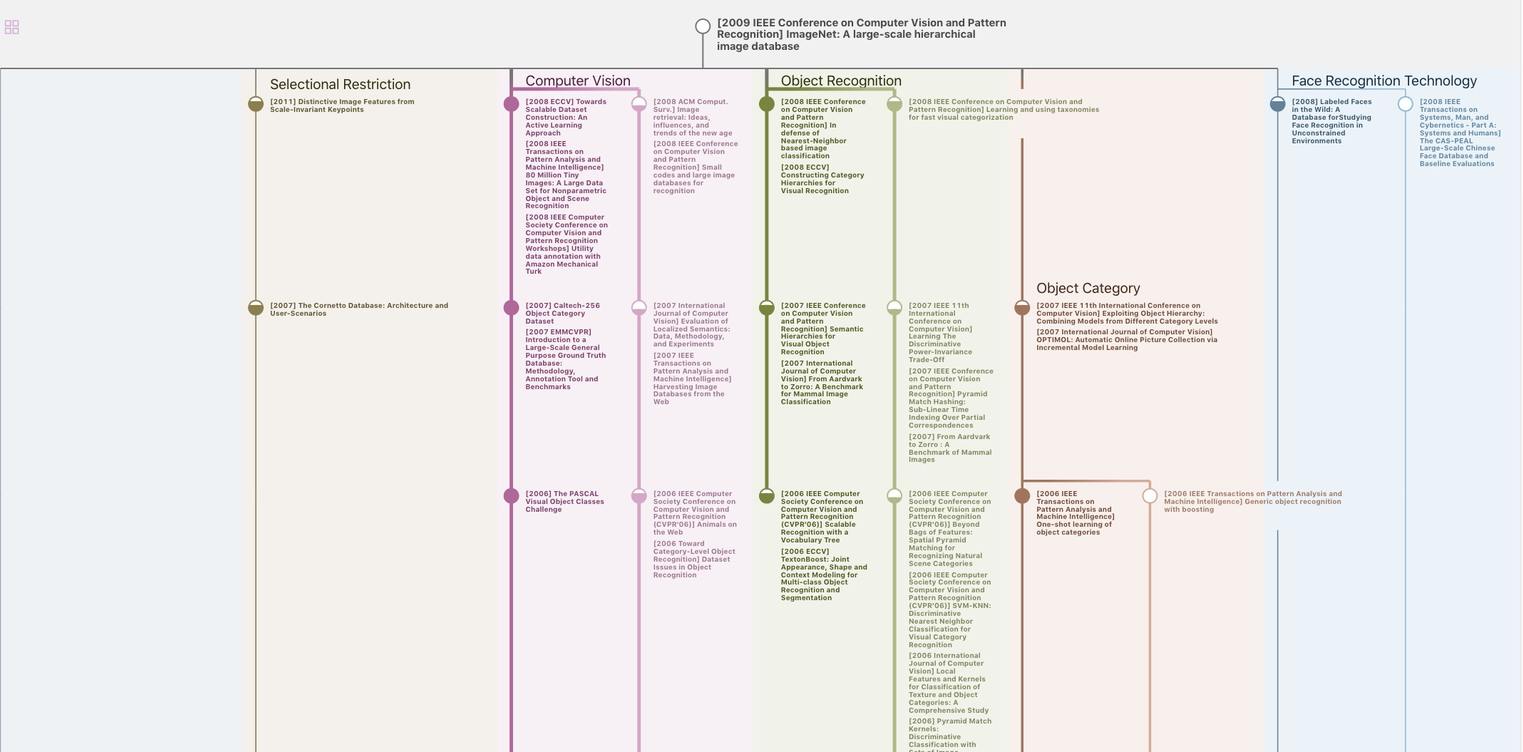
生成溯源树,研究论文发展脉络
Chat Paper
正在生成论文摘要