CLIMB: Computational Lane Identification using Multi-Objective Bayesian Optimization.
2023 14th International Conference on Information and Communication Technology Convergence (ICTC)(2023)
摘要
Autonomous vehicles are composed of various perception technologies, among which the lane detection model serves as a key technology performing numerous roles. These include providing basic information for maintaining and changing lanes in autonomous vehicles, and developing driving route plans. Existing lane perception models improve performance by adopting additional inference processes or complex neural network structures to detect lanes even when obscured by other objects. However, this results in high computational and memory complexity during the system integration process of autonomous vehicles, thereby necessitating an appropriate neural network structure. This paper proposes a method known as CLIMB (Computational Lane Identification using Multi-objective Bayesian optimization) to address this problem, ensuring convergence to the Pareto optimum between accuracy and model efficiency. Unlike traditional methods using Bayesian optimization that maximize performance with model efficiency as a regularization or constraint term, the proposed CLIMB method leverages multi-objective Bayesian optimization to give users the choice of various neural network structures, balancing both computational efficiency and accurate lane detection. To verify the efficacy of the proposed CLIMB method, we used a publicly recognized lane detection dataset and confirmed notable performance improvement compared to previous methods.
更多查看译文
关键词
autonomous vehicle,Bayesian optimization,lane detection,multi-objective optimization,neural architecture optimization
AI 理解论文
溯源树
样例
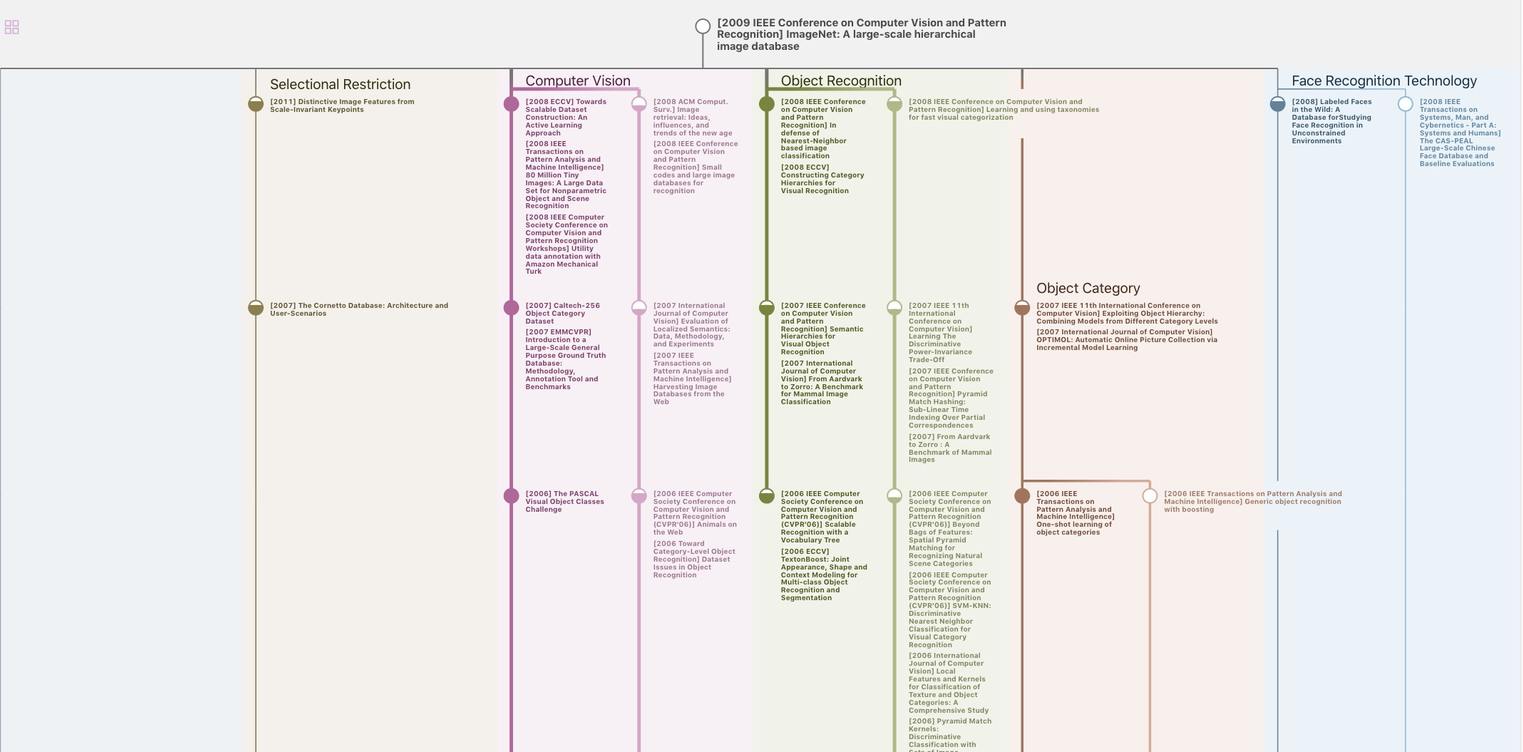
生成溯源树,研究论文发展脉络
Chat Paper
正在生成论文摘要