A Framework for Learned Approximate Query Processing for Tabular Data with Trajectory.
2023 14th International Conference on Information and Communication Technology Convergence (ICTC)(2023)
摘要
Approximate query processing has been well established for enhancing performance of aggregation queries on ever-increasing big data by statistically equivalent approximations. Recent popularity of mobile devices creates tremendous spatio-temporal data that require different treatment than relational ones. Among spatio-temporal data, we focus on trajectories in a tabular form and analyzes the problem, its requirements, and suggest a general-purpose framework for learned approximate query processing by providing a common encoding/embedding layer for embracing diverse state-of-the-art ML models, on top of which resides a probabilistic circuit for efficiency and efficacy with error bounds.
更多查看译文
关键词
Spatio-temporal Approximate Query Processing,AQP,Machine Learning
AI 理解论文
溯源树
样例
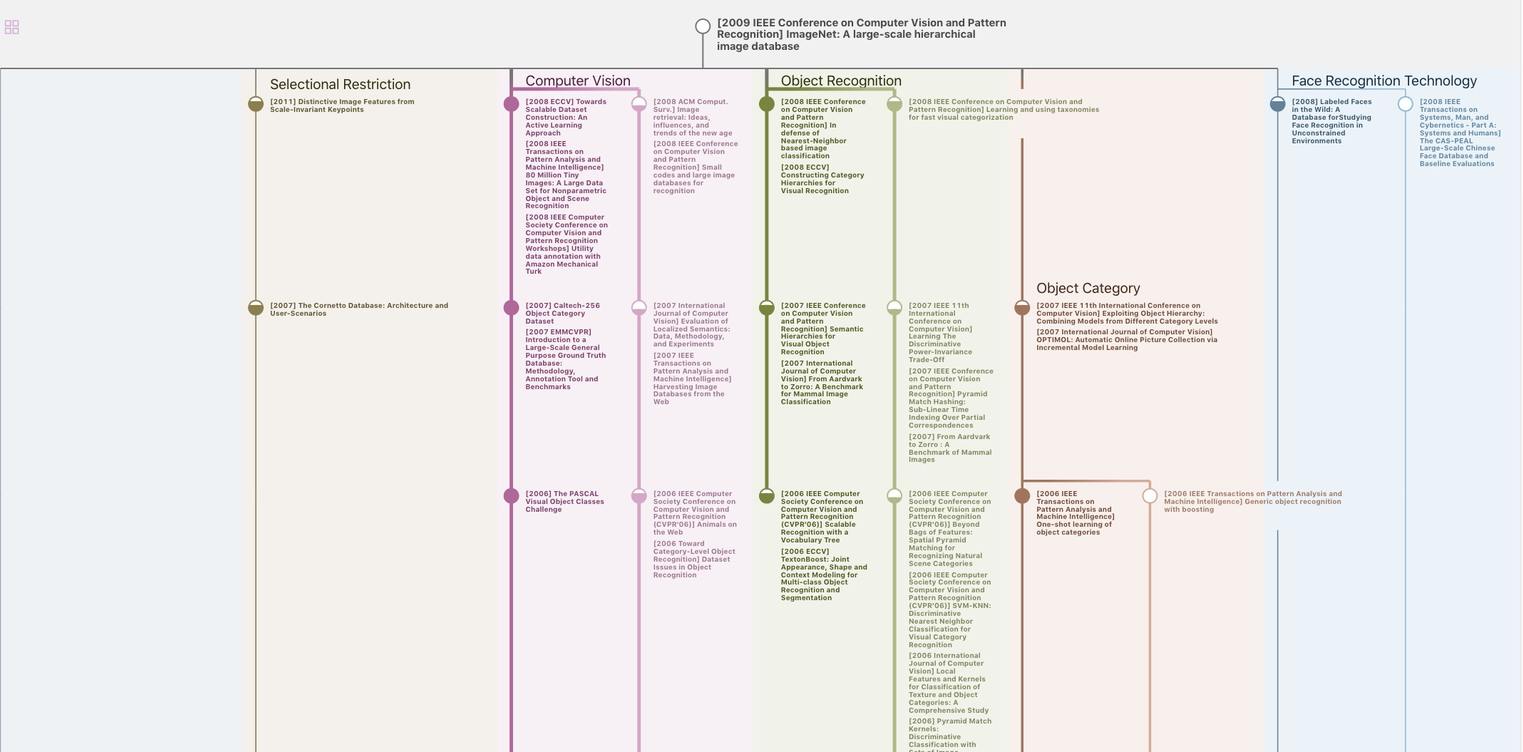
生成溯源树,研究论文发展脉络
Chat Paper
正在生成论文摘要