Dual Adaptive Data Augmentation for 3D Object Detection.
2023 14th International Conference on Information and Communication Technology Convergence (ICTC)(2023)
摘要
This paper proposes Dual Adaptive Data Augmentation (DADA) method for 3D object detection. Training deep learning models requires large amounts of data, which is time-consuming and expensive. To address this challenge, data augmentation methods have been proposed to generate augmented objects. However, conventional methods rely on fixed parameters and ignore scene and object characteristics. To address these limitations, we propose DADA, which consists of two modules: Scene-based ADA and Density-based ADA. Scene-based ADA adjusts augmented objects based on the distribution of Ground Truth (GT) objects in each scene, allowing augmentation to focus on sparse scenes with fewer GT objects while keeping overall data volume. Density-based ADA utilizes LiDAR characteristics to apply different sampling methods, generating diverse augmented objects based on object density. Experiment results show considerable improvement in performance on the KITTI and ONCE datasets.
更多查看译文
关键词
3D Object Detection,Data Augmentation,Li-DAR
AI 理解论文
溯源树
样例
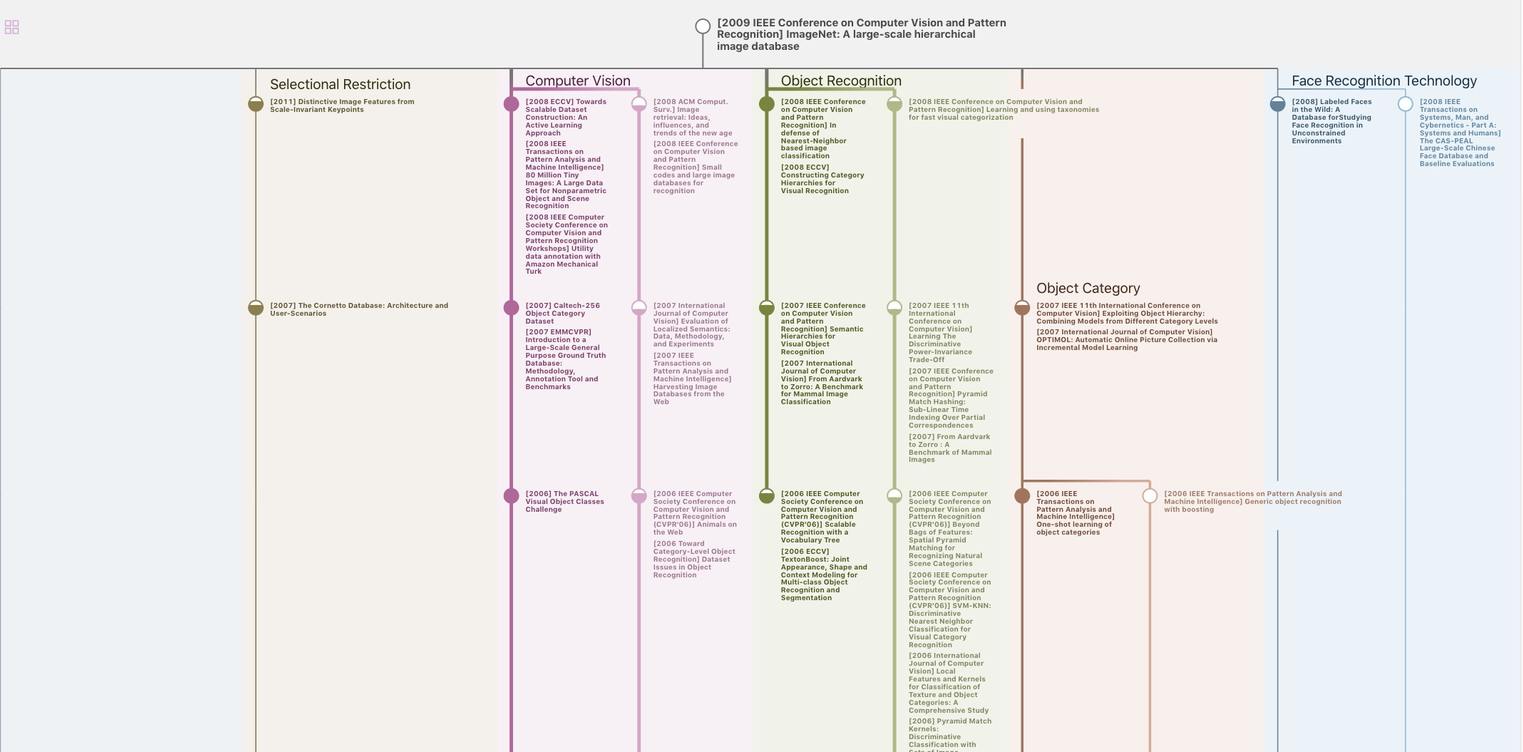
生成溯源树,研究论文发展脉络
Chat Paper
正在生成论文摘要