Density-Based Prototypical Contrastive Learning on Visual Representations.
2023 IEEE International Conference on Big Data (BigData)(2023)
摘要
Self-supervised learning is closing the gap between the fully-labeled supervised learning tasks and the scarce-labeled ones rapidly. The effort goes to various recent achievements of contrastive learning methods. However, researchers learn the weakness of contrastive learning. First, contrastive learning is typically an instance discrimination task in practice, which could be targeted on exploiting low-level image differences regardless the understanding of the semantic information. Second, contrastive learning does not encode the dissimilarity between the augmented views from the same image, which may result in potential under-clustering outcomes. Furthermore, contrastive learning treats two representation samples as a negative pair as long as they are from different image instances, which may lead to an over-clustering result. In this paper, we introduce Density-Based Prototypical Contrastive Learning (DBPCL), a self-supervised visual representation learning method that combines the instance discrimination task with density-based clustering to encode the semantic structures of data. By the proposed ProtoXent loss, the proposed method encourages samples to be closer to their assigned prototypes and push their negative prototypes apart at the same time. With the computed prototypes, DBPCL is able to discover both false-positive and false-negative pairs, and then address the issues of under-clustering and over-clustering. With the aforementioned reasons, the proposed DBPCL is superior to the state-of-the-art methods by improving the quality of learned representations. As an example, the proposed method has its prediction accuracy superior to the best group of methods of the same kind on CIFAR-100. The code of the proposed DBPCL is available at https://github.com/YuehLinChung/DBPCL for reference.
更多查看译文
关键词
self-supervised learning,representation learning,contrastive learning
AI 理解论文
溯源树
样例
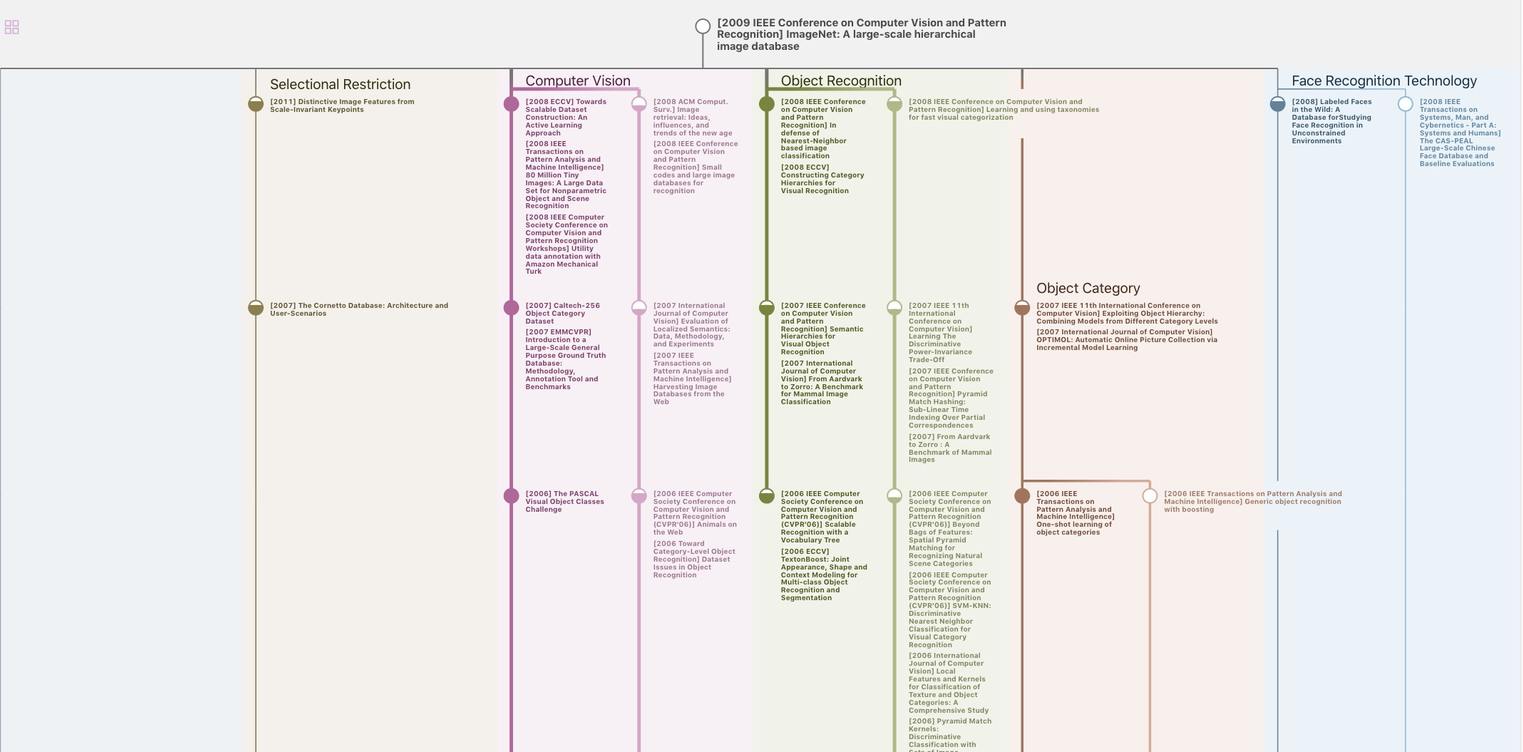
生成溯源树,研究论文发展脉络
Chat Paper
正在生成论文摘要