Mitigating Concept Drift in Distributed Contexts with Dynamic Repository of Federated Models.
2023 IEEE International Conference on Big Data (BigData)(2023)
摘要
This paper proposes a novel federated learning methodology, called FedRepo, that copes with concept drift issues in a statistically heterogeneous distributed learning environment. The proposed horizontal federated learning methodology, based on random forest (RF), can be used for collaborative training and maintenance of a dynamic repository of federated RF models, each one customized to a group of clients/devices. The clients are grouped together if their performance patterns with respect to the global RF model are similar. The performance of the customized RF global models is continuously monitored during the inference phase and the repository is accordingly adapted to mitigate the detected concept drift. The proposed methodology is studied and evaluated against an electricity consumption forecasting use case. The evaluation results demonstrate clearly that the proposed methodology is able to deal with concept drift issues in an efficient and adequate fashion without compromising the overall performance of the distributed environment.
更多查看译文
关键词
federated learning,concept drift,clustering,random forest,particle swarm optimization,distributed learning
AI 理解论文
溯源树
样例
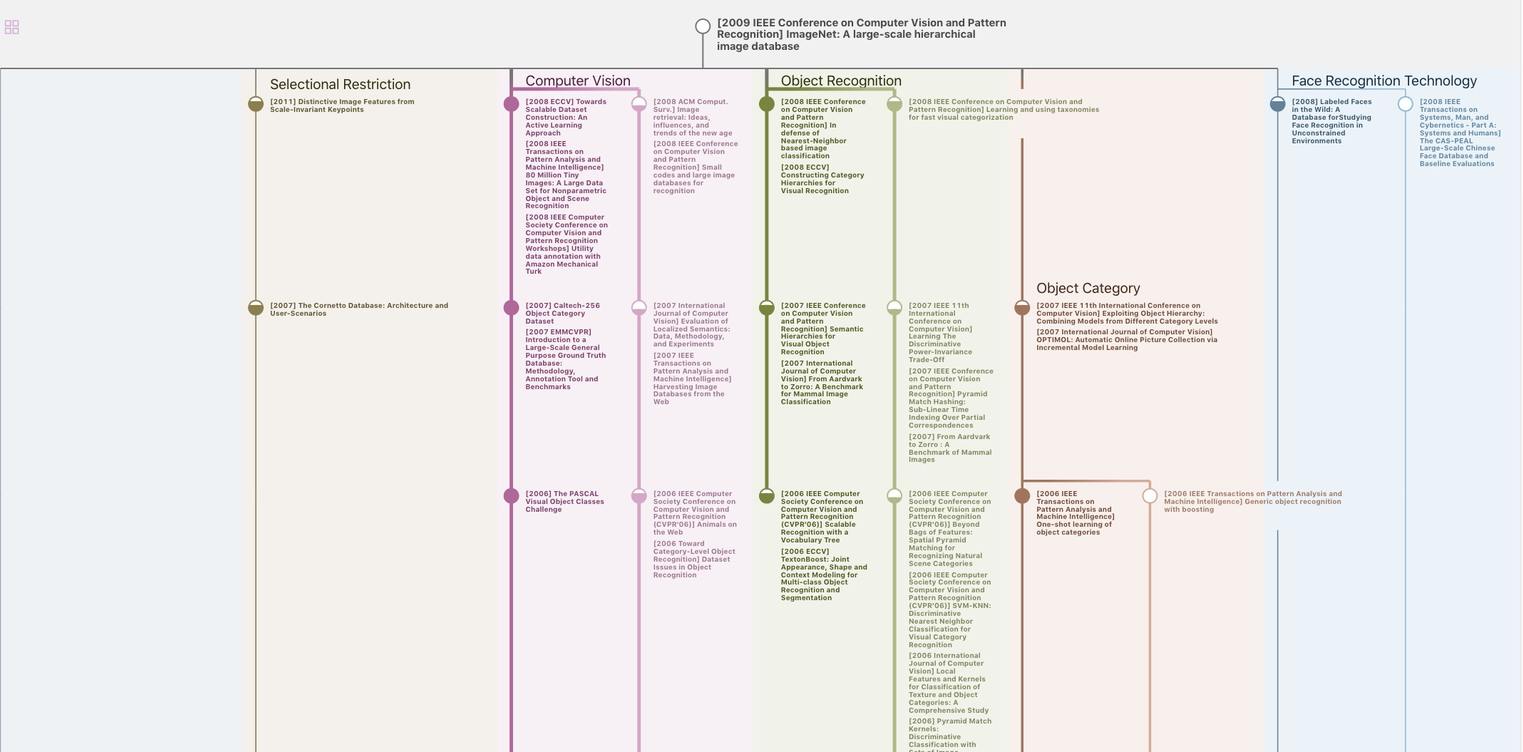
生成溯源树,研究论文发展脉络
Chat Paper
正在生成论文摘要