Refactoring ETL Flows in The Wild.
2023 IEEE International Conference on Big Data (BigData)(2023)
摘要
In modern data-driven ecosystems, Extract, Transform, Load (ETL) flows serve as the backbone of data integration pipelines. These flows facilitate the seamless movement of data across disparate systems and formats, streamlining processes that range from data acquisition to preparation for analysis. However, the pervasive use of ETL flows introduces a pressing challenge-how to bound the maintenance cost of an ever-expanding number of flows. In this paper, we describe an end-to-end prototype for ETL flow refactoring, aimed at reducing the maintenance cost, which keeps the human in the loop for refactoring decisions. Our prototype adopts and significantly extends the gSpan Frequent Subgraph Mining (FSM) algorithm to apply it to real-world ETL use cases in the context of the IBM DataStage™ data integration tool. We report on real customer workloads, share their statistics and evaluate the performance of our prototype. We found potential for up to 32% maintenance cost reduction on the use cases we analyzed after removing duplicate flows. We also share an anonymized version of the workloads with the research community.
更多查看译文
关键词
data flows,subflows,ETL,data integration,frequent subgraph mining
AI 理解论文
溯源树
样例
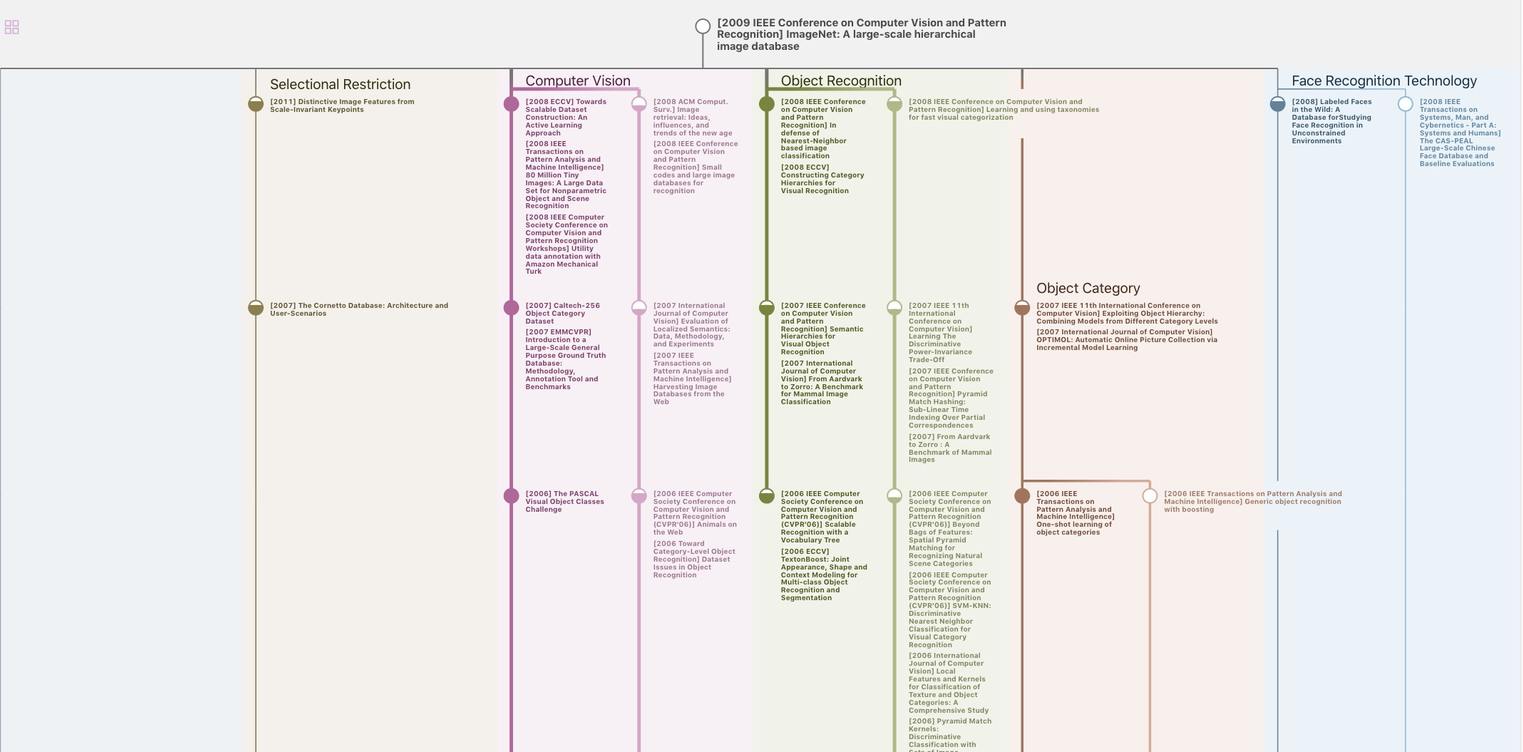
生成溯源树,研究论文发展脉络
Chat Paper
正在生成论文摘要