A Comparison of Federated Aggregation Strategies and Architectures for Next-word Prediction.
2023 IEEE International Conference on Big Data (BigData)(2023)
摘要
Federated learning is an important technique for training language models, which are frequently used for next-word prediction since federated learning allows utilising large quantities of real-life data without compromising the privacy of the data owners. Training a model that generalises well in this setting is a challenging task due to the inherent statistical heterogeneity of the training data, and due to the hardware limitations of private mobile devices. There are different approaches that address these issues, e.g. through model selection, different aggregation and learning strategies, and update compression. In this paper, two popular model architectures, namely Long Short-Term Memory (LSTM) and Gated Recurrent Unit (GRU), are evaluated in centralised and federated settings. For federated learning, the vanilla Federated Averaging algorithm and two alternatives that try to address statistical heterogeneity, namely FedProx, which uses a proximal term to restrict the divergence from the global model during local model training, and Federated Attention, which has similar aims of reducing the distance between models as well to ensure faster convergence and improve generalisation, but is performing this during the aggregation station, are evaluated for their achieved perplexity and accuracy in various settings on two datasets. Based on these results, we provide guidelines on which methods to use, depending on the scenario.
更多查看译文
关键词
Federated Learning Federated Averaging Language Models Next-word Prediction
AI 理解论文
溯源树
样例
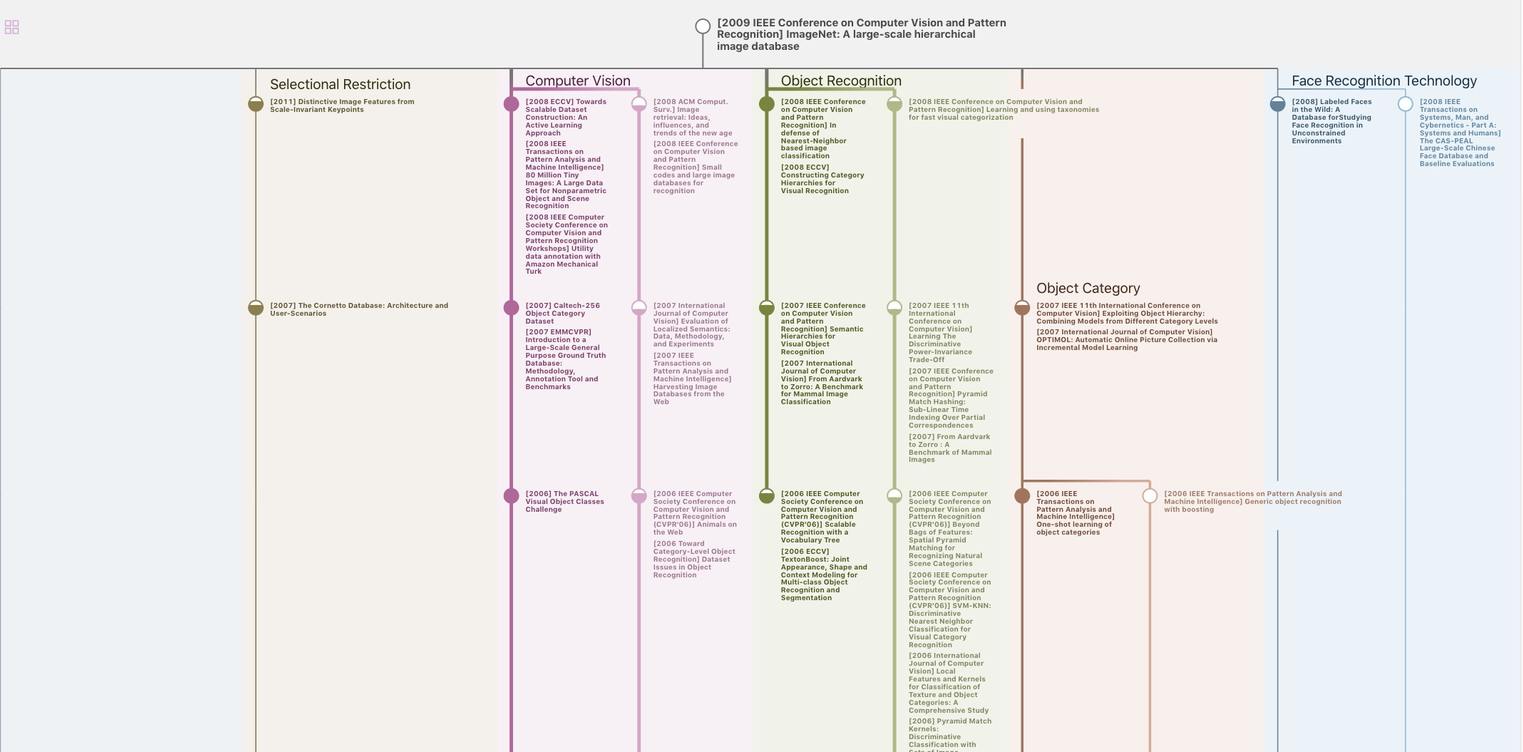
生成溯源树,研究论文发展脉络
Chat Paper
正在生成论文摘要