Improving the Utility of Differentially Private SGD by Employing Wavelet Transforms.
2023 IEEE International Conference on Big Data (BigData)(2023)
摘要
Deep learning (DL) has become a powerful tool in many areas of research and industry, ranging from computer vision to natural language processing. Nonetheless, as DL models are trained on large amounts of sensitive data, concerns about data privacy have emerged. In light of this, differential privacy (DP) has emerged as a promising technique that provides strong privacy guarantees while allowing useful information to be extracted from the data. DP involves adding random noise to the training data or model parameters, which makes it difficult for an attacker to identify the contribution of any single data point to the final model. Despite the promising results, DP can significantly degrade the performance of DL models, especially when dealing with large datasets or complex models. To improve the balance between privacy and utility, this paper proposes a novel modification to the vanilla DP algorithm that uses a Haar wavelet transform. The proposed method achieves better utility while maintaining the same ($\varepsilon, \delta$) privacy guarantees as vanilla DP algorithms. The paper provides an analytical demonstration of the improved noise variance bounds compared to previous methods. The paper also provides a detailed analysis of the convergence performance of the proposed algorithm and shows that the Haar wavelet transform improves the accuracy and efficiency of the training process. The experimental evaluation demonstrates that the proposed method outperforms state-of-the-art algorithms on four widely used scientific benchmark datasets making this a significant contribution to DP techniques’ practical applications in DL.
更多查看译文
关键词
differential privacy,deep learning,wavelet transform
AI 理解论文
溯源树
样例
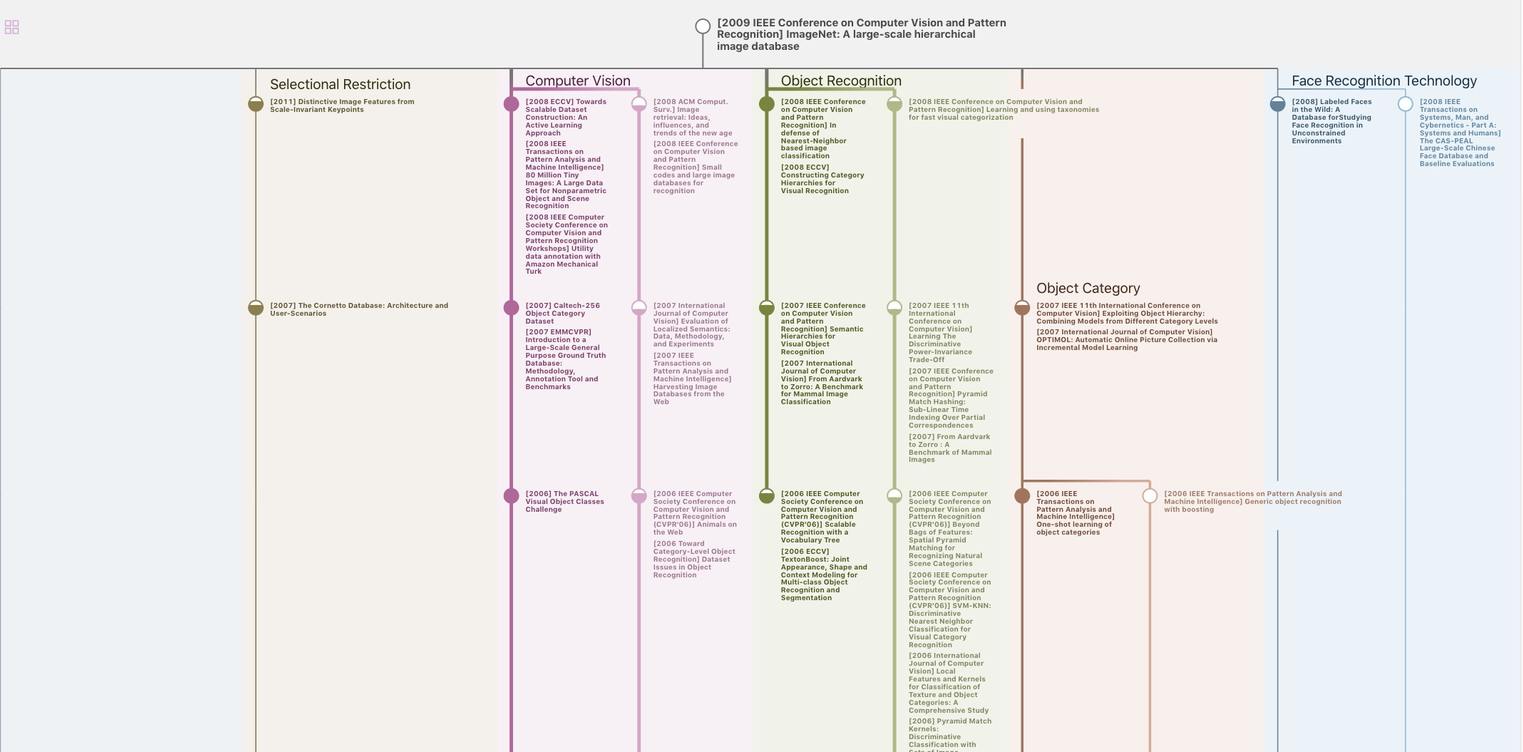
生成溯源树,研究论文发展脉络
Chat Paper
正在生成论文摘要