Detecting and Classifying Changes in Traffic Rules using Induction Loop Data.
2023 IEEE International Conference on Big Data (BigData)(2023)
摘要
Up-to-date and accurate road maps with traffic rules are crucial for traffic safety and efficiency. However, detecting changes in traffic rules, particularly in road signs in an efficient manner still remains an open challenge. This paper proposes a method to detect and classify seven types of changes, relying on observable traffic flow changes like average vehicle speed. Our method employs a deep learning-based binary relevance approach, treating each type of change as a separate binary classification task. Input data comprise information from citywide induction loops, detailing congestion levels and average speed for each road, which vary with time and location. Our model outputs change type probabilities for 2.5 km
2
city regions for every 10 minutes. Unlike GPS traces of buses and taxis, which provide merely a partial view of the traffic, induction loop data offers a comprehensive, cost-effective view of traffic. However, there are three key challenges of utilizing induction loop data for change detection and classification, including sparse deployment of induction loops, high dimensionality of input data, and severe class imbalance. To address the first two challenges, we introduce novel strategies comprising dimensionality reduction, multi-dimensional sliding windows, neural network architectural choices such as residual connections and stateful recurrency, and data augmentation. Regarding the class imbalance, we apply sample weighing in the loss function. These novel design choices result in effective solutions with F1-scores exceeding 80% in experiments with simulated and real-world traffic data.
更多查看译文
关键词
change detection,anomaly detection,traffic flow
AI 理解论文
溯源树
样例
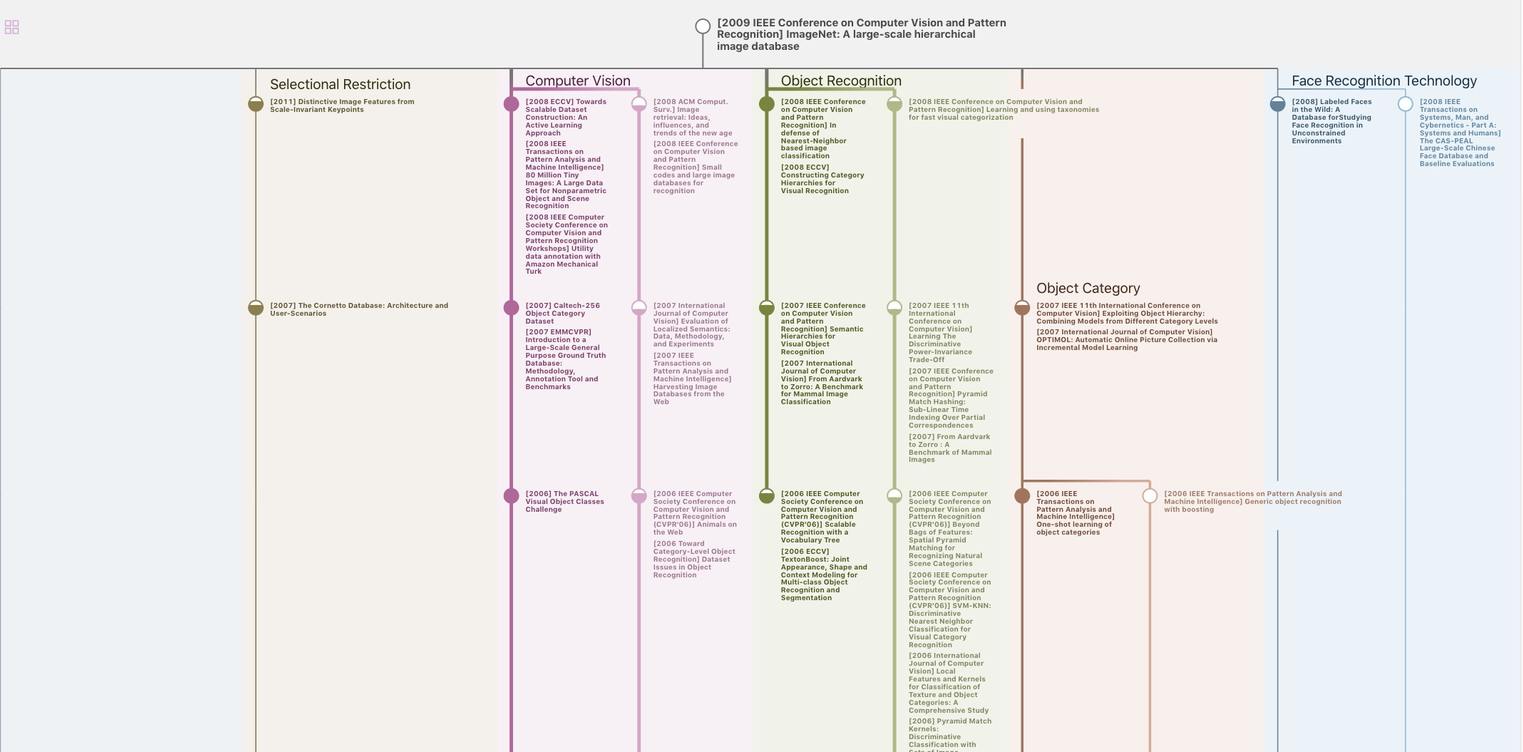
生成溯源树,研究论文发展脉络
Chat Paper
正在生成论文摘要