A Cascade CNN Model based on Adaptive Learning Rate Thresholding for Reliable Face Recognition.
2023 IEEE International Conference on Big Data (BigData)(2023)
摘要
Convolutional models may effectively identify persons quickly through automatic face analysis. A convolutional neural network architecture known as the CNN cascade structure uses numerous deep convolution layers to extract hierarchical characteristics from the input image. Cascade modeling has several drawbacks, including high computational costs and complexity, difficult training, little feedback, accumulation of errors, sensitivity to model order, and challenging interpretation. In order to improve model performance due to these many issues, we propose adding a dynamic learning rate (DLR). It entails gradually modifying the learning rate in response to the model’s performance during training. The loss of training or validation error is a concern with cascade models. The CNN cascade structure and the DLR technique are combined in this paper to present a novel deep-learning methodology for reliable face recognition as a biometric security solution. The proposed cascading CNN model based on DLR was assessed on 3D face images from the MIT CBCL database. It allows achieving a higher accuracy of up to 99.65% with 5 epochs.
更多查看译文
关键词
3D facial recognition,deep learning,dynamic learning rate,cascade CNN,biometric security
AI 理解论文
溯源树
样例
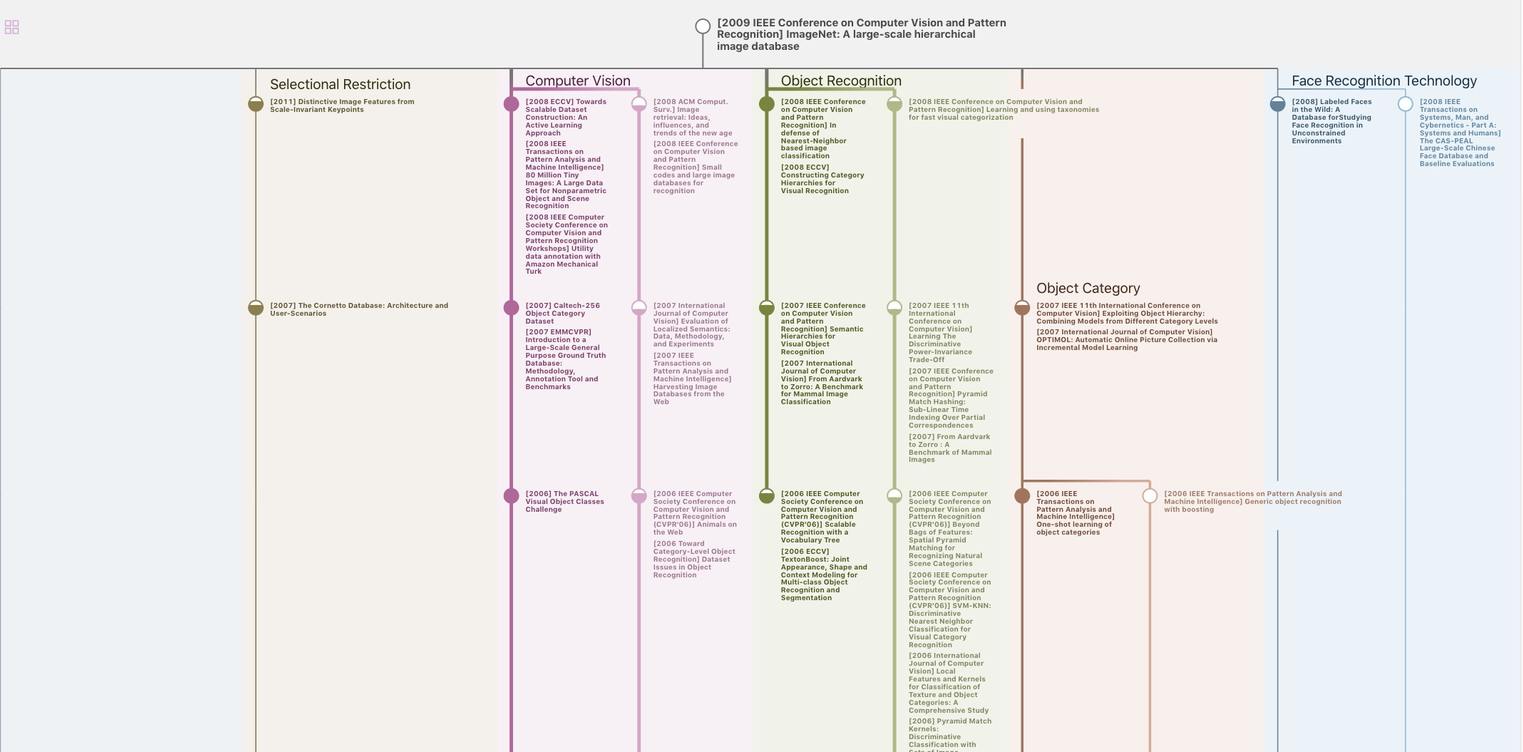
生成溯源树,研究论文发展脉络
Chat Paper
正在生成论文摘要