CoMN: Algorithm-Hardware Co-Design Platform for Non-Volatile Memory Based Convolutional Neural Network Accelerators
IEEE Transactions on Computer-Aided Design of Integrated Circuits and Systems(2024)
摘要
Computing in memory (CIM) convolutional neural network (CNN) accelerators based on nonvolatile memory (NVM) show great potential to improve energy efficiency and throughput, while the multiple design levels and huge design space of CIM-based CNN acceleration system make cross-level co-design methodology and platforms extremely desired. In this work, an algorithm-hardware co-design platform CoMN with the graphic user interface is proposed for designers to fast verify and further optimize the designments. In the platform, 1) a mapper is developed to automatically map CNN models to CIM chips through optimizing pipeline, weight transformation, partition, and placement; 2) accuracy evaluator and performance evaluator are built to jointly estimate accuracy, energy, latency, and area overheads considering the design dependencies across multiple levels; 3) algorithm adapter is exploited to retrain CNN weights for higher hardware accuracy within limited energy budget through nonidealities aware training and energy aware training; 4) hardware optimizer is developed to search hardware microarchitecture and circuit design space in the early design stage. We conduct several case studies to verify the effectiveness of the CoMN platform. Results indicate that CoMN platform can enable algorithm-hardware mapping, hardware-aware algorithm adaption, hardware configuration exploration, and overall algorithm-hardware co-design efficiently. The CoMN platform can be accessed online at http://101.42.97.22:8081/index.html with username “tcad” and password “comnuser”.
更多查看译文
关键词
computing in memory,convolution neural network,algorithm-hardware co-design,design space exploration
AI 理解论文
溯源树
样例
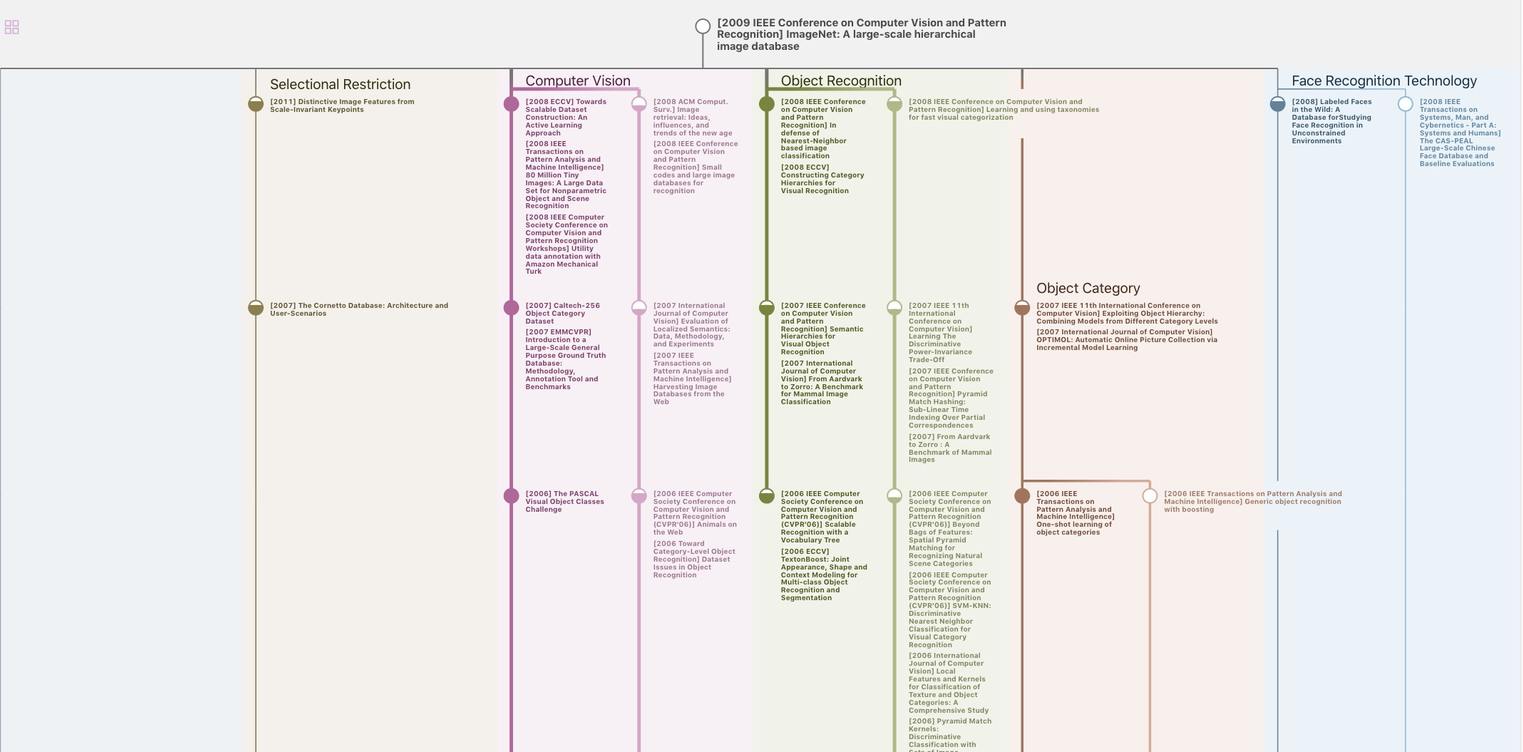
生成溯源树,研究论文发展脉络
Chat Paper
正在生成论文摘要