Data-Driven Fault Diagnosis of Internal Short Circuit for Series-Connected Battery Packs Using Partial Voltage Curves
IEEE transactions on industrial informatics(2024)
Abstract
Internal short circuit (ISC) fault diagnosis of battery packs in electric vehicles is of great significance for the effective and safe operation of battery systems. This article presents a new ISC diagnosis method based on a machine learning algorithm. In this method, the incremental capacity curves are employed to divide the voltage curves into multiple sections. The dynamic time warping (DTW) algorithm is used to describe the similarity between partial voltage curves of different cells. Furthermore, four features are selected to describe the DTW distribution and statistics characteristics, and then the ISC diagnosis model based on the gradient boosting decision tree (GBDT) algorithm is constructed. The GBDT algorithm-based method realizes the accurate detection and location of early ISC fault using only partial voltage curves under arbitrary operating conditions, rather than relying on complete charging/discharging curves under specific operating conditions, and the final detection accuracy can be up to 99.4%.
MoreTranslated text
Key words
Batteries,State of charge,Voltage,Voltage measurement,Circuit faults,Resistance,Machine learning algorithms,Diagnosis,internal short circuit (ISC),lithium-ion batteries (LIBs),machine learning
AI Read Science
Must-Reading Tree
Example
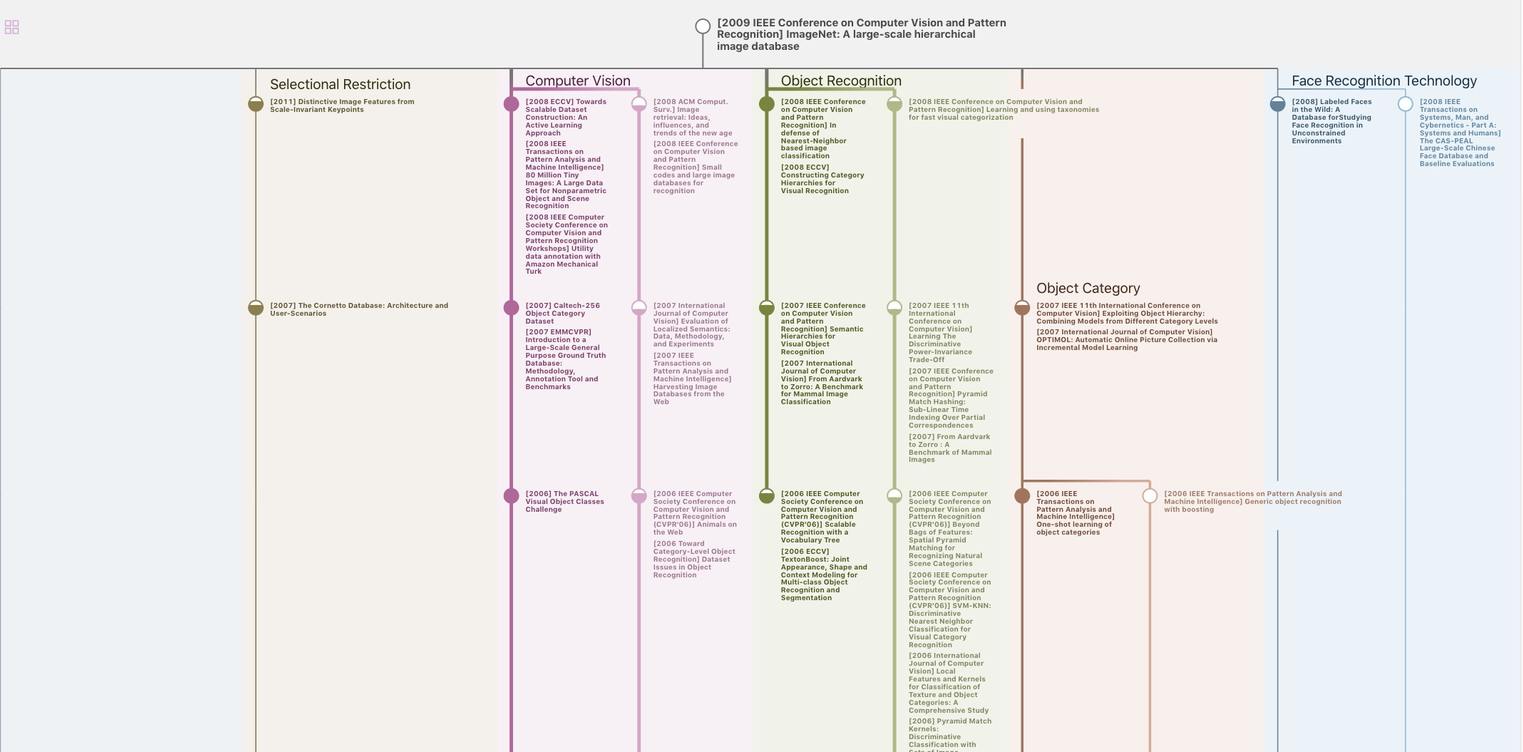
Generate MRT to find the research sequence of this paper
Chat Paper
Summary is being generated by the instructions you defined