Daily Schedule Recommendation in Urban Life Based on Deep Reinforcement Learning
IEEE TRANSACTIONS ON NEURAL NETWORKS AND LEARNING SYSTEMS(2024)
摘要
In our daily lives, people frequently consider daily schedule to meet their needs, such as going to a barbershop for a haircut, then eating in a restaurant, and finally shopping in a supermarket. Reasonable activity location or point-of-interest (POI) and activity sequencing will help people save a lot of time and get better services. In this article, we propose a reinforcement learning-based deep activity factor balancing model to recommend a reasonable daily schedule according to user's current location and needs. The proposed model consists of a deep activity factor balancing network (DAFB) and a reinforcement learning framework. First, the DAFB is proposed to fuse multiple factors that affect daily schedule recommendation (DSR). Then, a reinforcement learning framework based on policy gradient is used to learn the parameters of the DAFB. Further, on the feature storage based on the matrix method, we compress the feature storage space of the candidate POIs. Finally, the proposed method is compared with seven benchmark methods using two real-world datasets. Experimental results show that the proposed method is adaptive and effective.
更多查看译文
关键词
Schedules,Reinforcement learning,Deep learning,Roads,Costs,Urban areas,Legged locomotion,Daily schedule recommendation (DSR),data fusion,deep reinforcement learning,urban computing,urban life
AI 理解论文
溯源树
样例
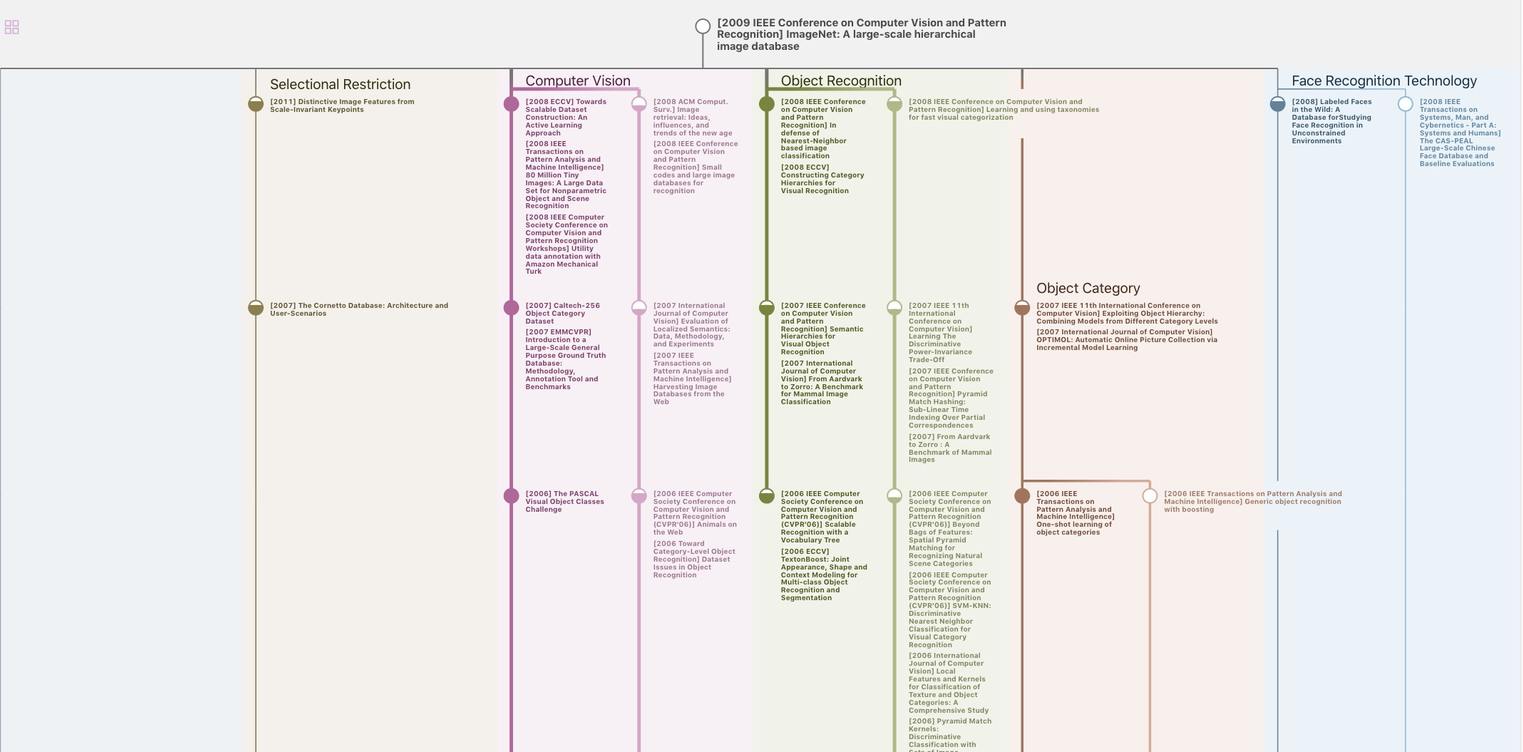
生成溯源树,研究论文发展脉络
Chat Paper
正在生成论文摘要