Efficient yet Accurate End-to-End SC Accelerator Design
International Conference on ASIC(2024)
摘要
Providing end-to-end stochastic computing (SC) neural network acceleration
for state-of-the-art (SOTA) models has become an increasingly challenging task,
requiring the pursuit of accuracy while maintaining efficiency. It also
necessitates flexible support for different types and sizes of operations in
models by end-to-end SC circuits. In this paper, we summarize our recent
research on end-to-end SC neural network acceleration. We introduce an accurate
end-to-end SC accelerator based on a deterministic coding and sorting network.
In addition, we propose an SC-friendly model that combines low-precision data
paths with high-precision residuals. We introduce approximate computing
techniques to optimize SC nonlinear adders and provide some new SC designs for
arithmetic operations required by SOTA models. Overall, our approach allows for
further significant improvements in circuit efficiency, flexibility, and
compatibility through circuit design and model co-optimization. The results
demonstrate that the proposed end-to-end SC architecture achieves accurate and
efficient neural network acceleration while flexibly accommodating model
requirements, showcasing the potential of SC in neural network acceleration.
更多查看译文
关键词
Stochastic Approximation,Low Precision,Circuit Design,Different Types Of Support,Datapath,Efficient Acceleration,State-of-the-art Models,Activation Function,High Precision,Energy Efficiency,Batch Normalization,Computational Accuracy,Fault-tolerant,Input Information,Bit Error Rate,Accuracy Of Network,Residual Connection,ReLU Function
AI 理解论文
溯源树
样例
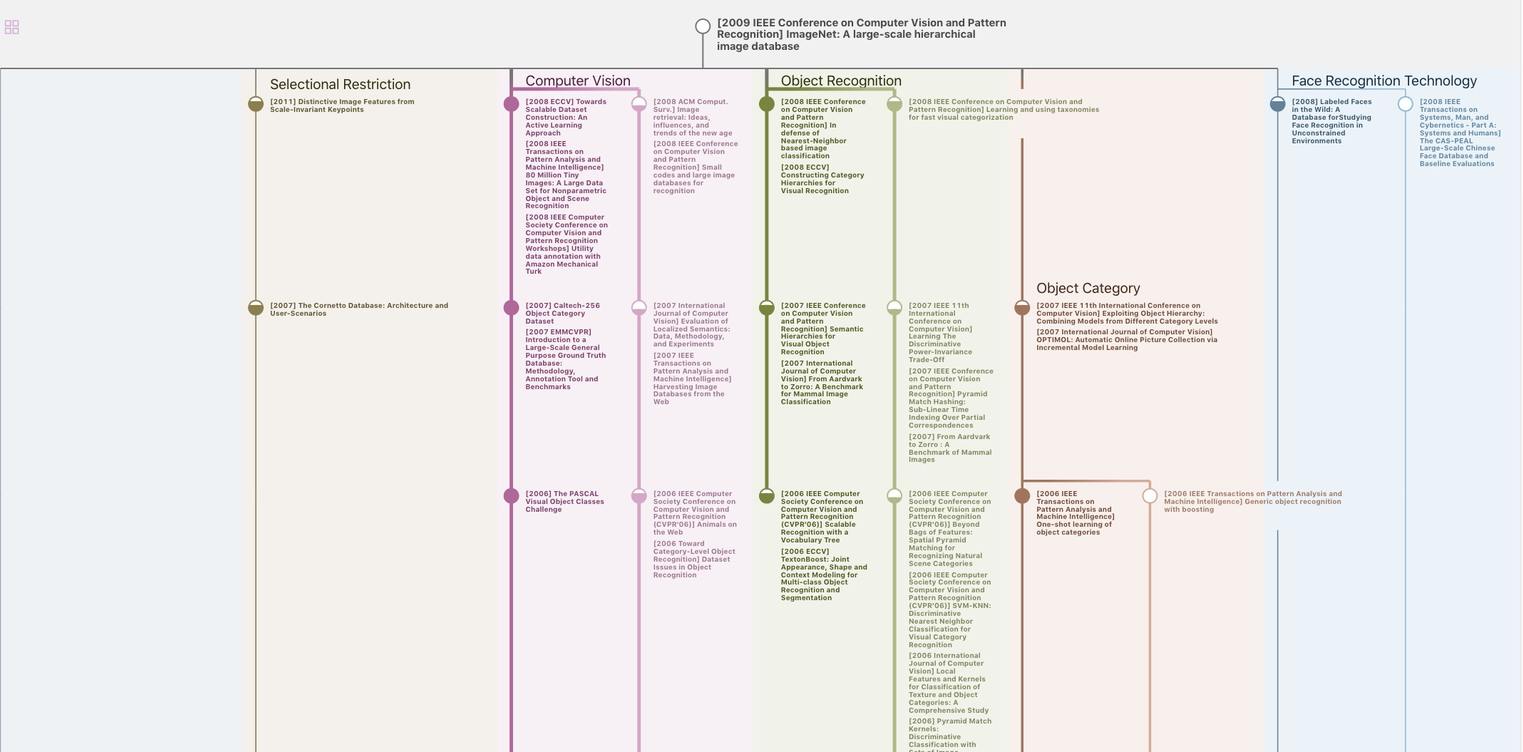
生成溯源树,研究论文发展脉络
Chat Paper
正在生成论文摘要