Performance Assessment of U-Net for Semantic Segmentation of Liquid Spray Images with Gaussian Blurring
2023 IEEE International Conference on Computing (ICOCO)(2023)
摘要
Semantic segmentation plays a vital role in various computer vision applications. One of the convolutional networks which have been scholarly accepted as the prominent solution for semantic segmentation is U-Net, known for its effectiveness in capturing spatial context with fewer training images. In real-world applications of analysing liquid spray images, motion blur and defocus blur distortions likely appear due to camera positioning. The robustness of U-Net segmenting the object in the presence of image distortions still needs to be explored in the context of liquid spray images. This study aims to analyse the visual impact of U-Net for semantic segmentation in liquid spray images. Gaussian blurring is applied with different levels of distortion to the test image to simulate the blurring effect caused by the camera defocusing and unstable motion-positioning. Surprisingly, the experimental results showed that U-Net could recognise many initially unrecognisable objects in the test image after applying Gaussian blurring. Following this finding, a hypothesis is proposed that inducing an appropriate blurring artefact into the test image will significantly maximise the contour boundaries visibility, allowing the U-N et to segmentise the recognisable contour efficiently. A closer inspection of the pixel histogram revealed a narrow range of high-frequency values. The reduction of pixel variation makes the object appear connected without sharp separation, improving the continuity of the contour boundary. This quantitative observation is harmonised with the above hypothesis. Code is available at https://github.com/lynerlwl/unet-gaussian-blurring
更多查看译文
关键词
computer vision,image processing,image statistics,convolutional network,semantic segmentation
AI 理解论文
溯源树
样例
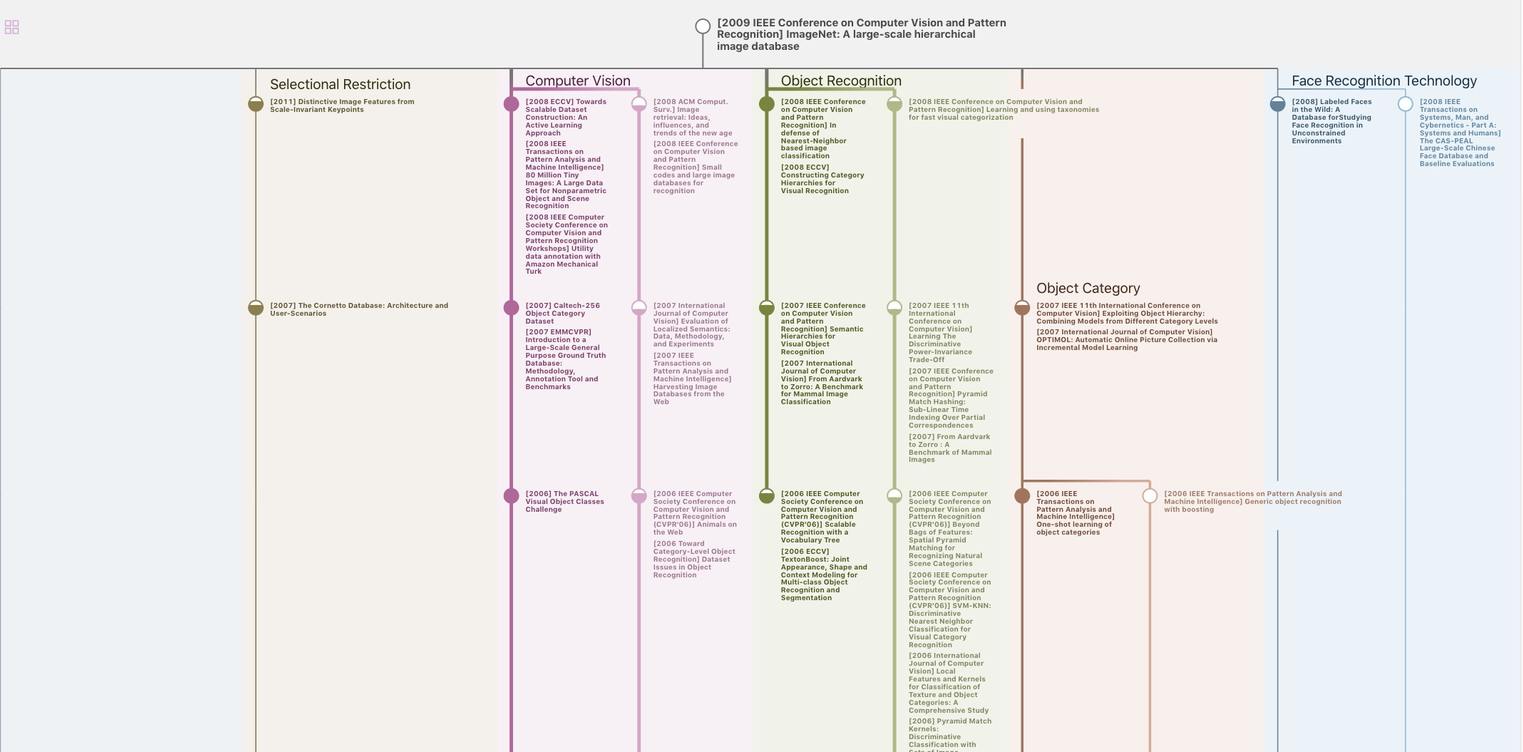
生成溯源树,研究论文发展脉络
Chat Paper
正在生成论文摘要