A novel method for predicting shallow hydrocarbon accumulation based on source-fault-sand (S-F-Sd) evaluation and ensemble neural network (ENN)
APPLIED ENERGY(2024)
摘要
Shallow hydrocarbon accumulation (SHA) and predrilling volume prediction are important components of offshore oil and gas exploration. However, SHA prediction is complex and involves geological and technical uncertainties. Despite advances in available technology, reliable and convenient methods for predicting SHA are urgently needed by oil companies to avoid costly drilling mistakes. This study proposes a novel method for SHA prediction by combining source-fault-sand (S-F-Sd) evaluation and ensemble neural network (ENN) algorithms. First, twelve main controlling factors affecting SHA, which predominantly included geological parameters related to source rocks (S), fault zones (F) and sandstone reservoirs (Sd), were screened and quantified. Second, the six principal components obtained after the dimensionality reduction of the main control factors were selected as the model inputs. Then, using the BP neural network (BPNN), bagged neural network ensemble (Bagged-NNE) and boosted neural network ensemble (Boosted-NNE) algorithms, three different SHA prediction models with hydrocarbon column height (HCH) as the output were constructed. These models were applied to the K gasfield in the Xihu Depression, East China Sea Basin, to evaluate and optimize the model performance. Finally, the variable importance and the possible uncertainties in SHA prediction were discussed. The results show that the Boosted-NNE model is superior to the Bagged-NNE and BPNN models in SHA prediction. Moreover, the geological reserves of sandstone reservoirs calculated using the predicted HCH are close to the existing evaluation, which proves the effectiveness of the model output. In terms of variable importance, the synthetic parameters F1, F2, F5 and F4 obtained after dimensionality reduction are the four top principal components contributing to the model output. Under single-factor control, the HCH is positively correlated with the hydrocarbon expulsion rate, shale gouge ratio, sandstone thickness, porosity and permeability, but the relationship between the HCH and other controlling factors tends to be complicated. In addition, the model accuracy is affected by the uncertainties arising from the quantification and screening of the main controlling factors, as well as the dataset size and the machine learning algorithm selection. This contribution provides a reliable method for SHA prediction and corresponding predrilling volume evaluation, which can help avoid costly drilling mistakes and advance intelligent exploration techniques.
更多查看译文
关键词
Shallow hydrocarbon accumulation,Quantitative prediction,Machine learning,Ensemble neural network,Source -fault -sand evaluation
AI 理解论文
溯源树
样例
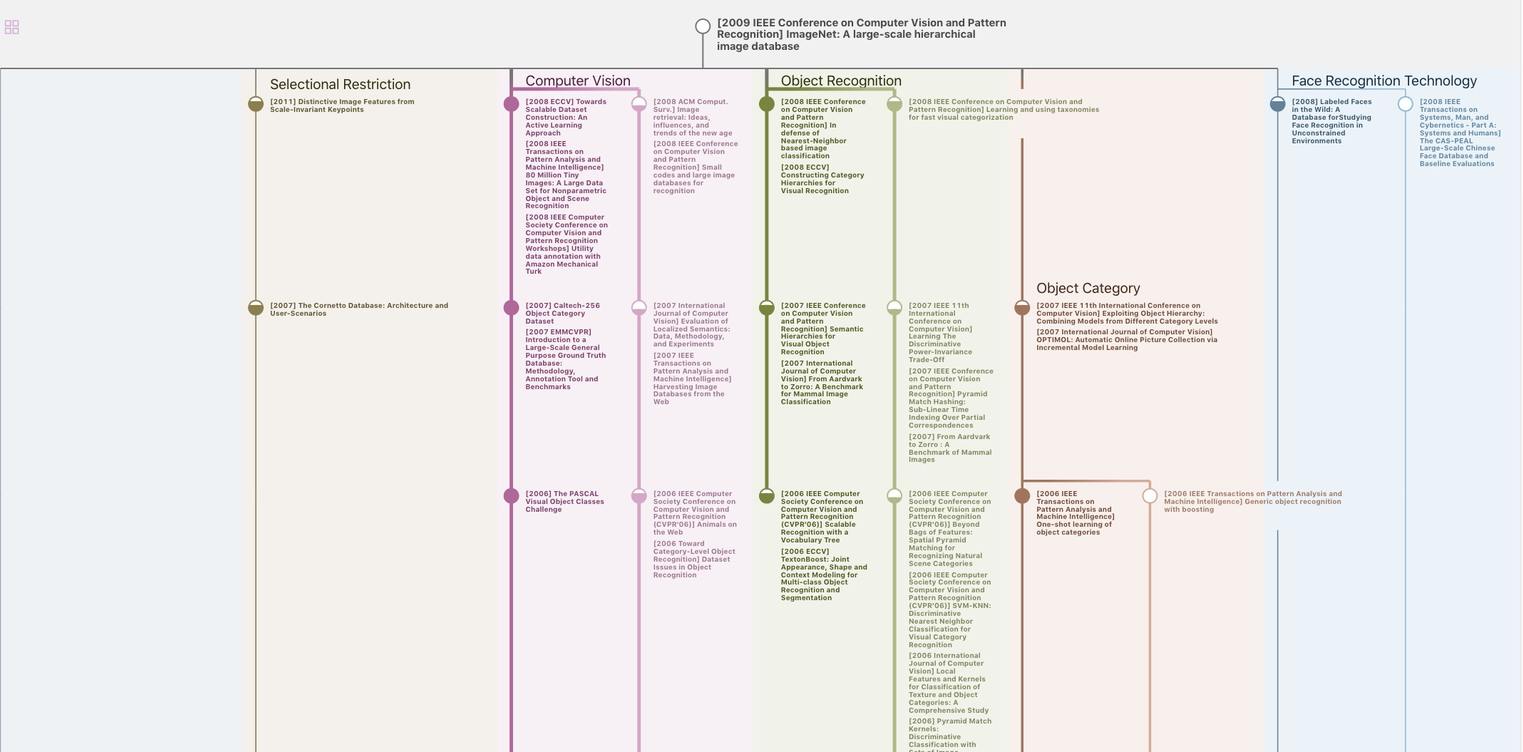
生成溯源树,研究论文发展脉络
Chat Paper
正在生成论文摘要