Differentiable Automatic Structural Optimization Using Graph Deep Learning
Advanced Engineering Informatics(2024)
摘要
The current limitations of non-differentiable evaluation models and computationally expensive heuristic algorithms hinder the optimization of real-world structures. This research proposes an efficient differentiable structural optimization framework using graph deep learning. We introduce a high-fidelity graph representation of structural systems and leverage graph neural networks to establish surrogate models for evaluating structural responses. Gradient-based algorithms are then utilized for optimization. Case studies on optimizing concrete frame structures demonstrate that our proposed framework achieves satisfactory outcomes within a remarkably short timeframe of 2-4 min, while reducing costs by at least 22% compared to manual design. Furthermore, when compared to traditional approaches that rely on heuristic algorithms and finite element methods, our differentiable framework exhibits a significant efficiency improvement of 25,000 times. The versatility of our framework extends beyond design problems, offering potential applications in reverse problems during the construction and maintenance stages, such as model updating and calibration.
更多查看译文
关键词
Differentiable structural optimization,Graph deep learning,Intelligent design,Building structures,Reverse problem
AI 理解论文
溯源树
样例
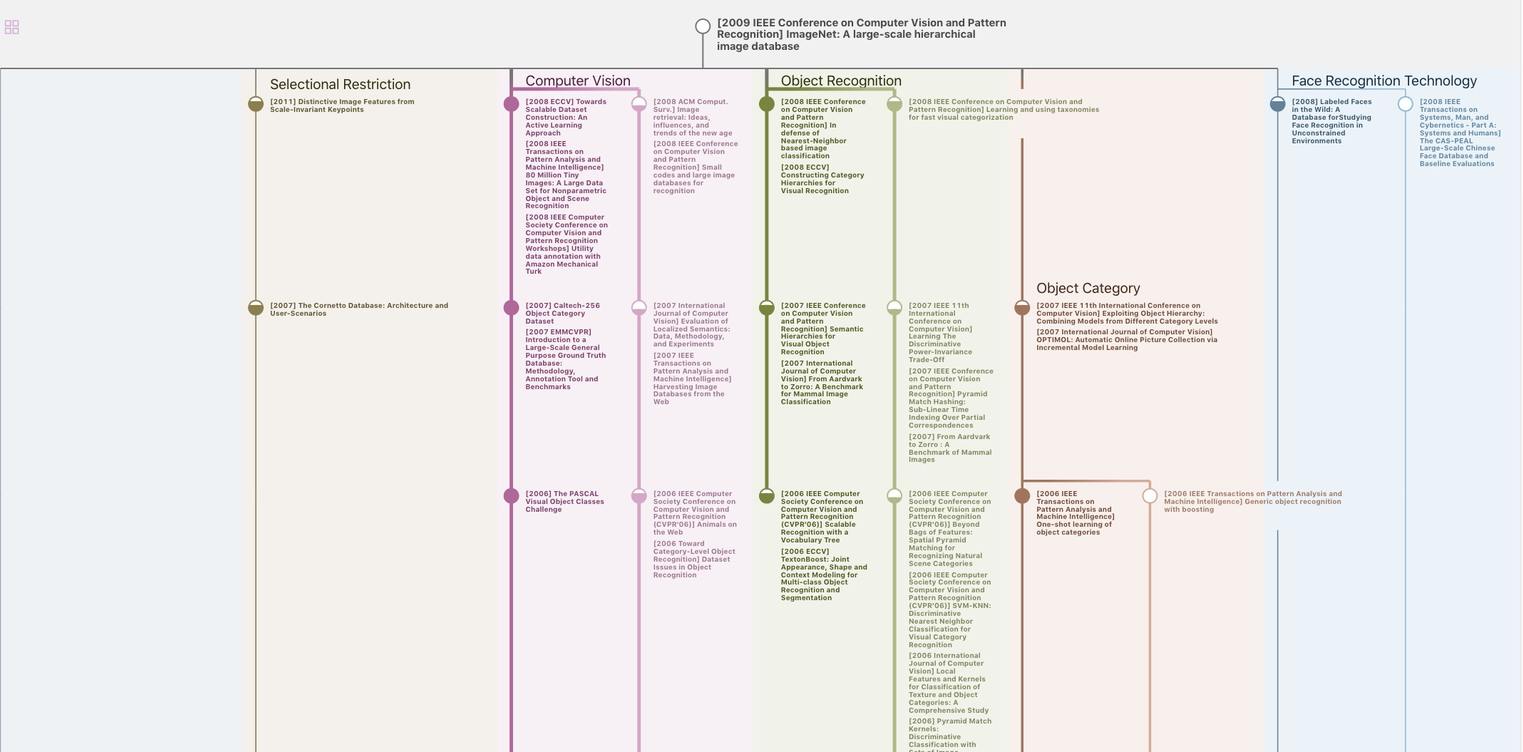
生成溯源树,研究论文发展脉络
Chat Paper
正在生成论文摘要