Subdomain adaptation joint attention network enabled two-stage strategy towards few-shot fault diagnosis of LRE turbopump
ADVANCED ENGINEERING INFORMATICS(2024)
摘要
Turbopump is the key component of liquid rocket engine (LRE), and its working status directly affects the performance of the launch system. However, it is usually difficult to obtain abundant training samples for turbopump, which has become a major factor limiting the application of deep learning based fault diagnosis technologies. In this paper, a two -stage strategy towards turbopump fault diagnosis under both limited labeled and unlabeled data based on variational auto -encoder (VAE) and subdomain adaptation joint attention network (SAJANet) is developed. In the suggested strategy, to better coordinate the importance of different layers during domain adaptation, the joint attention mechanism that can take the relationship between different layers into consideration is proposed and embedded into a subdomain adaptation network to developed the SAJANet. Subsequently, by combining the developed SAJANet with VAE, the "Generation -Transfer" two -stage diagnosis strategy for LRE turbopump under both limited labeled and unlabeled data is developed. In the suggested twostage strategy, the function of VAE is to preliminary expand the data volume utilizing extremely few labeled data, while the SAJANet followed by is utilized to perform transfer learning from the VAE manual samples to the actual samples. Through the above strategy, the SAJANet can be well pre -trained by the VAE data, and the introduction of the adaptation stage can further improve the model performance against actual data by fully utilizing the limited actual data. The superiority of the proposed method is verified by the data of an actual LRE turbopump.
更多查看译文
关键词
LRE turbopump,Fault diagnosis,Transfer learning,Attention mechanism,Data generation
AI 理解论文
溯源树
样例
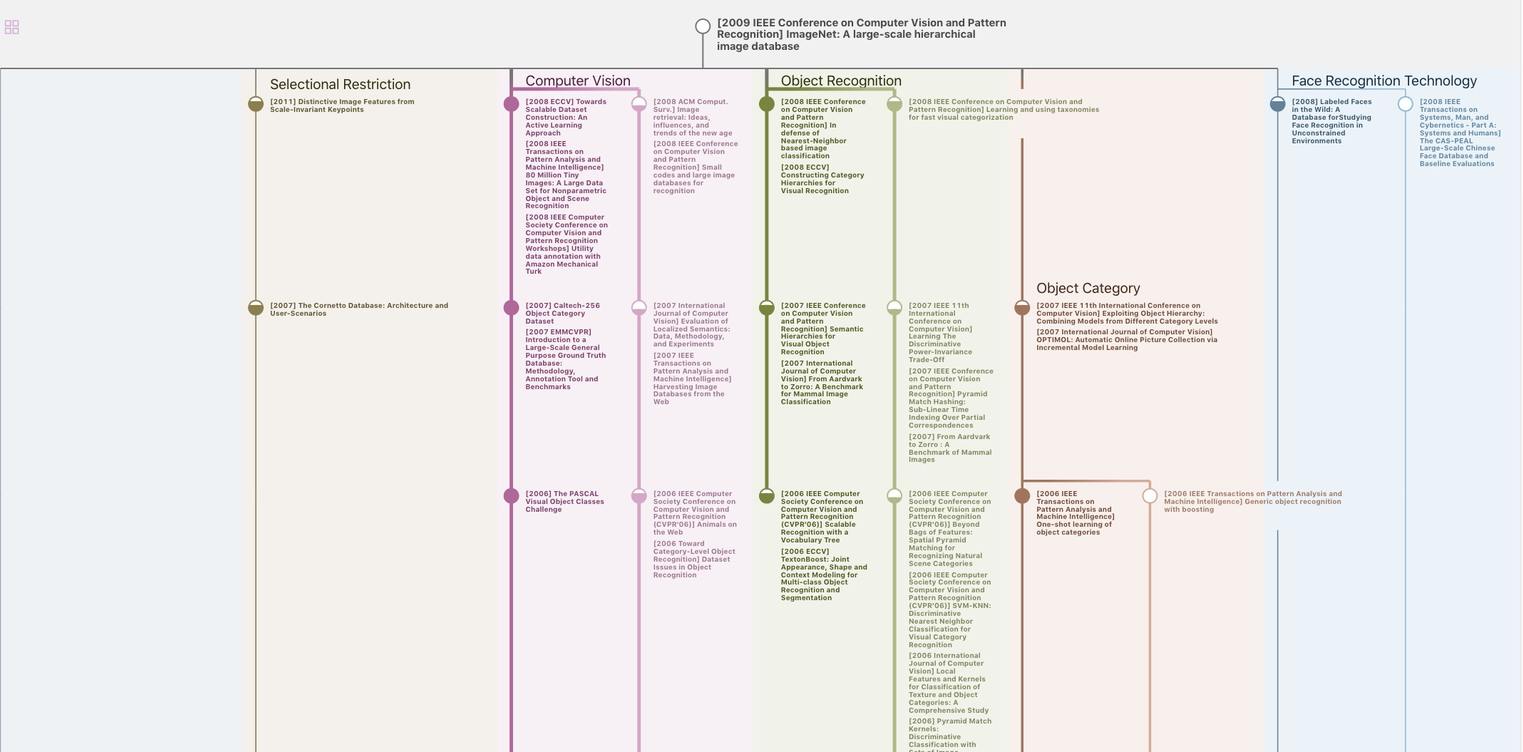
生成溯源树,研究论文发展脉络
Chat Paper
正在生成论文摘要