Deep learning in food authenticity: Recent advances and future trends
TRENDS IN FOOD SCIENCE & TECHNOLOGY(2024)
摘要
Background: The development of fast, efficient, accurate, and reliable techniques and methods for food authenticity identification is crucial for food quality assurance. Traditional machine learning algorithms often have limitations when handling complex sample data, exhibiting a suboptimal performance, particularly when addressing intricate problems and in large-scale data applications. In recent years, the emergence of deep learning algorithms has heralded revolutionary breakthroughs in the field of food authenticity identification, and the ongoing deep learning developments will continue to propel advancements in this field. Scope and approach: This review presents an overview of the deep learning algorithms and various categories of deep neural network models and structures, including the multilayer perceptron (MLP), convolutional neural network (CNN), recurrent neural network (RNN), autoencoder (AE), generative adversarial network (GAN), and attention mechanism (AM). It also summarizes the applications of these models, as well as the use of integrated models together with various analytical techniques in food authenticity. In addition, the latest developments and trends in deep learning in this field are discussed. Key findings and conclusions: The formidable capabilities of deep learning algorithms, in synergy with a broad array of analytical techniques, enhance the precision and efficiency of the analysis of the diverse food components. Concurrently, they have distinct advantages over traditional machine learning algorithms, showing significant potential for food authenticity identification. Although the use of deep learning still faces some challenges, with continuous technological advancements, more deep learning applications are expected to emerge in the food industry in the future to safeguard food authenticity.
更多查看译文
关键词
Deep learning,Food authenticity,Adulteration,Geographical traceability,Application
AI 理解论文
溯源树
样例
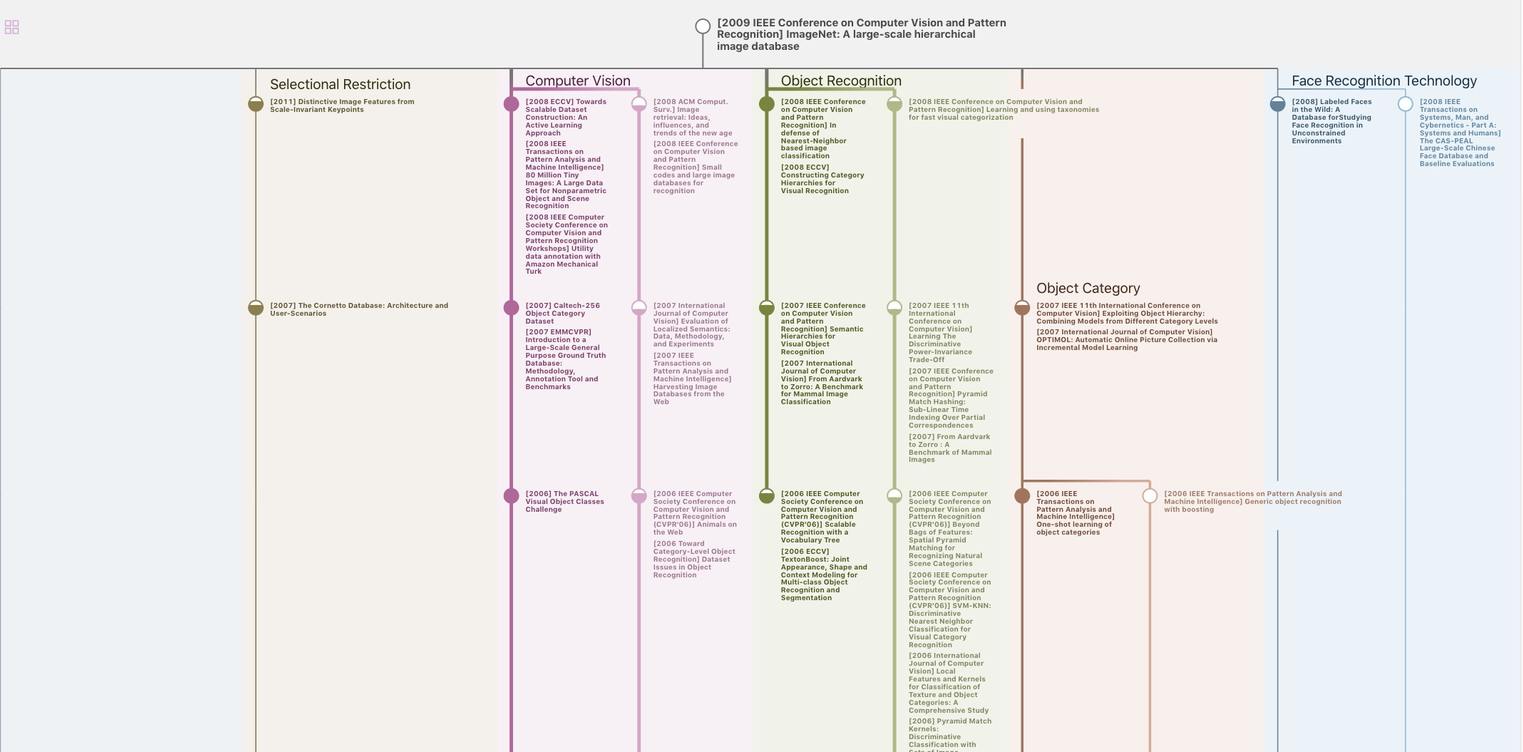
生成溯源树,研究论文发展脉络
Chat Paper
正在生成论文摘要