Rapid prediction of indoor airflow field using operator neural network with small dataset
BUILDING AND ENVIRONMENT(2024)
摘要
Indoor airflow is one of the most critical factors affecting room comfort. The accurate prediction of indoor airflow fields is essential for efficient environmental control. However, representative methods for calculating indoor airflow organization, such as computational fluid dynamics (CFD), are complex and time consuming. Therefore, this study proposes a machine learning approach that can rapidly reconstruct indoor airflow fields. This method employs an improved deep operator network (DeepONet) and a Fourier neural operator (FNO) neural network to learn a set of solutions to a problem resembling a flow field and then realizes an accuracypreserving prediction of an unseen domain. First, we decompose the computational domain into subdomains and then reconstruct the flow field for the subdomains. Subsequently, the subdomains are stitched into the original computational domain. Using data enhancement techniques along with a small dataset, this method achieves satisfactory training results. The results indicate that for a two-dimensional isothermal flow field problem, both the improved DeepONet and FNO models accurately reconstruct the flow field. The mean squared errors are 0.28% and 1.89% for the improved DeepONet and FNO models, respectively, with both requiring less than 0.01s. This method does not require repeated training under different operating conditions, which significantly reduces the computation time. Compared with conventional CFD technology, the proposed method improves the speed by up to three to six orders of magnitude.
更多查看译文
关键词
Deep learning,DeepONet,FNO,Operator neural network,Data -driven,Rapid prediction
AI 理解论文
溯源树
样例
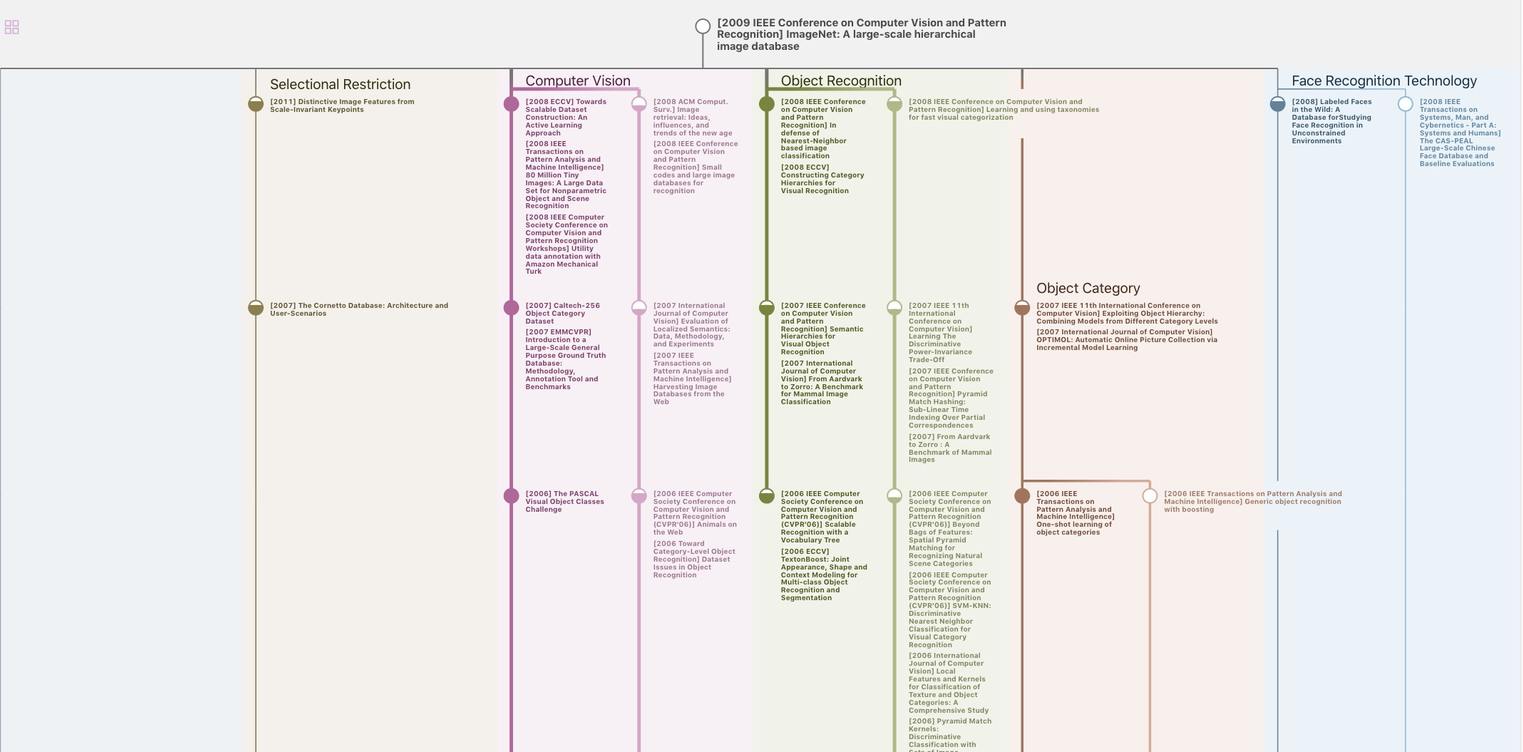
生成溯源树,研究论文发展脉络
Chat Paper
正在生成论文摘要