Application of near-infrared hyperspectral imaging for determination of cheese chemical composition
JOURNAL OF FOOD COMPOSITION AND ANALYSIS(2024)
摘要
Cheeses vary in chemical composition as they have different applications according to the technological features; some hard cheeses are consumed fresh while melting is a desirable characteristic for others. The conventional reference methods for determination of cheese composition and quality are accurate, but include physicalchemical analyses that are time-consuming and require use of chemical reagents. The objective of this work was to apply near-infrared hyperspectral imaging (NIR-HSI) as a fast method for prediction and visualization of spatial distribution of chemical composition of different types of cheeses (mozzarela, requeija similar to o do norte, minas meia cura, coalho, prato). Moisture, fat, protein, and free oil were measured, and used as reference for prediction models, while spectral information extracted from images in the range of 928-2524 nm were used as predictors. Partial Least Squares (PLS) regression models were built with full spectra and using only few selected regions with higher importance, which were applied to the images to build chemical maps and visualize the spatial variation of the cheese composition. The regression models were able to predict moisture (R2P = 0.92, RMSEP = 1.1%); fat (R2P = 0.84, RMSEP = 1.5%); protein (R2P = 0.80, RMSEP = 1.8%); and free oil (R2P =0.64, RMSEP = 0.5%) for an external set of samples, while requiring a small number of latent variables (LVs) (between 3 and 4). The chemical maps allowed visualization of the spatial distribution for each attribute, demonstrating that the composition may vary within sample. The study demonstrated the potential of NIR-HSI for the determination of cheese composition and possible application to cheese quality control.
更多查看译文
关键词
Chemical imaging,Partial least squares regression,Mozzarela,Coalho,Prediction,Dairy
AI 理解论文
溯源树
样例
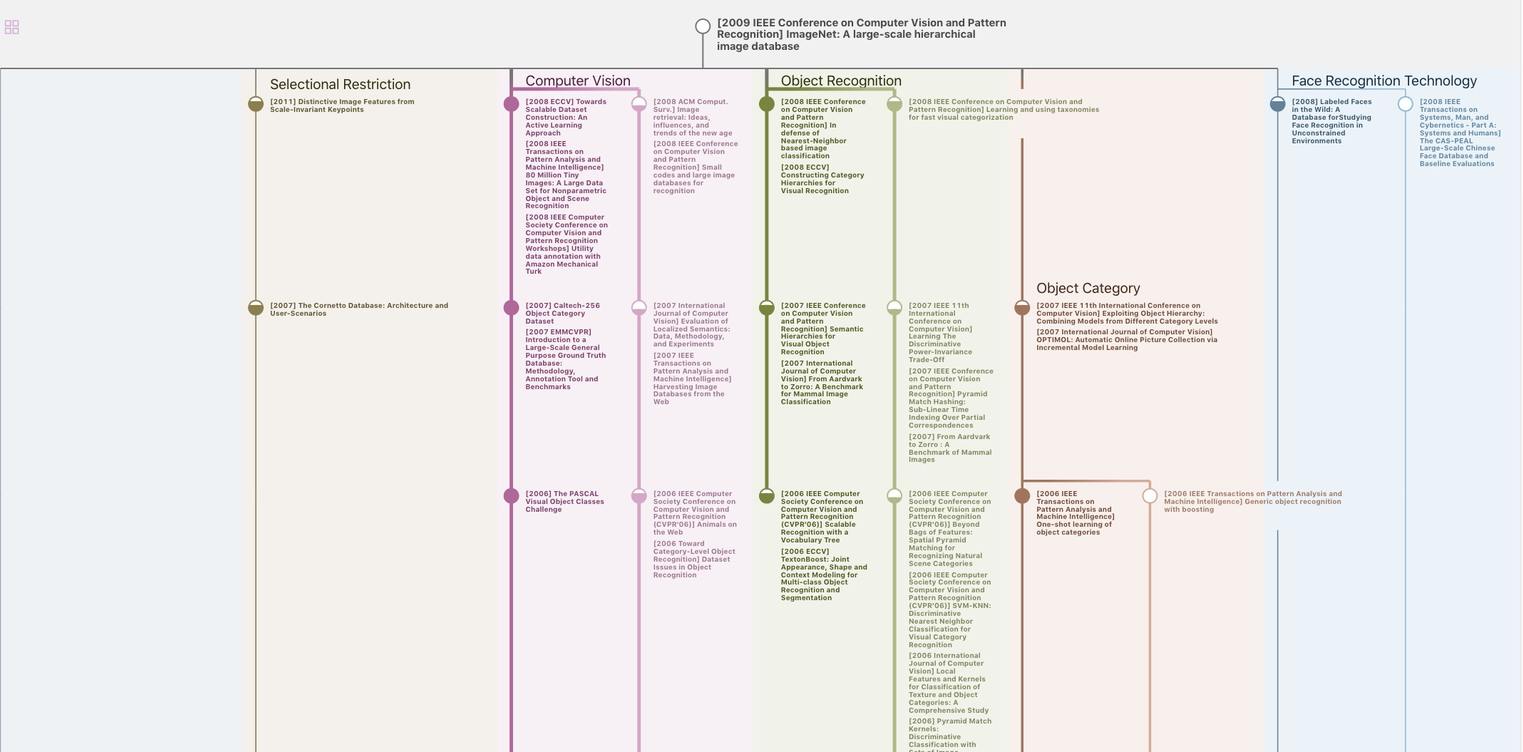
生成溯源树,研究论文发展脉络
Chat Paper
正在生成论文摘要