Synergizing Human Expertise and AI Efficiency with Language Model for Microscopy Operation and Automated Experiment Design
CoRR(2024)
摘要
With the advent of large language models (LLMs), in both the open source and
proprietary domains, attention is turning to how to exploit such artificial
intelligence (AI) systems in assisting complex scientific tasks, such as
material synthesis, characterization, analysis and discovery. Here, we explore
the utility of LLM, particularly ChatGPT4, in combination with application
program interfaces (APIs) in tasks of experimental design, programming
workflows, and data analysis in scanning probe microscopy, using both in-house
developed API and API given by a commercial vendor for instrument control. We
find that the LLM can be especially useful in converting ideations of
experimental workflows to executable code on microscope APIs. Beyond code
generation, we find that the GPT4 is capable of analyzing microscopy images in
a generic sense. At the same time, we find that GPT4 suffers from inability to
extend beyond basic analyses or more in-depth technical experimental design. We
argue that a LLM specifically fine-tuned for individual scientific domains can
potentially be a better language interface for converting scientific ideations
from human experts to executable workflows, such a synergy between human
expertise and LLM efficiency in experimentation can open new door for
accelerating scientific research, enabling effective experimental protocols
archive and sharing in scientific community.
更多查看译文
关键词
automated experiment,microscopy,language model,application program interface
AI 理解论文
溯源树
样例
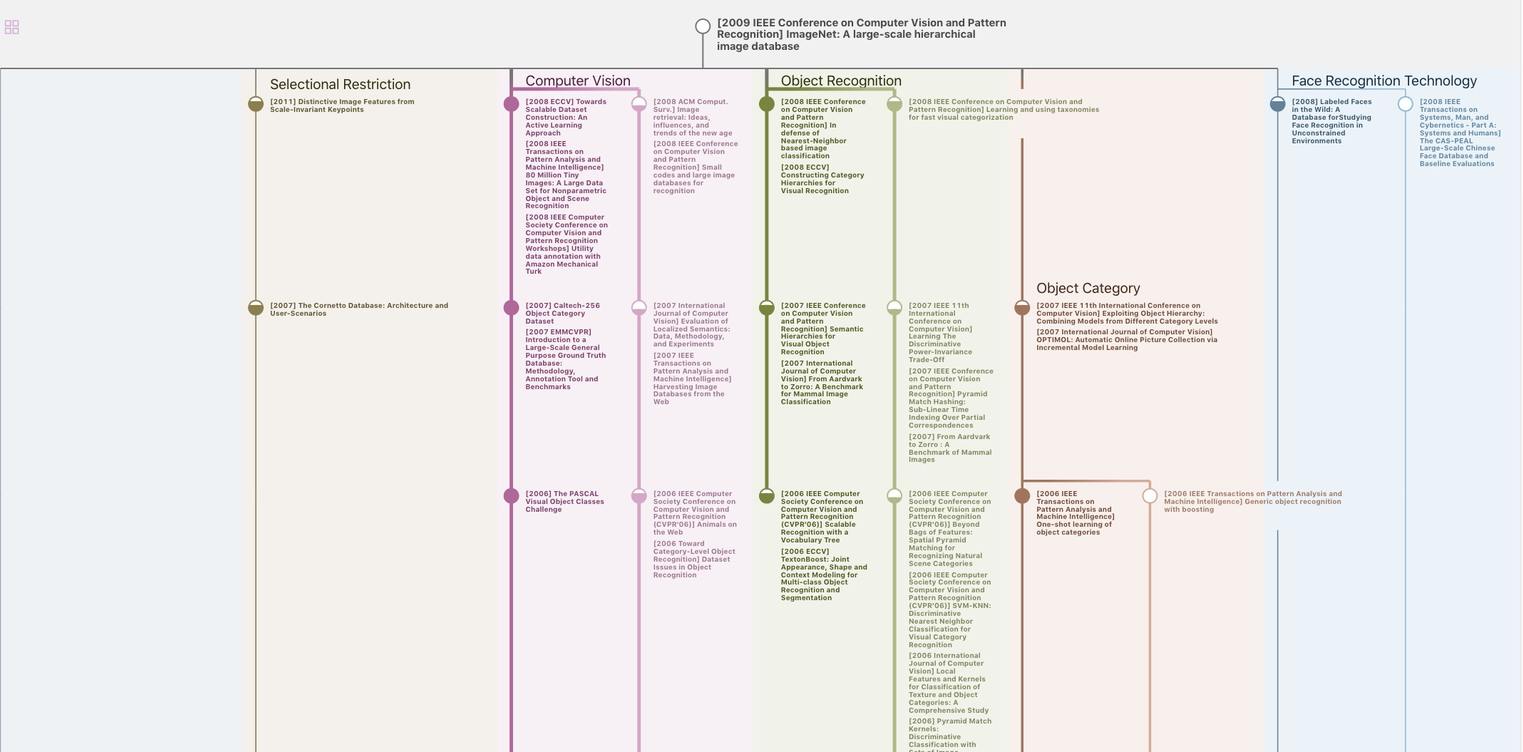
生成溯源树,研究论文发展脉络
Chat Paper
正在生成论文摘要