Sparse and Transferable Universal Singular Vectors Attack
CoRR(2024)
摘要
The research in the field of adversarial attacks and models' vulnerability is
one of the fundamental directions in modern machine learning. Recent studies
reveal the vulnerability phenomenon, and understanding the mechanisms behind
this is essential for improving neural network characteristics and
interpretability. In this paper, we propose a novel sparse universal white-box
adversarial attack. Our approach is based on truncated power iteration
providing sparsity to (p,q)-singular vectors of the hidden layers of Jacobian
matrices. Using the ImageNet benchmark validation subset, we analyze the
proposed method in various settings, achieving results comparable to dense
baselines with more than a 50
and utilizing 256 samples for perturbation fitting. We also show that our
algorithm admits higher attack magnitude without affecting the human ability to
solve the task. Furthermore, we investigate that the constructed perturbations
are highly transferable among different models without significantly decreasing
the fooling rate. Our findings demonstrate the vulnerability of
state-of-the-art models to sparse attacks and highlight the importance of
developing robust machine learning systems.
更多查看译文
AI 理解论文
溯源树
样例
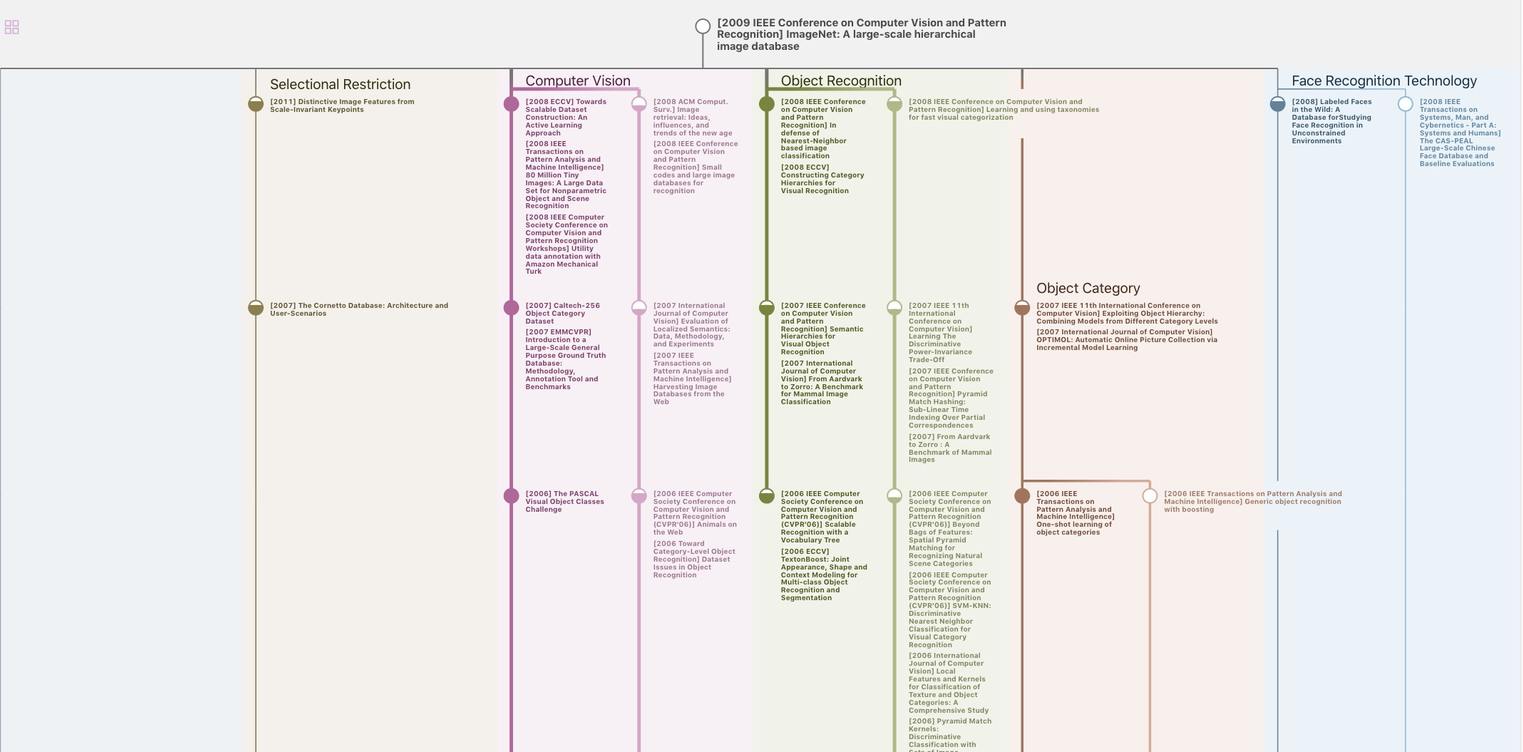
生成溯源树,研究论文发展脉络
Chat Paper
正在生成论文摘要