NUNI-Waste: novel semi-supervised semantic segmentation waste classification with non-uniform data augmentation
Multimedia Tools and Applications(2024)
摘要
Waste categorization and recycling are critical approaches for converting waste into valuable and functional materials, thereby significantly aiding in land preservation, reducing pollution, and optimizing resource usages. However, real-world classification and identification of recyclable waste face substantial hurdles due to the intricate and unpredictable nature of wastes, as well as the limited availability of comprehensive waste datasets. These factors limit efficacy of the existing research work in the domain of waste management. In this paper, we utilize semantic segmentation at individual pixel level and introduce a semi-supervised metod for authentic waste classification scenarios, leveraging the Zerowaste dataset. We devise a non-standard data augmentation strategy that mimics the ever-changing conditions of real-world waste environments. Additionally, we introduce an adaptive weighted loss function and dynamically adjust the ratio of positive to negative samples through a masking method, ensuring the model learns from relevant samples. Lastly, to maintain consistency between predictions made on data-augmented images and the original counterparts, we remove input perturbations. Our method proves to be effective, as verified by an array of standard experiments and ablation studies, achieved an accuracy improvement of 3.74% over the baseline Zerowaste method.
更多查看译文
关键词
Semi-supervised semantic segmentation,Data augmentation,Waste classification,Adaptive weighted loss function
AI 理解论文
溯源树
样例
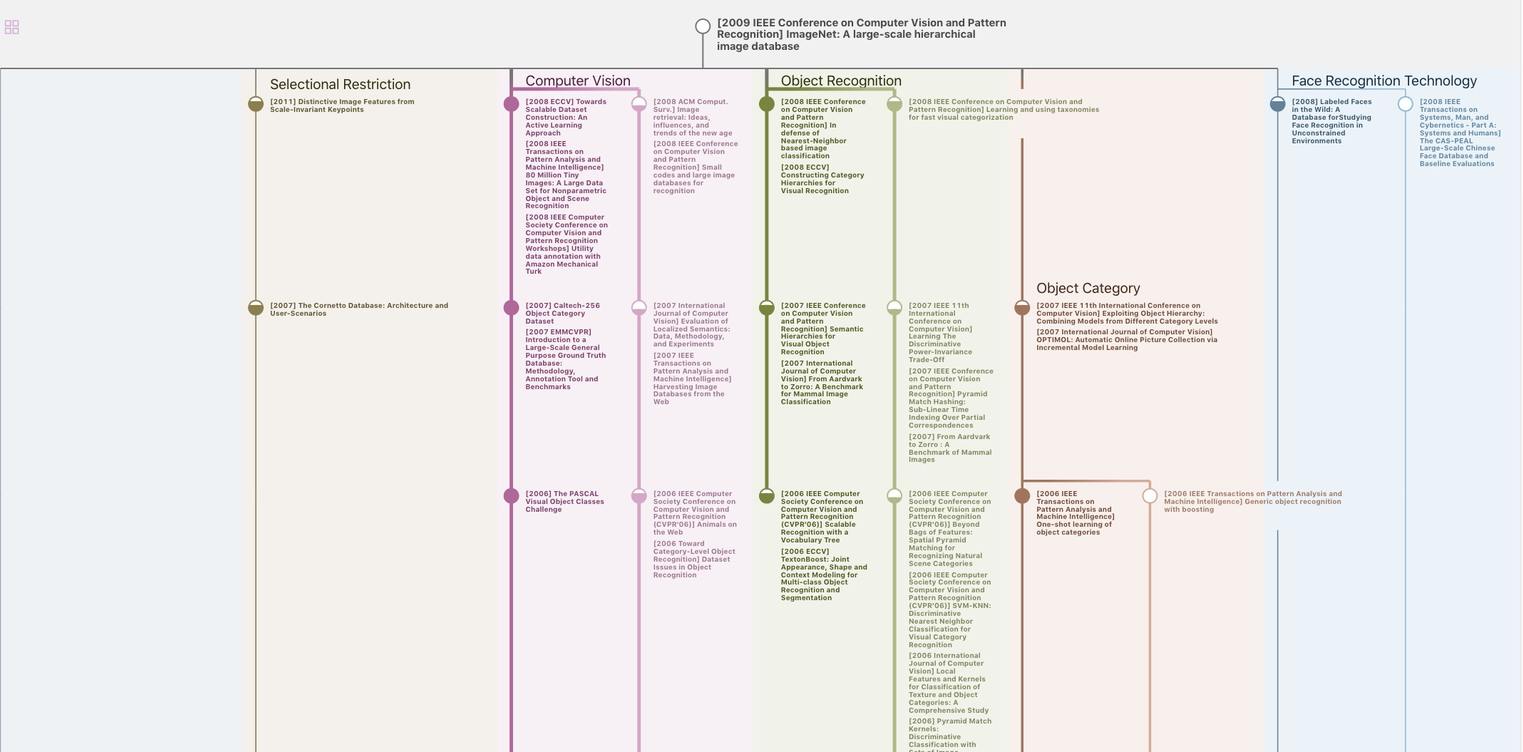
生成溯源树,研究论文发展脉络
Chat Paper
正在生成论文摘要