Metaheuristic learning algorithms for accurate prediction of hydraulic performance of porous embankment weirs
APPLIED SOFT COMPUTING(2024)
摘要
A porous weir is an environmentally friendly structure with minimal negative environmental impact. Due to the complex flow mechanism around porous weirs, it is difficult to provide a general empirical relationship to estimate the free and submerged discharge coefficients and threshold submergence or modular limit. Hence, the current study attempts to introduce an efficient alternative approach leveraging on multilayer perceptron (MLP) coupled with generalized normal distribution optimization (GNDO), Runge-Kutta optimization algorithm (RUN), slime mould algorithm (SMA), whale optimization algorithm (WOA), and grey wolf optimizer (GWO). These models' robustness and accuracy are investigated to solve the free discharge coefficient (FDC), modular limit index (MLI), and submerge discharge reduction factor (SDRF) of the porous embankment weirs (PEWs) modeling problem where 6514 datasets are studied experimentally for free, modular limit, and submerged flow conditions. The performance of developed metaheuristic-MLP models is compared with a gradient algorithm-based MLP, namely Levenberg-Marquardt (LM) model. The obtained results from analysis of different performance indicators demonstrate that the GNDO-MLP outperforms other developed MLP models. The results demonstrate that the metaheuristic-based MLP models are more reliable in forecasting FDC, MLI, and SDRF of PEWs than the ML-MLP model. To measure how much the MLP model improved when combined with meta-heuristic algorithms, an aggregated statistical index called objective function was applied. The results of this index showed that the MLP model with GNDO performed better than the MLP models with WOA, SMA, GWO, RUN, and LM by 82%, 66%, 69%, 69%, and 96% for FDC, by 41%, 13%, 9%, 8%, and 48% for MLI, and by 64%, 20%, 8%, 4%, and 69% for SDRF condition, respectively. Furthermore, the results of the uncertainty analysis, using the P-factor and R-factor, demonstrated that GNDO-MLP had lower uncertainty compared to other models. The robustness of the GNDO-MLP model indicates that it can be used for further research on the probabilistic analysis of the PEWs.
更多查看译文
关键词
Discharge coefficient,Porous embankment weir,Metaheuristic algorithms,Multilayer perceptron
AI 理解论文
溯源树
样例
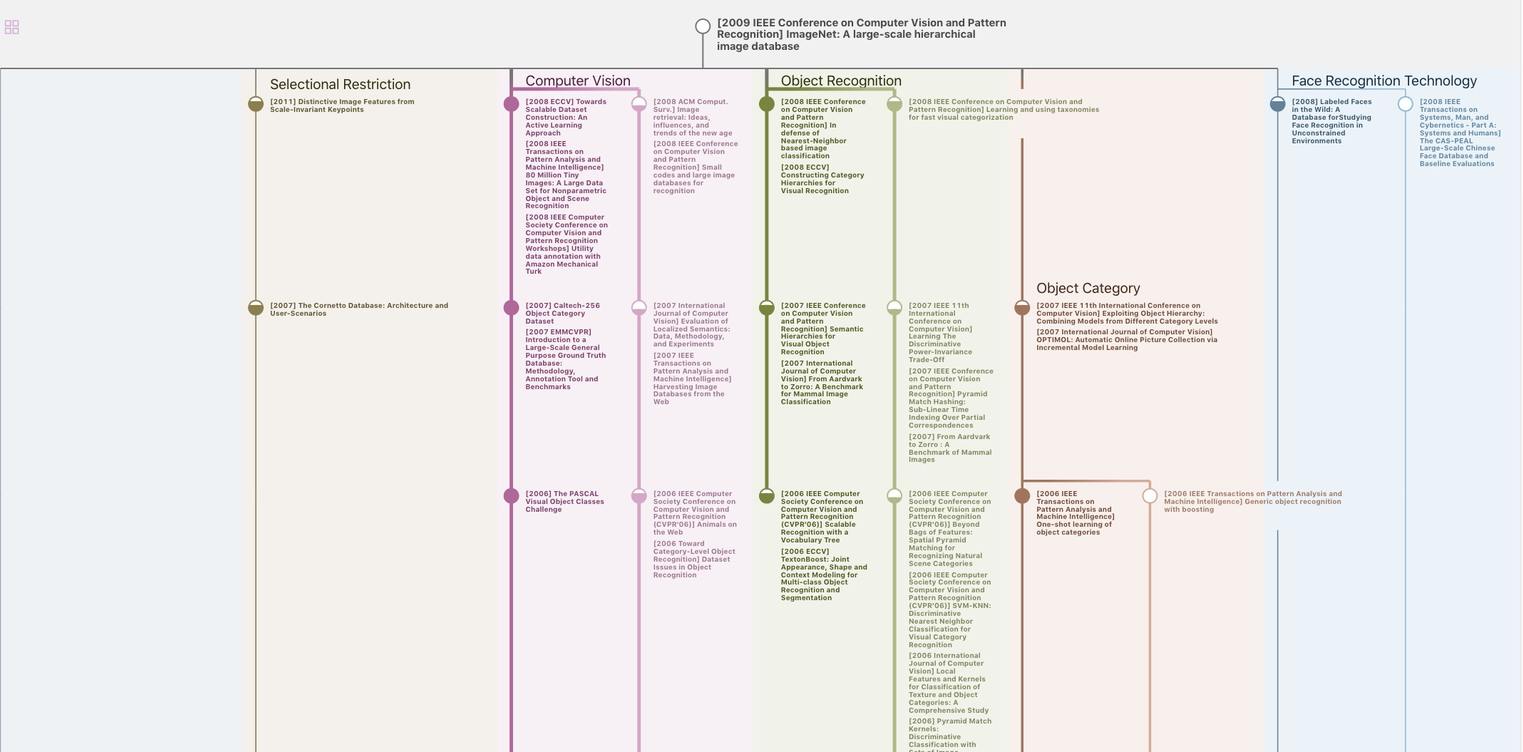
生成溯源树,研究论文发展脉络
Chat Paper
正在生成论文摘要