Towards deep understanding of graph convolutional networks for relation extraction
DATA & KNOWLEDGE ENGINEERING(2024)
摘要
Relation extraction aims at identifying semantic relations between pairs of named entities from unstructured texts and is considered an essential prerequisite for many downstream tasks in natural language processing (NLP). Owing to the ability in expressing complex relationships and interdependency, graph neural networks (GNNs) have been gradually used to solve the relation extraction problem and have achieved state-of-the-art results. However, the designs of GNN-based relation extraction methods are mostly based on empirical intuition, heuristic, and experimental trial-and-error. A clear understanding of why and how GNNs perform well in relation extraction tasks is lacking. In this study, we investigate three well-known GNN-based relation extraction models, CGCN, AGGCN, and SGCN, and aim to understand the underlying mechanisms of the extractions. In particular, we provide a visual analytic to reveal the dynamics of the models and provide insight into the function of intermediate convolutional layers. We determine that entities, particularly subjects and objects in them, are more important features than other words for relation extraction tasks. With various masking strategies, the significance of entity type to relation extraction is recognized. Then, from the perspective of the model architecture, we find that graph structure modeling and aggregation mechanisms in GCN do not significantly affect the performance improvement of GCN-based relation extraction models. The above findings are of great significance in promoting the development of GNNs. Based on these findings, an engineering oriented MLP-based GNN relation extraction model is proposed to achieve a comparable performance and greater efficiency.
更多查看译文
关键词
Graph neural networks,Relation extraction,Interpretability analysis,Visualizing and understanding
AI 理解论文
溯源树
样例
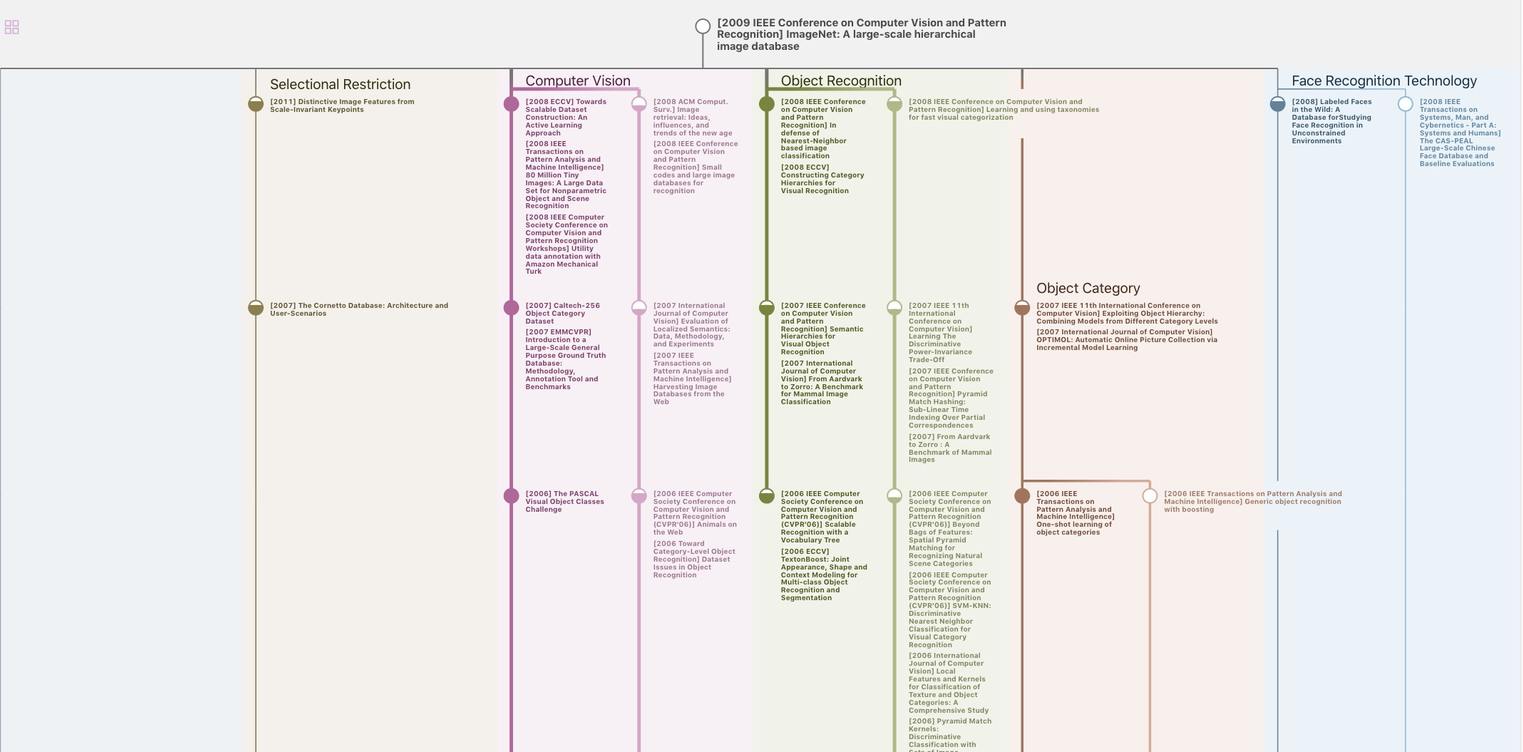
生成溯源树,研究论文发展脉络
Chat Paper
正在生成论文摘要