Neural network for predicting Peierls barrier spectrum and its influence on dislocation motion
Acta Materialia(2024)
摘要
The Peierls barrier represents the inherent lattice resistance to dislocation glide, controlling dislocation movement and dictating the resulting mechanical properties. The rise of multi-principal element alloys (MPEAs), with their vast compositional space and local chemical fluctuations, introduces notable challenges in precisely computing the Peierls barriers. Here, we propose a neural network model that captures the chemistry and structure of screw dislocations by incorporating two crucial inputs, the local atomic type and displacement, thus enabling accurate and efficient prediction of the Peierls barriers. Leveraging this neural network model, we construct a barrier map across the entire ternary space of refractory Nb-Mo-Ta alloys, from which the compositions with high or low barriers can be quickly identified. We discover that the NbMo binary alloy can have a higher barrier than NbMoTa ternary system, suggesting chemical complexity may not be the predominant factor governing the dislocation barrier. Subsequently, three screened compositions with different barriers are selected for studying their effects on dislocation motion. Our atomistic simulations indicate that a higher mean barrier slows down dislocation mobility, while a broader distribution of barriers facilitates kink pair nucleation, altering the rate-limiting process from kink pair nucleation to kink glide and cross kink. This study presents a general neural network model that enables rapid screening composition with the desired barrier spectrum and will impact the exploration and discovery of alloys with improved dislocation-controlled properties.
更多查看译文
关键词
Dislocation,Peierls barrier,Neural network,Refractory Alloys,High Entropy Alloys
AI 理解论文
溯源树
样例
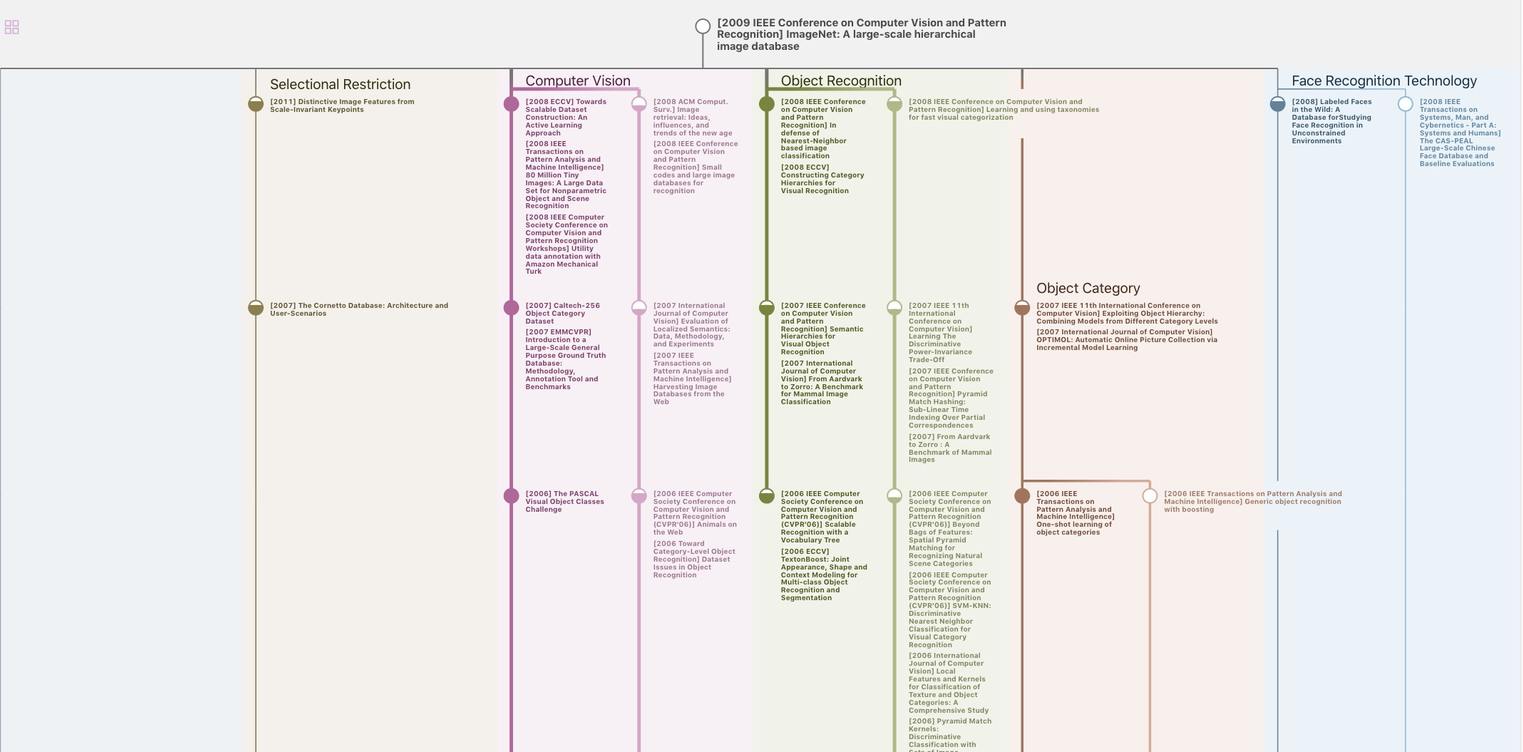
生成溯源树,研究论文发展脉络
Chat Paper
正在生成论文摘要