Intelligent fault diagnosis methods toward gas turbine: A review
CHINESE JOURNAL OF AERONAUTICS(2024)
摘要
Fault diagnosis plays a significant role in conducting condition-based maintenance and health management for gas turbines (GTs) to improve reliability and reduce costs. Various diagnosis methods developed by modeling engine systems or certain components implement faults detection and diagnosis based on the measurement of systemic parameters deviations. However, these conventional model-based methods are hindered by limitations of inability to handle the nonlinear nature, measurement uncertainty, fault coupling and other implementing problems. Recently, the development of artificial intelligence algorithms has provided an effective solution to the above problems, triggering broad researches for data-driven fault diagnosis methods with better accuracy, dynamic performance, and universality. This paper presents a systematic review of recently proposed intelligent fault diagnosis methods for GT engines, according to the classification of shallow learning methods, deep learning methods and hybrid intelligent methods. Moreover, the principle of typical algorithms, the evolution of enhanced methods, and the assessment of pros and cons are summarized to conclude the present status and look forward to the future in the field of GT fault diagnosis. Possible directions for development in method validation, information fusion, and interpretability of intelligent diagnosis methods are concluded in the end to provide insightful concepts for scholars in related fields. (c) 2023 Production and hosting by Elsevier Ltd. on behalf of Chinese Society of Aeronautics and Astronautics.
更多查看译文
关键词
Fault diagnosis,Health management,Gas turbine,Artificial intelligence,Intelligent diagnosis method
AI 理解论文
溯源树
样例
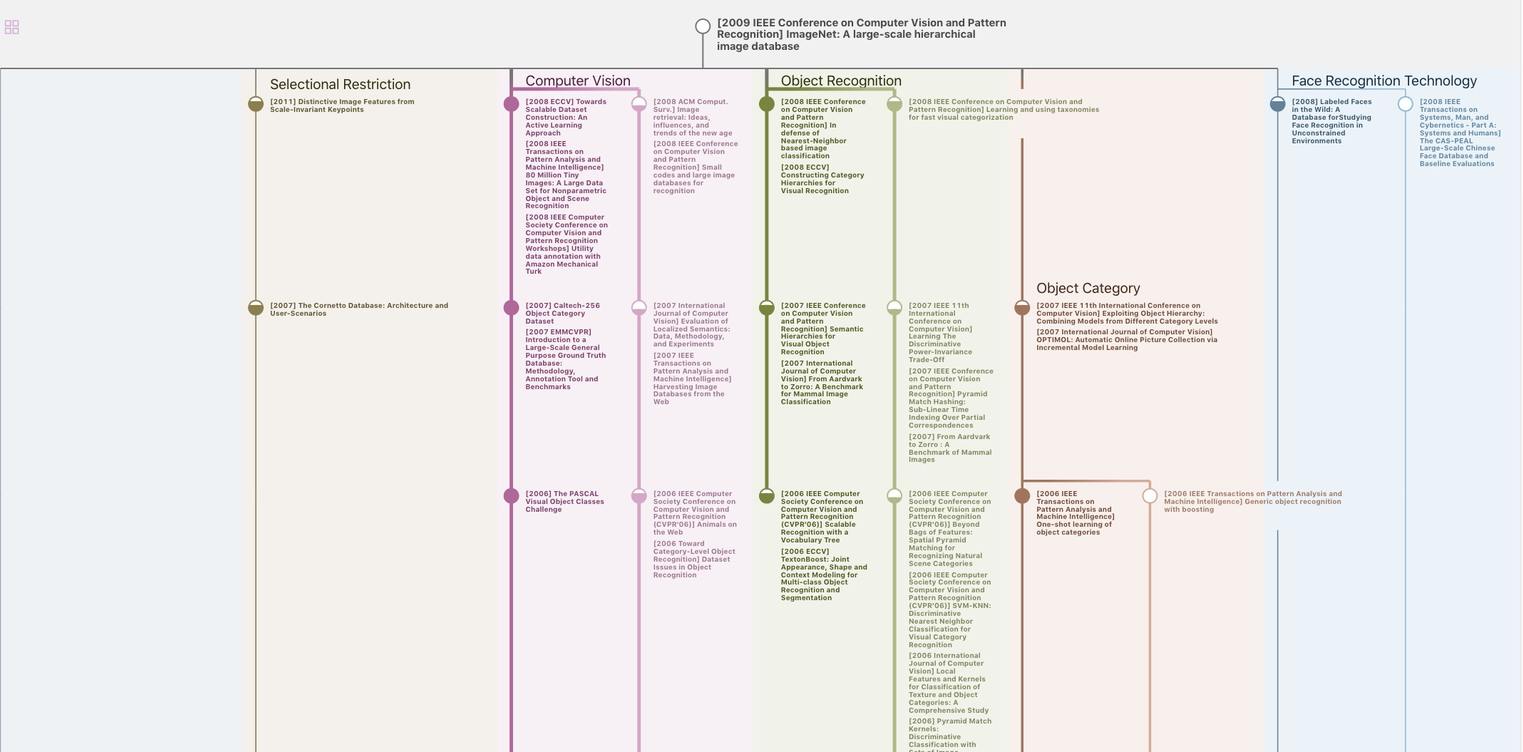
生成溯源树,研究论文发展脉络
Chat Paper
正在生成论文摘要