A dual-population multiobjective co-evolutionary matching ensemble learning for product multi-indicator prediction in continuous annealing
NEUROCOMPUTING(2024)
摘要
Achieving simultaneous and precise prediction of multiple product performance indicators is crucial to ensure stable production in the continuous annealing process. However, the majority of existing quality prediction modeling methods concentrate on developing single-output regression models that are limited to singleindicator prediction scenarios. When dealing with the multi-indicator prediction scenarios, these methods usually require the construction of a separate model for each performance indicator, which leads to more computational and storage resources and more complex maintenance in practical applications. To address this issue, a dual-population multiobjective co-evolutionary matching ensemble learning method called DP-CEMEL is developed. It utilizes dual-population co-optimization to enable the joint learning of the algorithm on input- output relationships and inter-indicator correlations. More specifically, a two-stage learning based individual encoding and decoding method and a performance boosting degree (PBD) based individual evaluation strategy are designed to achieve co-optimization of the dual populations to evolve a set of individuals (i.e., base learners) with good accuracy and low complexity. Then, a PBD based matching ensemble method is applied to get the final multi-indicator prediction model by the optimal matching and ensemble of the evolved individuals. Experimental results on both benchmark data and practical strip production data demonstrate that the proposed DP-CEMEL attains competitive or better performance compared to other state-of-the-art learning methods.
更多查看译文
关键词
Iron and steel industry,Dual-population co-evolutionary,Multiobjective optimization,Ensemble learning,Multi-indicator prediction
AI 理解论文
溯源树
样例
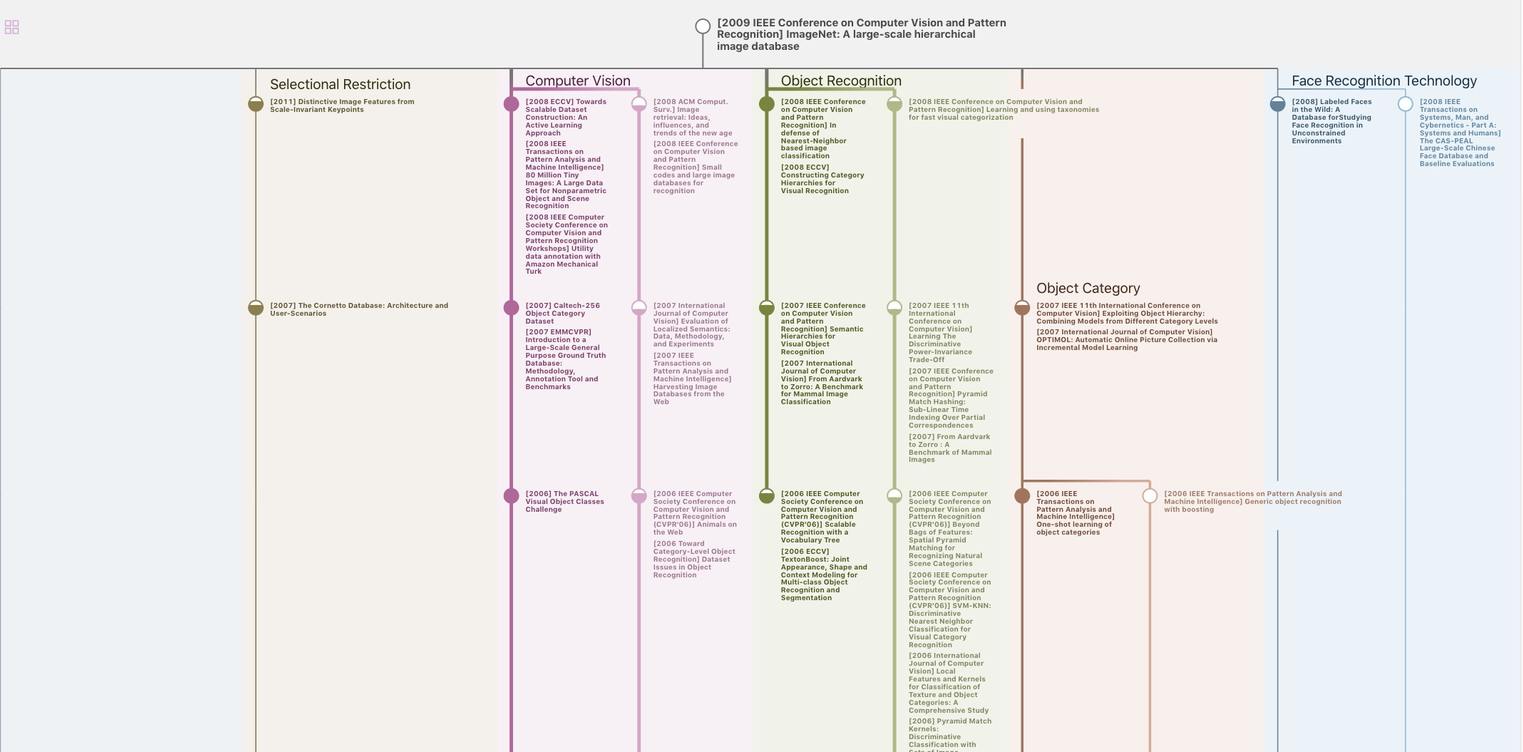
生成溯源树,研究论文发展脉络
Chat Paper
正在生成论文摘要