Outlier item detection in bundle recommendation via the attention mechanism
High-Confidence Computing(2024)
摘要
Bundle recommendation offers users more holistic insights by recommending multiple compatible items at once. However, the intricate correlations between items, varied user preferences, and the pronounced data sparsity in combinations present significant challenges for bundle recommendation algorithms. Furthermore, current bundle recommendation methods fail to identify mismatched items within a given set, a process termed as “outlier item detection”. These outlier items are those with the weakest correlations within a bundle. Identifying them can aid users in refining their item combinations. While the correlation among items can predict the detection of such outliers, the adaptability of combinations might not be adequately responsive to shifts in individual items during the learning phase. This limitation can hinder the algorithm’s performance. To tackle these challenges, we introduce an encoder–decoder architecture tailored for outlier item detection. The encoder learns potential item correlations through a self-attention mechanism. Concurrently, the decoder garners efficient inference frameworks by directly assessing item anomalies. We have validated the efficacy and efficiency of our proposed algorithm using real-world datasets.
更多查看译文
关键词
Bundle recommendation,Outlier item detection,Attention mechanism,Decoder encoder
AI 理解论文
溯源树
样例
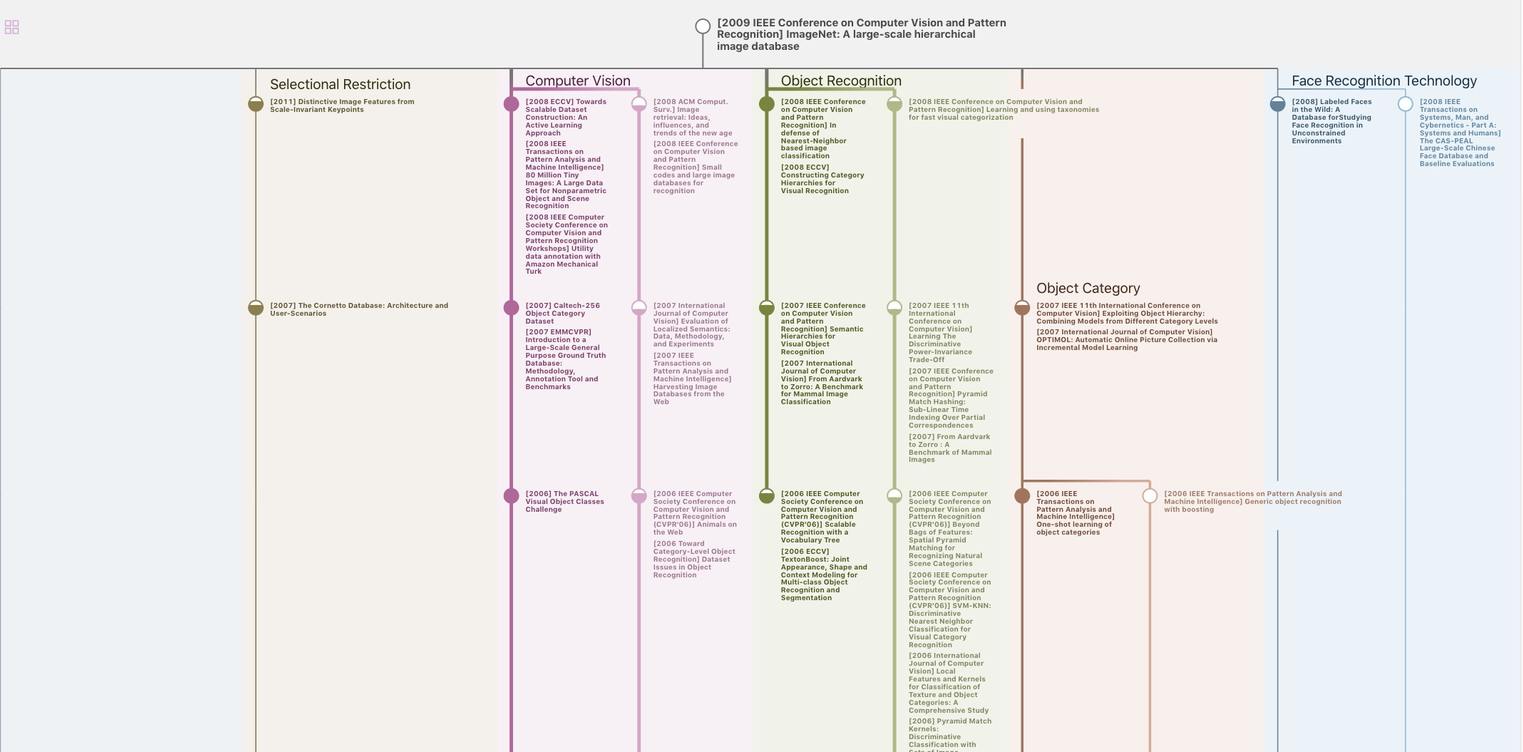
生成溯源树,研究论文发展脉络
Chat Paper
正在生成论文摘要