Joint condition monitoring framework of wind turbines based on multi-task learning with poor-quality data
ISA Transactions(2024)
摘要
Effective condition monitoring can improve the reliability of the turbine and reduce its downtime. However, due to the complexity of the operating conditions, the monitoring data is always mixed with poor-quality data. Poor-quality data mixed in monitoring tasks disrupts long-term dependency on data, which challenges traditional condition monitoring methods to work. To solve it, a joint reparameterization feature pyramid network (JRFPN) is proposed. Firstly, three different reparameterization tricks are designed to reform temporal information and exchange cross-temporal information, to alleviate the damage of long-term dependency. Secondly, a joint condition monitoring framework is designed, aiming to suppress feature confounding between poor-quality data and faulty data. The auxiliary task is trained to extract the degradation trend. The main task fights against feature confounding and dynamically delineates the failure threshold. The degradation trend and failure threshold decisions are corrected for each other to make the final joint state inference. Besides, considering the different quality of the monitoring variables, a channel weighting mechanism is designed to strengthen the ability of JRFPN. The measured data proved that JRFPN is more effective than other methods.
更多查看译文
关键词
Wind turbine,Condition Monitoring,Multi-task learning (MTL),Dilated causal Convolution (DCConv),Feature pyramid
AI 理解论文
溯源树
样例
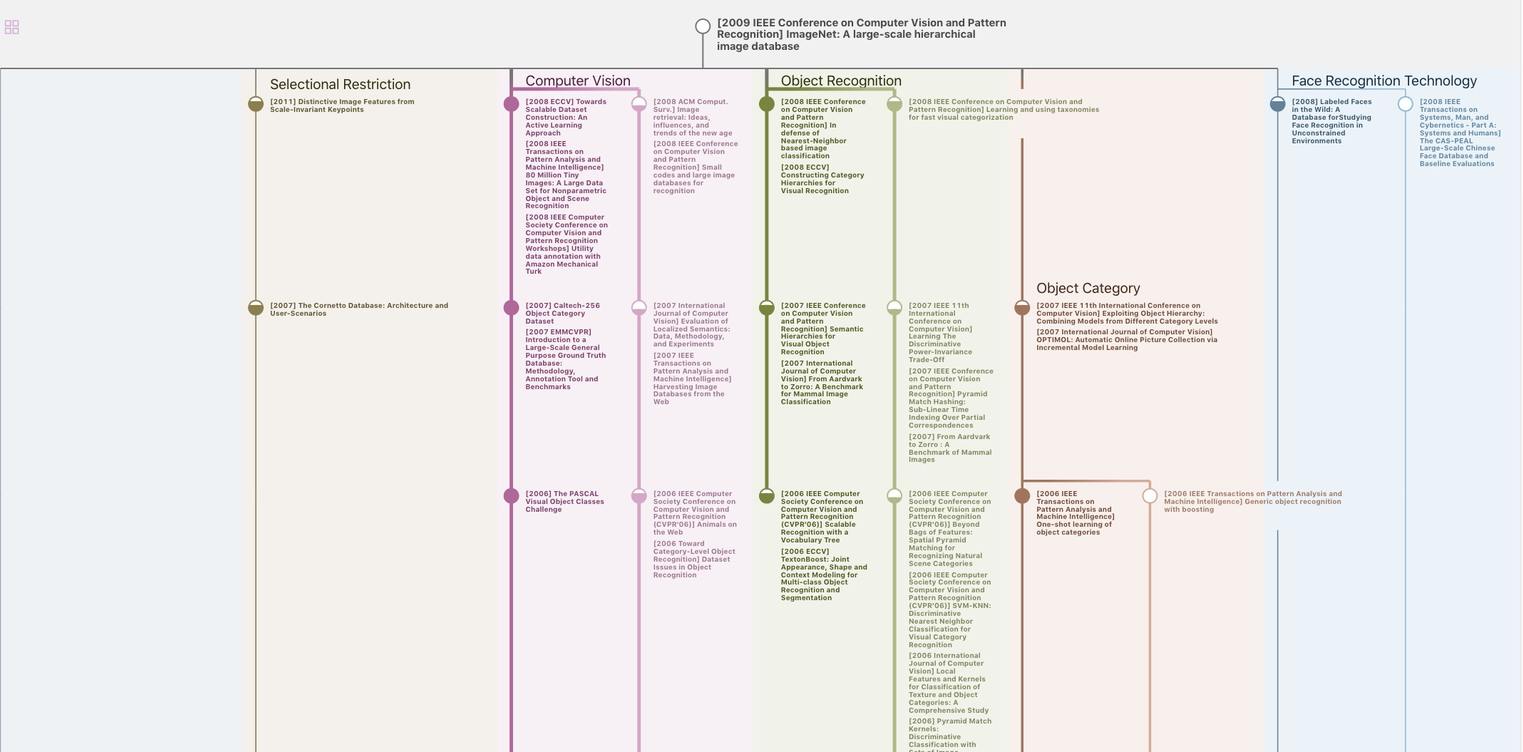
生成溯源树,研究论文发展脉络
Chat Paper
正在生成论文摘要